The Forecasting of Evolutionary Multi-Source Data limits
PROCEEDINGS OF THE 6TH INTERNATIONAL CONFERENCE ON INFORMATION ENGINEERING FOR MECHANICS AND MATERIALS(2016)
摘要
This paper gives a method of forecasting data evolution in a long term, its practical significance is predicting data evolution direction and targeting the data that need to be updated. With the assumption of no absolutly real data in the world, we could view the data updating process as a data evolution in order to infinitely close to an objective entity. The EVO elements could be considered as the most close to real data in a specified period. In the long term, data evolution constitutes a Markov chain. According to Chapman-K Olmogorov Equation, we derive the Markov chains of data evolution in the long term. Furthermore, we greatly simplifies the evolutionary computation by a properties of limiting distribution and the EVO elements. Experiments show that after a great times of updating, it still has a high prediction precision for the initial price.
更多查看译文
关键词
Data Evolution,Dynamic Evolution Factor,Evolutionary Matrix,Limit Distribution
AI 理解论文
溯源树
样例
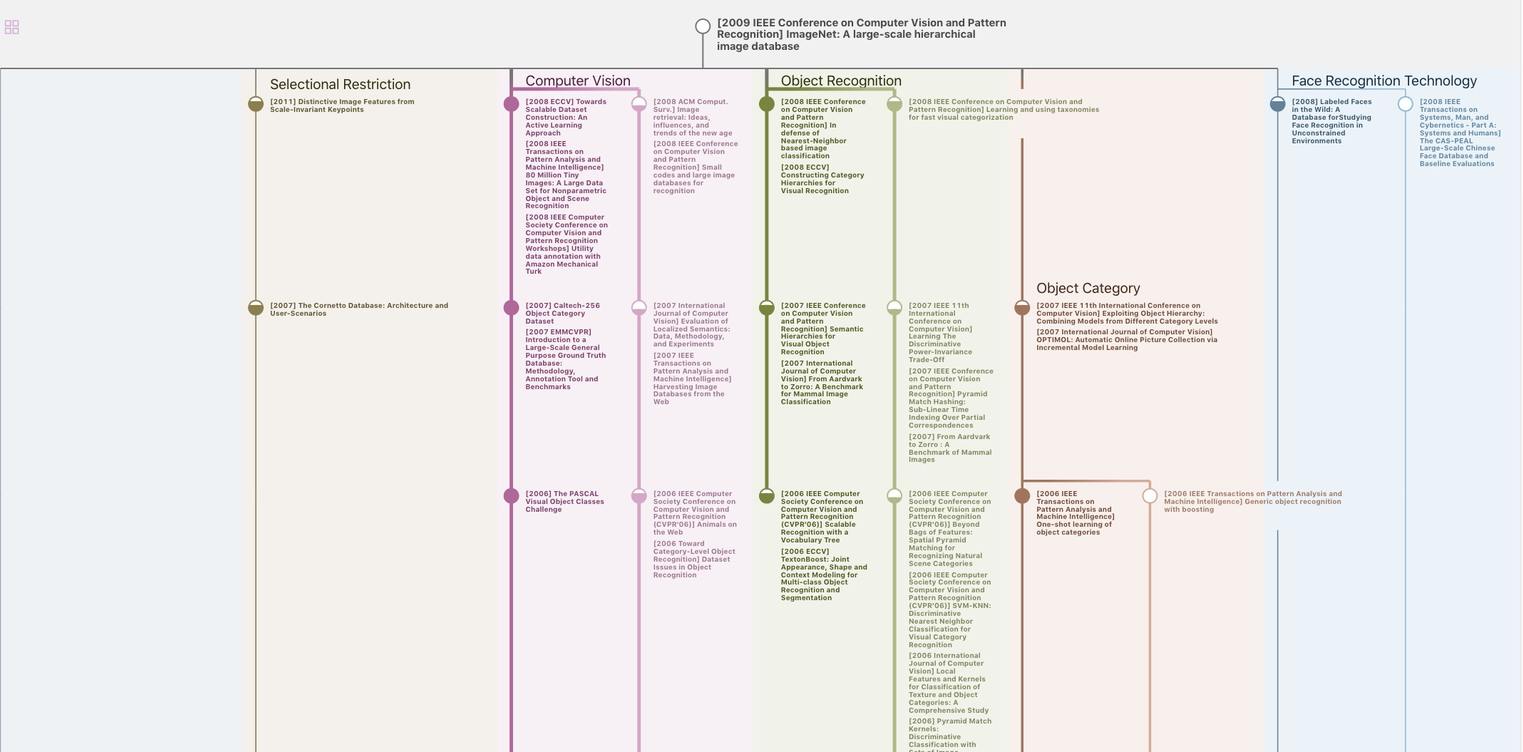
生成溯源树,研究论文发展脉络
Chat Paper
正在生成论文摘要