A Spatial–Temporal Positioning Algorithm Using Residual Network and LSTM
IEEE Transactions on Instrumentation and Measurement(2020)
Abstract
With the ever-increasing demand for location-based services in the indoor environments, Wi-Fi-based positioning technology has attracted much attention in decades of years because of its ubiquitous deployment and low cost. There is the fact that Wi-Fi signal not only changes with the distance away from the target, but also changes with time. To improve positioning accuracy and robustness, we consider both the spatial relation and temporal sequential relation simultaneously, and propose a spatial–temporal positioning algorithm that combines residual network and long short-term memory (LSTM) network. In this algorithm, to avoid the degradation problem, we adopt the residual-based network to extract the spatial features of the Wi-Fi signal at the same time slice. Furthermore, the LSTM is used to extract temporal features of the Wi-Fi signal among successive time slices. Finally, a fully connected layer is used to obtain the final location estimation. Extensive experiments on the IPIN2016 data sets demonstrate that our proposed algorithm can obtain 4.93-, 5.40-, 3.20-, and 4.98-m average positioning error on the UAH, CAR, UJIUB, and UJITI subdata set, respectively. The experimental results show that our proposed algorithm outperforms other state-of-the-art positioning algorithms with better accuracy and robustness.
MoreTranslated text
Key words
Wireless fidelity,Feature extraction,Fingerprint recognition,Residual neural networks,Deep learning,Robustness,Degradation
AI Read Science
Must-Reading Tree
Example
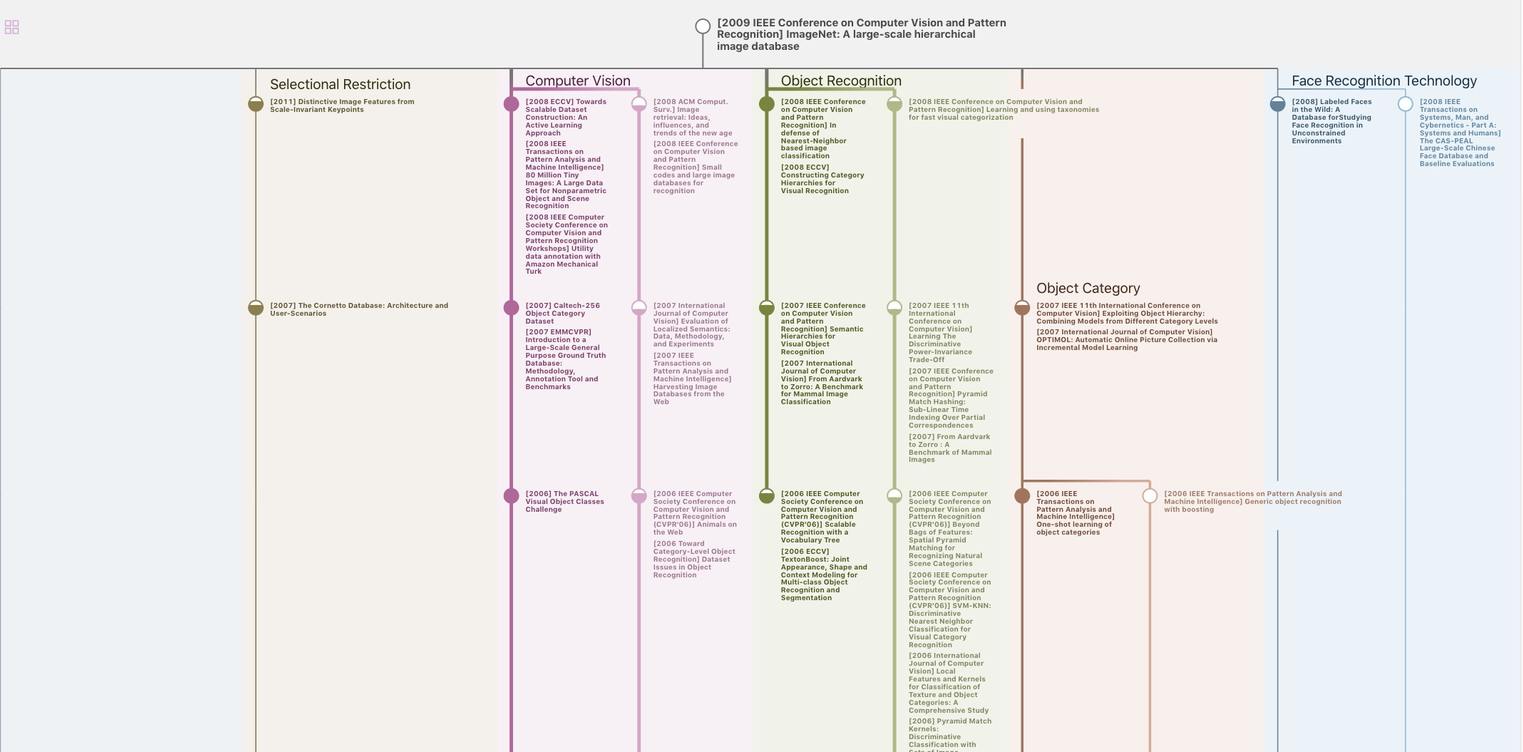
Generate MRT to find the research sequence of this paper
Chat Paper
Summary is being generated by the instructions you defined