Unsupervised Joint k-node Graph Representations with Compositional Energy-Based Models
NIPS 2020(2020)
摘要
Existing Graph Neural Network (GNN) methods that learn inductive unsupervised graph representations focus on learning node and edge representations by predicting observed edges in the graph. Although such approaches have shown advances in downstream node classification tasks, they are ineffective in jointly representing larger k-node sets, k{>}2. We propose MHM-GNN, an inductive unsupervised graph representation approach that combines joint k-node representations with energy-based models (hypergraph Markov networks) and GNNs. To address the intractability of the loss that arises from this combination, we endow our optimization with a loss upper bound using a finite-sample unbiased Markov Chain Monte Carlo estimator. Our experiments show that the unsupervised joint k-node representations of MHM-GNN produce better unsupervised representations than existing approaches from the literature.
更多查看译文
关键词
graph representations,models,energy-based
AI 理解论文
溯源树
样例
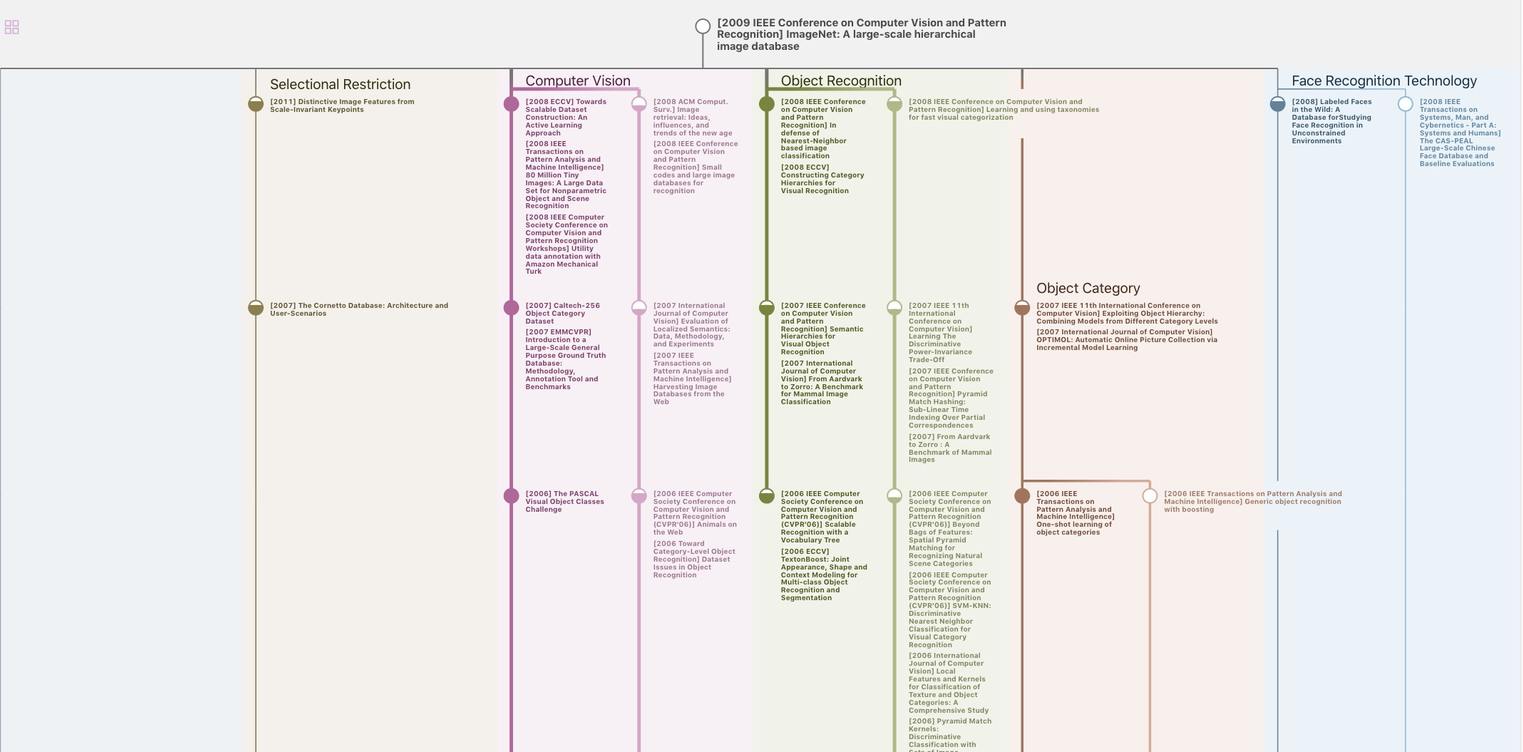
生成溯源树,研究论文发展脉络
Chat Paper
正在生成论文摘要