Reinforcement Learning for Traffic-Adaptive Sleep Mode Management in 5G Networks
2020 IEEE 31st Annual International Symposium on Personal, Indoor and Mobile Radio Communications(2020)
摘要
In mobile networks, base stations (BSs) have the largest share in energy consumption. To reduce BS energy consumption, BS components with similar (de)activation times can be grouped and put into sleep during their times of inactivity. The deeper and the more energy saving a sleep mode (SM) is, the longer (de)activation time it takes to wake up, which incurs a proportional service interruption. Therefore, it is challenging to timely decide on the best SM, bearing in mind the daily traffic fluctuation and imposed service level constraints on delay/dropping. In this study, we leverage an online reinforcement learning technique, i.e., SARSA, and propose an algorithm to decide which SM to choose given time and BS load. We use real mobile traffic obtained from a BS in Stockholm to evaluate the performance of the proposed algorithm. Simulation results show that considerable energy saving can be achieved at the cost of acceptable delay, i.e., wake-up time until we serve users, compared to two lower/upper baselines, namely, fixed (non-adaptive) SMs and optimal non-causal solution.
更多查看译文
关键词
5G,base station sleeping,discontinuous transmission,energy efficiency,reinforcement learning
AI 理解论文
溯源树
样例
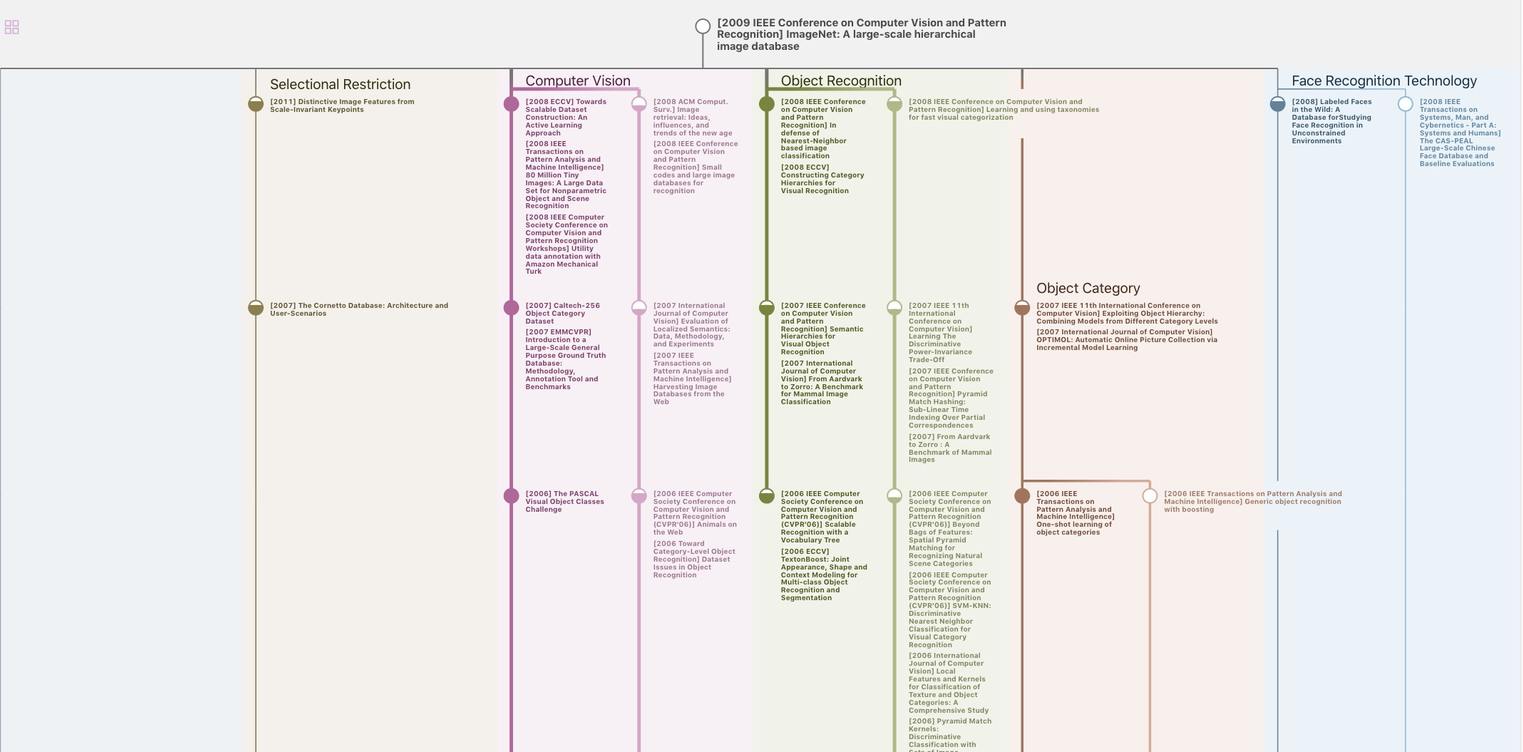
生成溯源树,研究论文发展脉络
Chat Paper
正在生成论文摘要