Sequential changepoint detection in neural networks with checkpoints
Statistics and Computing(2022)
摘要
We introduce a framework for online changepoint detection and simultaneous model learning which is applicable to highly parametrized models, such as deep neural networks. It is based on detecting changepoints across time by sequentially performing generalized likelihood ratio tests that require only evaluations of simple prediction score functions. This procedure makes use of checkpoints, consisting of early versions of the actual model parameters, that allow to detect distributional changes by performing predictions on future data. We define an algorithm that bounds the Type I error in the sequential testing procedure. We demonstrate the efficiency of our method in challenging continual learning applications with unknown task changepoints, and show improved performance compared to online Bayesian changepoint detection.
更多查看译文
关键词
Online changepoint detection,Generalized likelihood ratio tests,Neural networks,Continual learning
AI 理解论文
溯源树
样例
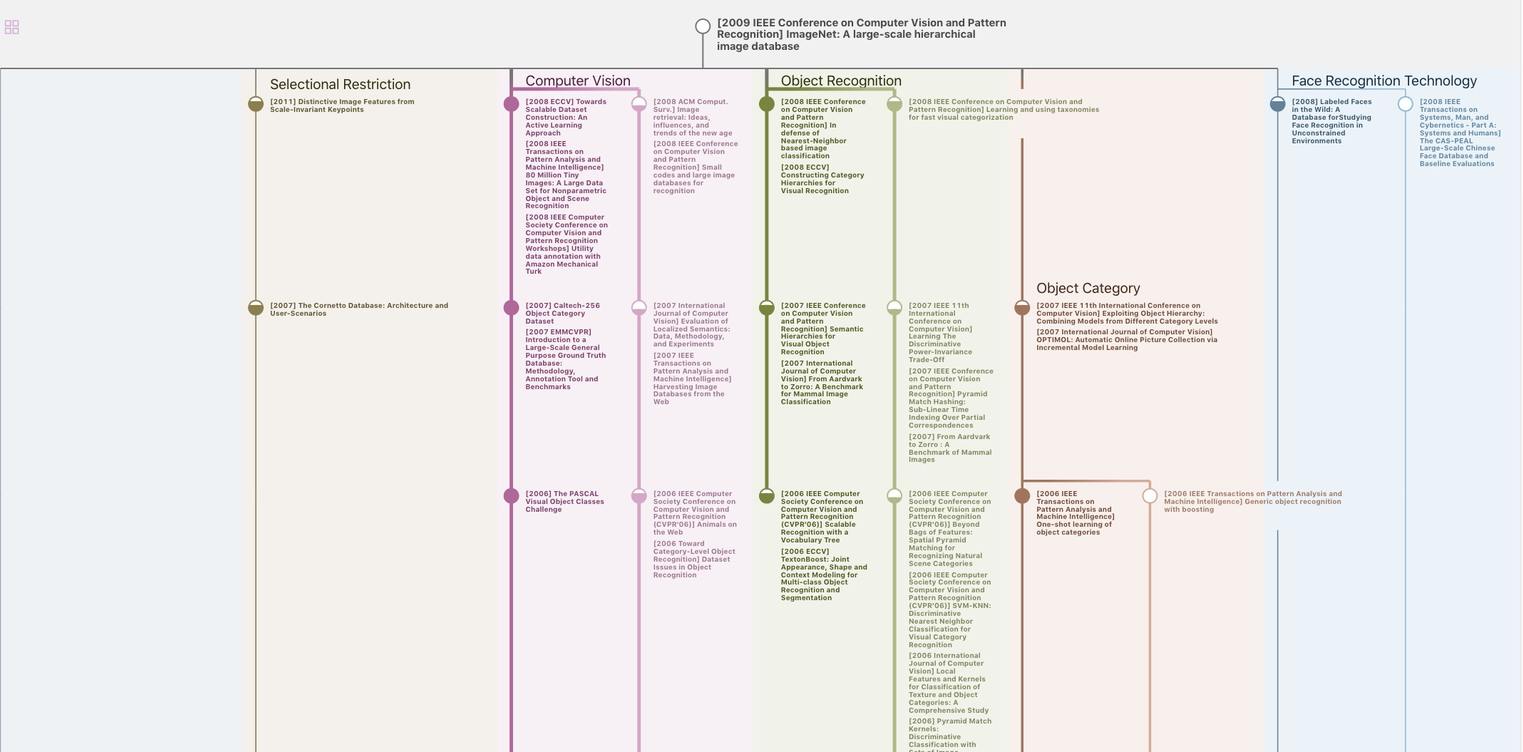
生成溯源树,研究论文发展脉络
Chat Paper
正在生成论文摘要