Deep Learning Approaches for Voice Activity Detection
CYBER SECURITY INTELLIGENCE AND ANALYTICS(2020)
摘要
This paper is involved with robustness for voice activity detection (VAD) approaches. The proposed approaches employ a few short term speech/non-speech discriminating characteristics to obtain a satisfactory performance in different environments. This paper mainly focuses on the performance improvement of recently proposed approaches which utilize spectral peak valley difference (SPVD) as a silence detection feature. The primary problem of this paper is to use a set of features with SPVD to improve the VAD robustness. The proposed approaches use deep learning approaches which are DNN, RNN and CNN, in order to analyze the robust VAD systems of the noise. The experiments show that the proposed deep learning approaches are compared with some other VAD techniques for better demonstration of its results in various noise and different SNRs circumstances. Applying the proposed approaches, the average of VAD performances are improved respectively to 89.72%, 95.01%, 92.05% for 5 diverse noise types. The result of LSTM performance is even 10.29% over than the method based on DNN and also 7.96% over than the CNN.
更多查看译文
关键词
VAD,Robustness,CNN,LSTM
AI 理解论文
溯源树
样例
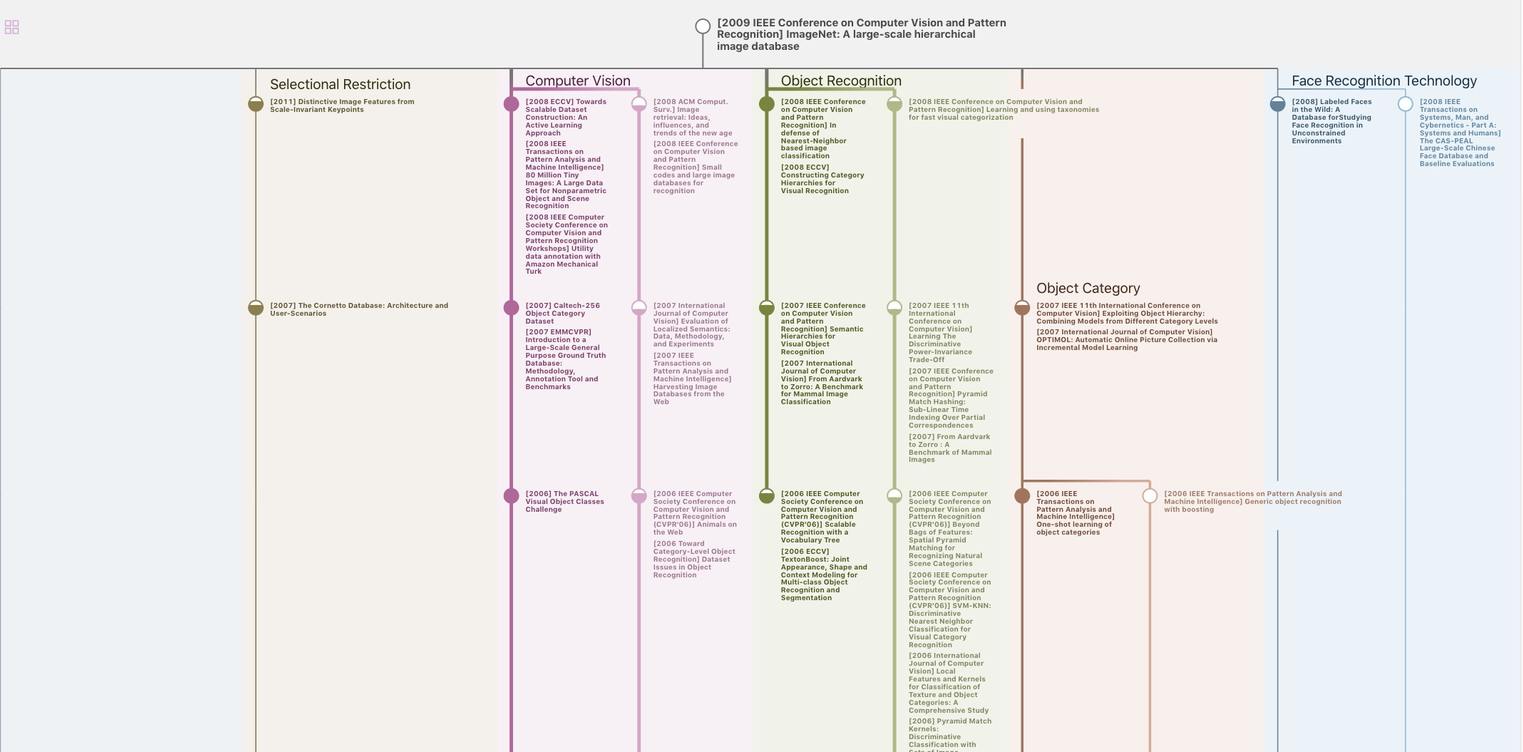
生成溯源树,研究论文发展脉络
Chat Paper
正在生成论文摘要