Recurrent Neural Networks with Grid Data Quantization for Modeling LHC Superconducting Magnets Behavior
INFORMATION TECHNOLOGY, SYSTEMS RESEARCH, AND COMPUTATIONAL PHYSICS(2020)
摘要
This paper presents a model based on Recurrent Neural Network architecture, in particular LSTM, for modeling the behavior of Large Hadron Collider superconducting magnets. High resolution data available in Post Mortem database was used to train a set of models and compare their performance with respect to various hyper-parameters such as input data quantization and number of cells. A novel approach to signal level quantization allowed to reduce a size of the model, simplify tuning of the magnet monitoring system and make the process scalable. The paper shows that RNNs such as LSTM or GRU may be used for modeling high resolution signals with an accuracy over 0.95 and as small number of the parameters ranging from 800 to 1200. This makes the solution suitable for hardware implementation essential in the case of monitoring performance critical and high speed signal of Large Hadron Collider superconducting magnets.
更多查看译文
关键词
LHC,RNN,GRU,LSTM,Signals modeling,Anomaly detection
AI 理解论文
溯源树
样例
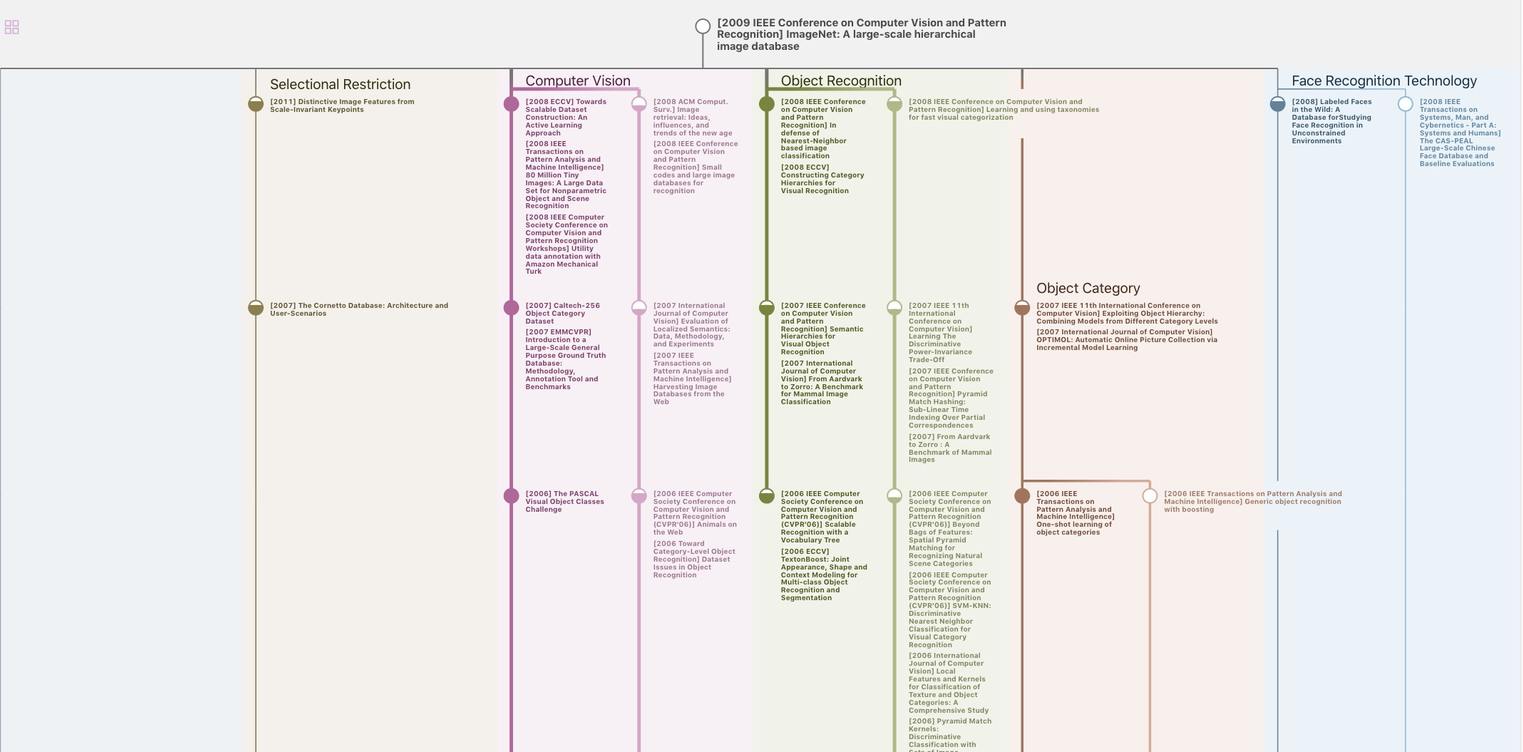
生成溯源树,研究论文发展脉络
Chat Paper
正在生成论文摘要