Deep learning–based image reconstruction for brain CT: improved image quality compared with adaptive statistical iterative reconstruction-Veo (ASIR-V)
NEURORADIOLOGY(2020)
摘要
Purpose To compare the image quality of brain computed tomography (CT) images reconstructed with deep learning–based image reconstruction (DLIR) and adaptive statistical iterative reconstruction-Veo (ASIR-V). Methods Sixty-two patients underwent routine noncontrast brain CT scans and datasets were reconstructed with 30% ASIR-V and DLIR with three selectable reconstruction strength levels (low, medium, high). Objective parameters including CT attenuation, noise, noise reduction rate, artifact index of the posterior cranial fossa, and contrast-to-noise ratio (CNR) were measured at the levels of the centrum semiovale and basal ganglia. Subjective parameters including gray matter-white matter differentiation, sharpness, and overall diagnostic quality were also assessed and compared with the interobserver agreement. Results There was a gradual reduction in the image noise and artifact index of the posterior cranial fossa as the strength levels of DLIR increased (all P < 0.001) compared with that of ASIR-V. CNR in both the centrum semiovale and basal ganglia levels also improved from the low to high strength levels of DLIR compared with that of ASIR-V (all P < 0.001). DLIR images with medium and high strength levels demonstrated the best subjective image quality scores among the reconstruction datasets. There was moderate to good interobserver agreement for the subjective image quality assessments with ASIR-V and DLIR. Conclusion On routine brain CT scans, optimized protocols with DLIR allowed significant reduction of noise and artifacts with improved subjective image quality compared with ASIR-V.
更多查看译文
关键词
Deep learning image reconstruction, Brain CT, Adaptive statistical iterative reconstruction-Veo, Image quality
AI 理解论文
溯源树
样例
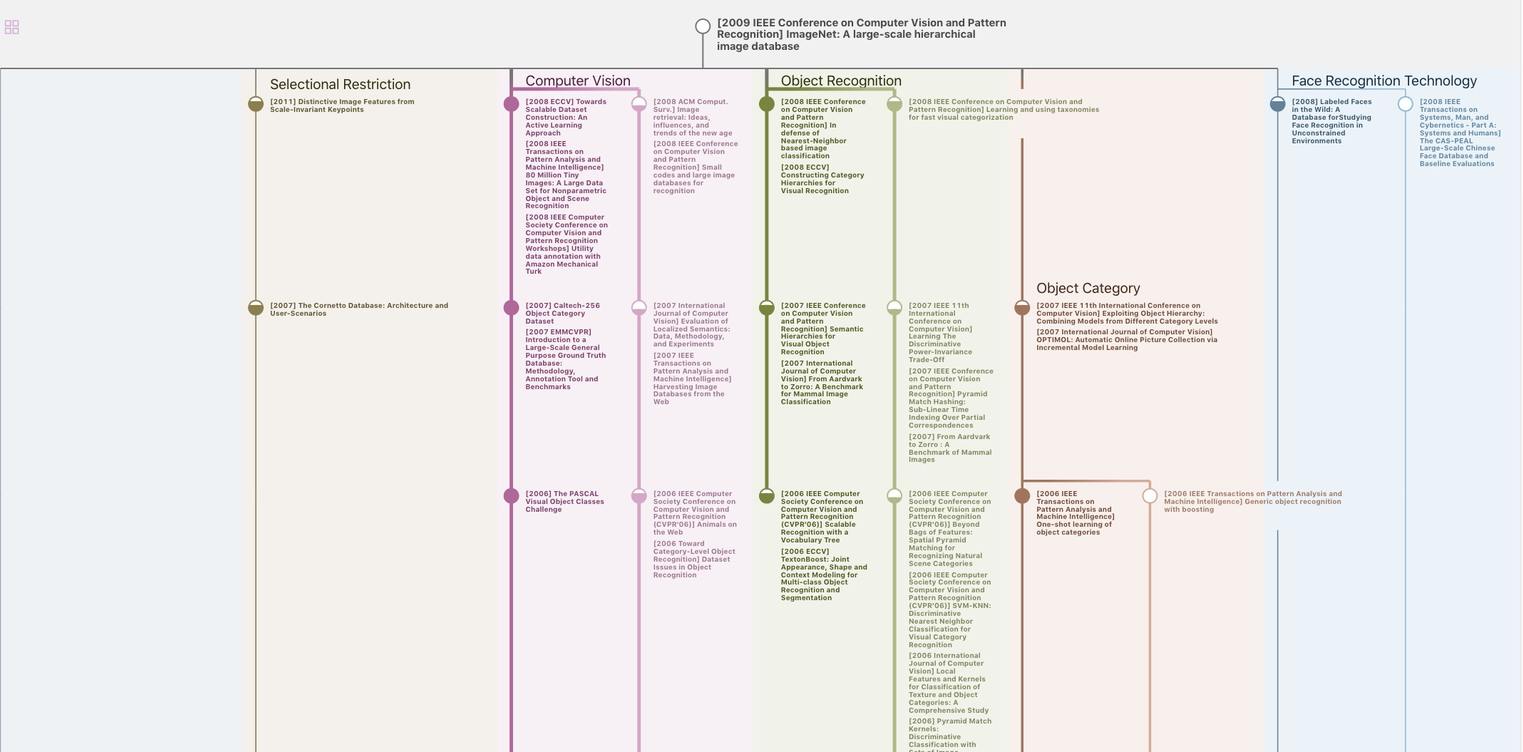
生成溯源树,研究论文发展脉络
Chat Paper
正在生成论文摘要