Rao-Blackwellizing the Straight-Through Gumbel-Softmax Gradient Estimator
ICLR(2021)
摘要
Gradient estimation in models with discrete latent variables is a challenging problem, because the simplest unbiased estimators tend to have high variance. To counteract this, modern estimators either introduce bias, rely on multiple function evaluations, or use learned, input-dependent baselines. Thus, there is a need for estimators that require minimal tuning, are computationally cheap, and have low mean squared error. In this paper, we show that the variance of the straight-through variant of the popular Gumbel-Softmax estimator can be reduced through Rao-Blackwellization without increasing the number of function evaluations. This provably reduces the mean squared error. We empirically demonstrate that this leads to variance reduction, faster convergence, and generally improved performance in two unsupervised latent variable models.
更多查看译文
关键词
gradient
AI 理解论文
溯源树
样例
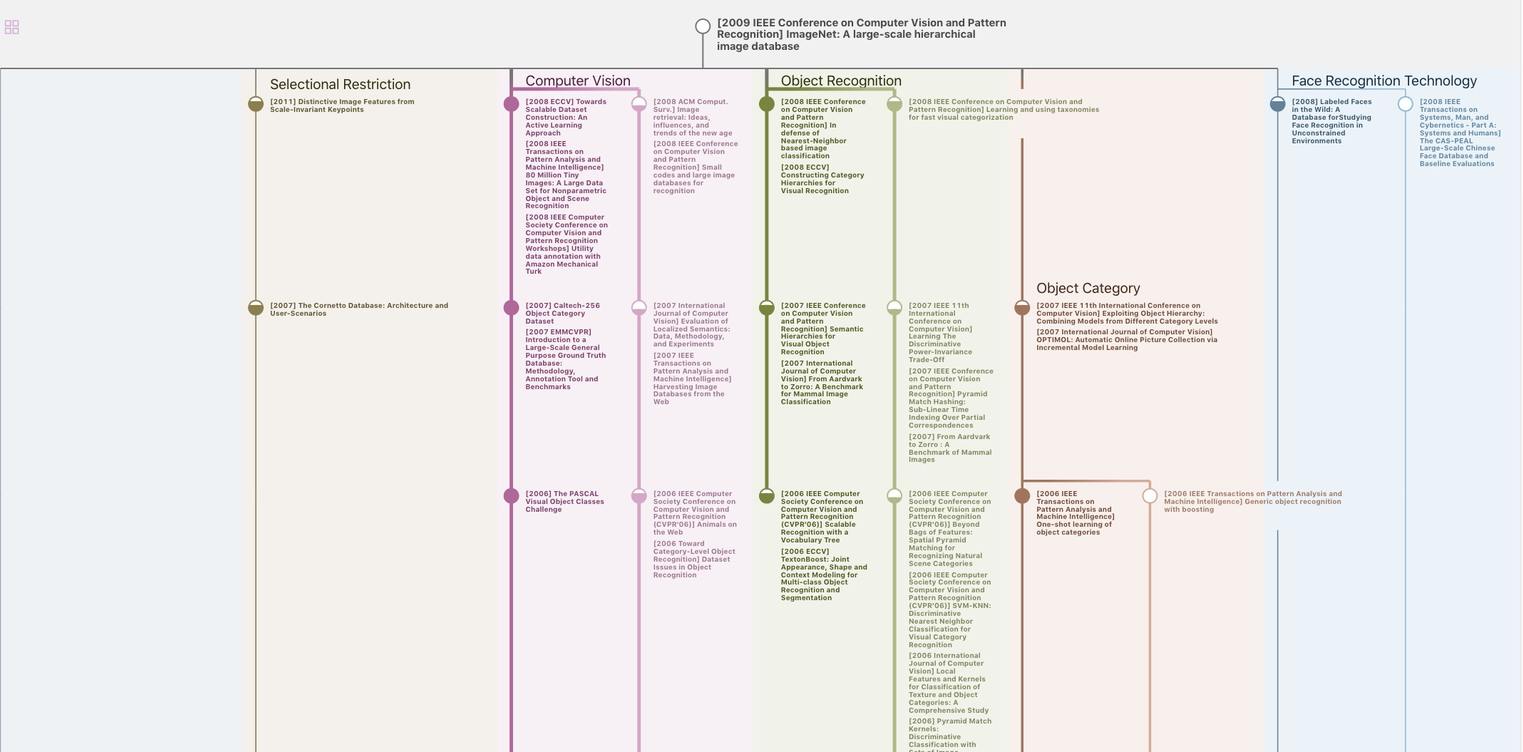
生成溯源树,研究论文发展脉络
Chat Paper
正在生成论文摘要