GreedyFool: Distortion-Aware Sparse Adversarial Attack
NIPS 2020(2020)
摘要
Modern deep neural networks(DNNs) are vulnerable to adversarial samples. Sparse adversarial samples are a special branch of adversarial samples that can fool the target model by only perturbing a few pixels. The existence of the sparse adversarial attack points out that DNNs are much more vulnerable than people believed, which is also a new aspect for analyzing DNNs. However, current sparse adversarial attack methods still have some shortcomings on both sparsity and invisibility. In this paper, we propose a novel two-stage distortion-aware greedy-based method dubbed as âÂÂGreedyFool". Specifically, it first selects the most effective candidate positions to modify by considering both the gradient(for adversary) and the distortion map(for invisibility), then drops some less important points in the reduce stage. Experiments demonstrate that compared with the start-of-the-art method, we only need to modify $3\times$ fewer pixels under the same sparse perturbation setting. For target attack, the success rate of our method is 9.96\% higher than the start-of-the-art method under the same pixel budget. Code can be found at https://github.com/LightDXY/GreedyFool.
更多查看译文
关键词
distortion-aware
AI 理解论文
溯源树
样例
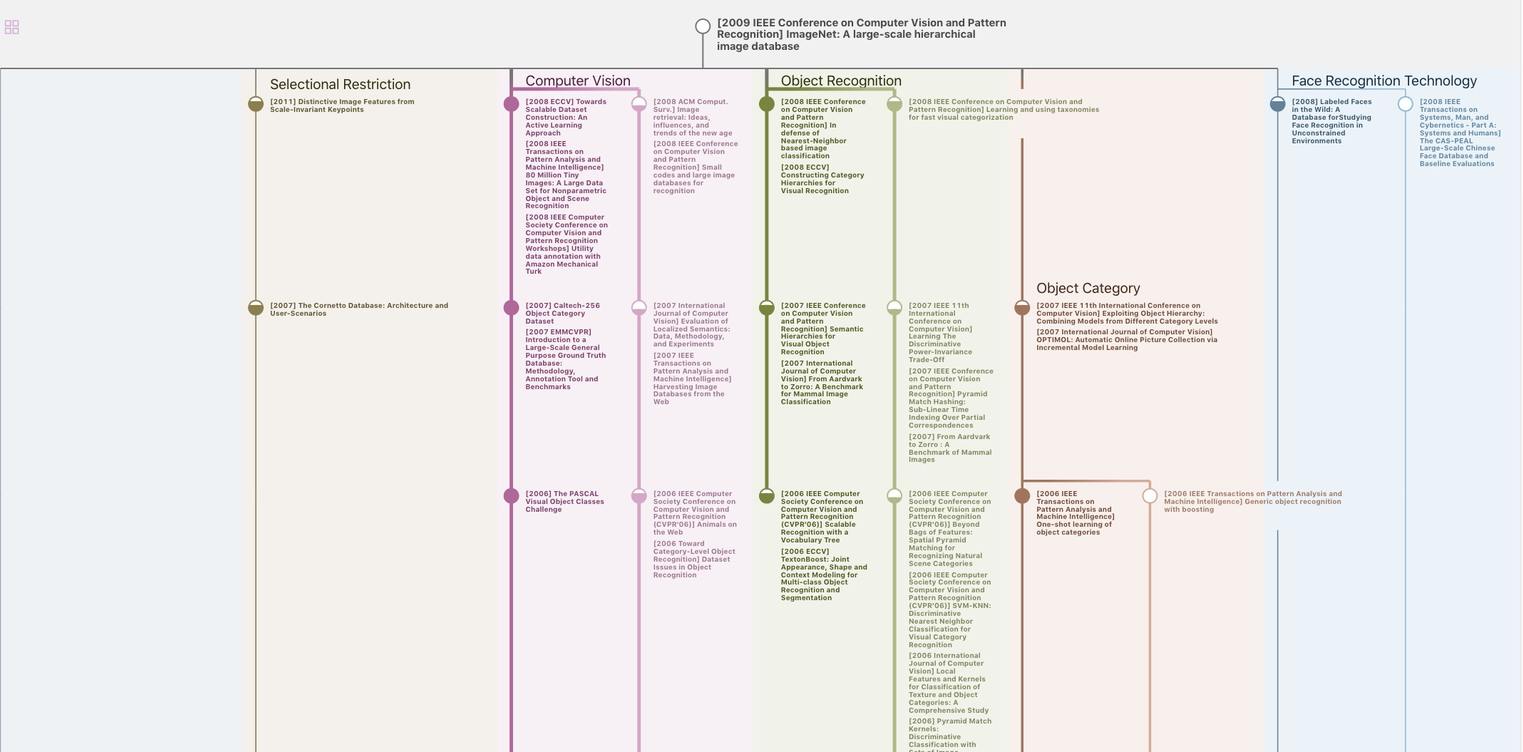
生成溯源树,研究论文发展脉络
Chat Paper
正在生成论文摘要