CurbScan: Curb Detection and Tracking Using Multi-Sensor Fusion
ITSC(2020)
摘要
Reliable curb detection is critical for safe autonomous driving in urban contexts. Curb detection and tracking are also useful in vehicle localization and path planning. Past work utilized a 3D LiDAR sensor to determine accurate distance information and the geometric attributes of curbs. However, such an approach requires dense point cloud data and is also vulnerable to false positives from obstacles present on both road and off-road areas. In this paper, we propose an approach to detect and track curbs by fusing together data from multiple sensors: sparse LiDAR data, a mono camera and low-cost ultrasonic sensors. The detection algorithm is based on a single 3D LiDAR and a mono camera sensor used to detect candidate curb features and it effectively removes false positives arising from surrounding static and moving obstacles. The detection accuracy of the tracking algorithm is boosted by using Kalman filter-based prediction and fusion with lateral distance information from low-cost ultrasonic sensors. We next propose a line-fitting algorithm that yields robust results for curb locations. Finally, we demonstrate the practical feasibility of our solution by testing in different road environments and evaluating our implementation in a real vehicle
1
. Our algorithm maintains over 90% accuracy within 4.5-22 meters and 0-14 meters for the KITTI dataset and our dataset respectively, and its average processing time per frame is approximately 10 ms on Intel i7 x86 and 100ms on NVIDIA Xavier board.
更多查看译文
关键词
NVIDIA Xavier board,Kalman filter-based prediction,distance information,autonomous driving,curb tracking,curb detection,CurbScan,sparse LiDAR data,off-road areas,geometric attributes,3D LiDAR sensor,path planning,vehicle localization,multisensor fusion,low-cost ultrasonic sensors,tracking algorithm,false positives removal,mono camera sensor
AI 理解论文
溯源树
样例
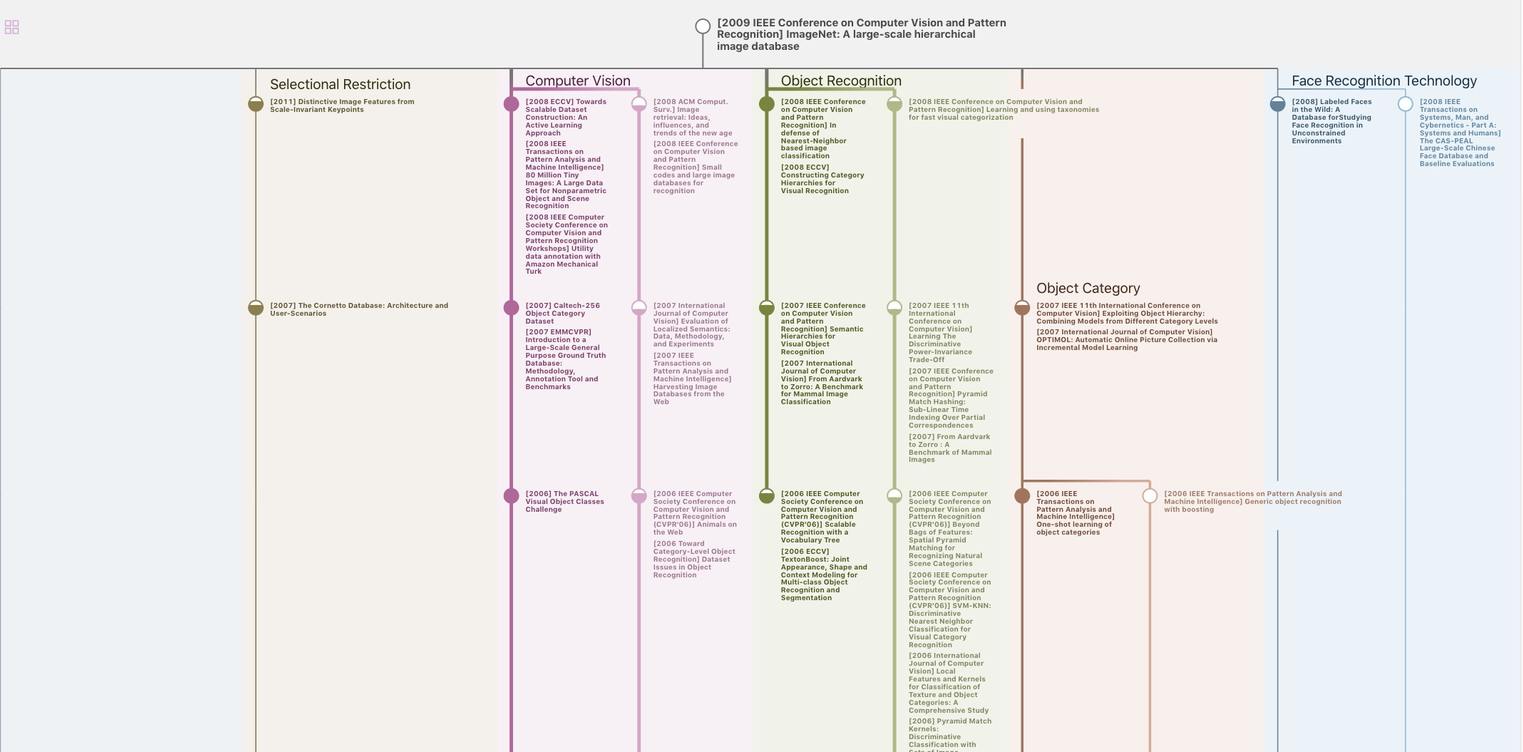
生成溯源树,研究论文发展脉络
Chat Paper
正在生成论文摘要