Copula-Based Uncertainty Quantification (Copula-UQ) for Multi-Sensor Data in Structural Health Monitoring
SENSORS(2020)
摘要
The problem of uncertainty quantification (UQ) for multi-sensor data is one of the main concerns in structural health monitoring (SHM). One important task is multivariate joint probability density function (PDF) modelling. Copula-based statistical inference has attracted significant attention due to the fact that it decouples inferences on the univariate marginal PDF of each random variable and the statistical dependence structure (called copula) among the random variables. This paper proposes the Copula-UQ, composing multivariate joint PDF modelling, inference on model class selection and parameter identification, and probabilistic prediction using incomplete information, for multi-sensor data measured from a SHM system. Multivariate joint PDF is modeled based on the univariate marginal PDFs and the copula. Inference is made by combing the idea of the inference functions for margins and the maximum likelihood estimate. Prediction on the PDF of the target variable, using the complete (from normal sensors) or incomplete information (due to missing data caused by sensor fault issue) of the predictor variable, are made based on the multivariate joint PDF. One example using simulated data and one example using temperature data of a multi-sensor of a monitored bridge are presented to illustrate the capability of the Copula-UQ in joint PDF modelling and target variable prediction.
更多查看译文
关键词
copula,missing data,multivariate random variable,probability density distribution,structural health monitoring
AI 理解论文
溯源树
样例
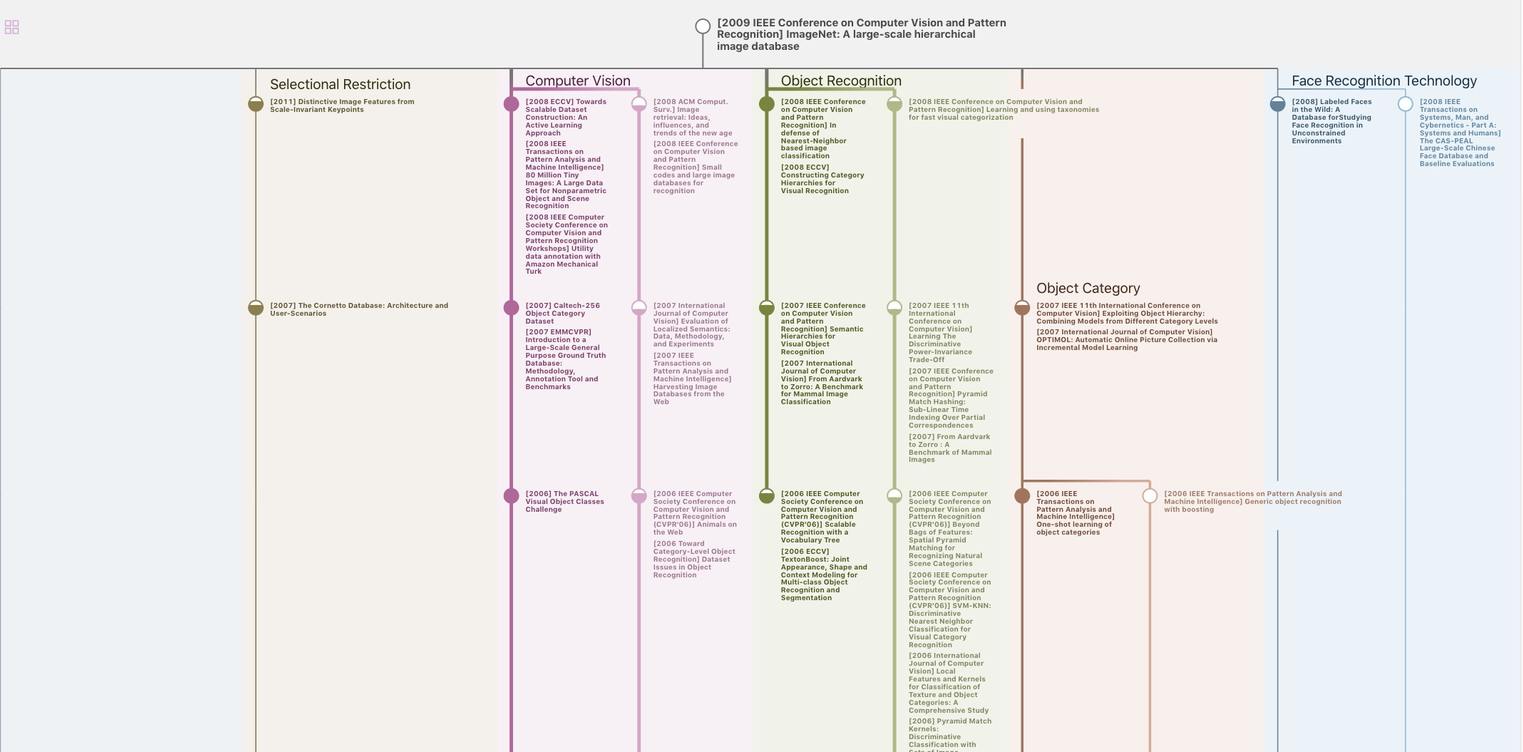
生成溯源树,研究论文发展脉络
Chat Paper
正在生成论文摘要