A Probabilistic Model for User Interest Propagation in Recommender Systems
IEEE ACCESS(2020)
摘要
User interests modeling has been exploited as a critical component to improve the predictive performance of recommender systems. However, with the absence of explicit information to model user interests, most approaches to recommender systems exploit users activities (user generated contents or user ratings) to inference the interest of users. In reality, the relationship among users also serves as a rich source of information of shared interest. To this end, we propose a framework which avoids the sole dependence of user activities to infer user interests and allows the exploitation of the direct relationship between users to propagate user interests to improve system's performance. In this paper, we advocate a novel modeling framework. We construct a probabilistic user interests model and propose a user interests propagation algorithm (UIP), which applies a factor graph based approach to estimate the distribution of the interests of users. Moreover, we incorporate our UIP algorithm with conventional matrix factorization (MF) for recommender systems. Experimental results demonstrate that our proposed approach outperforms previous methods used for recommender systems.
更多查看译文
关键词
Social networking (online),Recommender systems,Prediction algorithms,User-generated content,Probabilistic logic,Computational modeling,Sum product algorithm,Propagation,recommender system,sum-product algorithm,user interest modeling
AI 理解论文
溯源树
样例
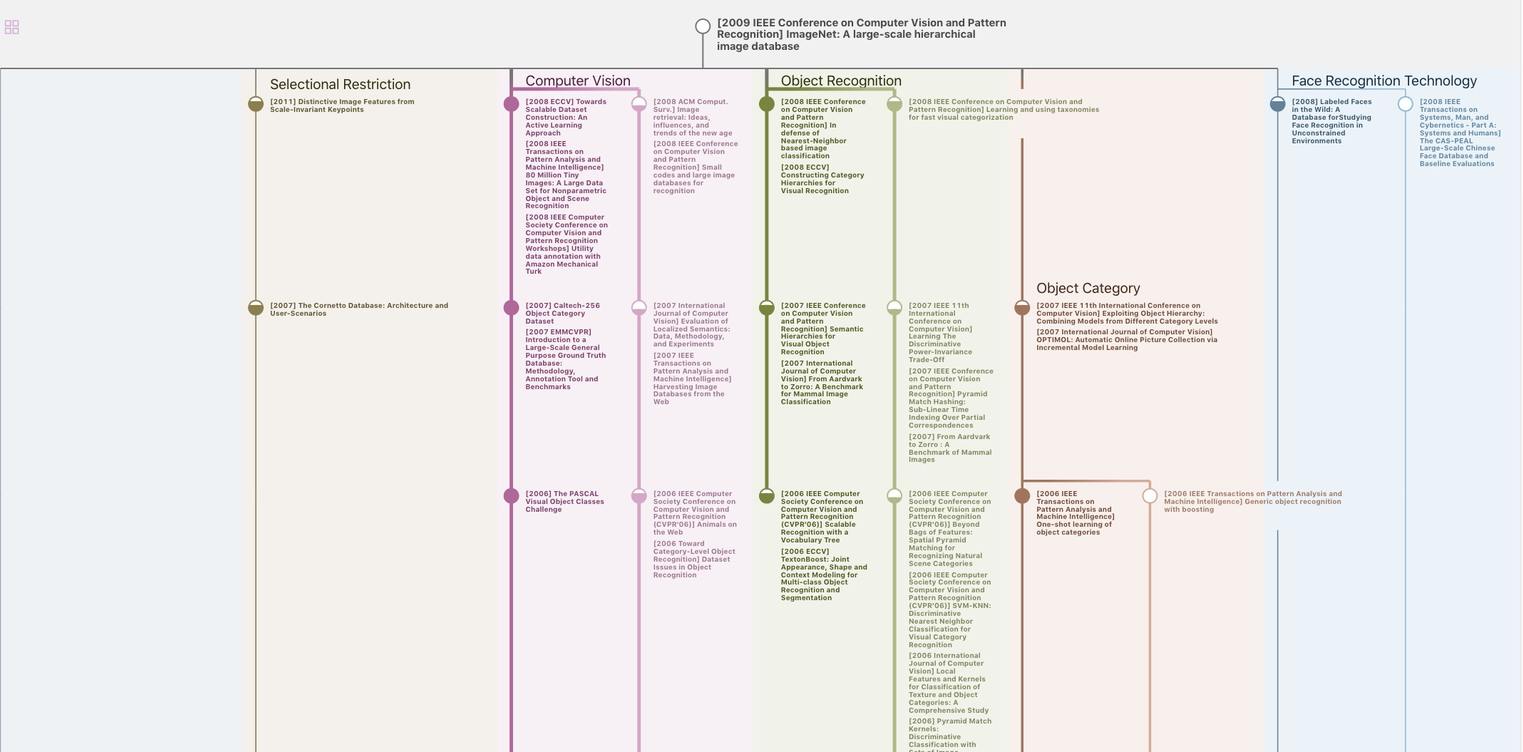
生成溯源树,研究论文发展脉络
Chat Paper
正在生成论文摘要