Zero-Inflated Spatial Models: Application and Interpretation
ADVANCES AND CHALLENGES IN PARAMETRIC AND SEMI-PARAMETRIC ANALYSIS FOR CORRELATED DATA(2016)
Abstract
Many environmental applications, such as species abundance studies, rainfall monitoring or tornado count reports, yield data with a preponderance of zero counts. Although standard statistical distributions may not fit these data, a large body of literature has been dedicated to methods for modeling zero-inflated data. One type of regression model for zero-inflated data is categorized as a mixture model. Mixture models postulate two types of zeros, represented using a latent variable, and model their probabilities separately. The latent classification of zeros may be of particular interest as it can provide important clues to physical characteristics associated with, for example, habitat suitability or resistance to disease or pest infestations. Different zero-inflated models can be developed depending on the biological and physical characteristics of the application at hand. Here, several zero-inflated spatial models are applied to a case study of spruce weevil (Pissodes-strobi) infestations in a Sitka spruce tree plantation. The data illustrate the unique features distinguished by various models and show the importance of using expert knowledge to inform model structures that in turn provide insight into underlying biological processes driving the probability of belonging to the zero, resistant, component. For instance, one model focuses on individually resistant trees located among infested trees. Another focuses on clusters of resistant trees which are likely located in unsuitable habitats. We apply six models: a standard generalized linear model (GLM); an overdispersion model; a random effects zero-inflated model; a conditional autoregressive random effects model (CAR); a multivariate CAR (MCAR) model; and a model developed using discrete random effects to accommodate spatial outliers. We discuss the distinct features identified by the zero-inflated spatial models and make recommendations regarding their application in general.
MoreTranslated text
Key words
Autocovariate,Discrete mixture model,Hierarchical Bayesian model,Mixed binomial model,Spatial autocorrelation,Spruce weevil
AI Read Science
Must-Reading Tree
Example
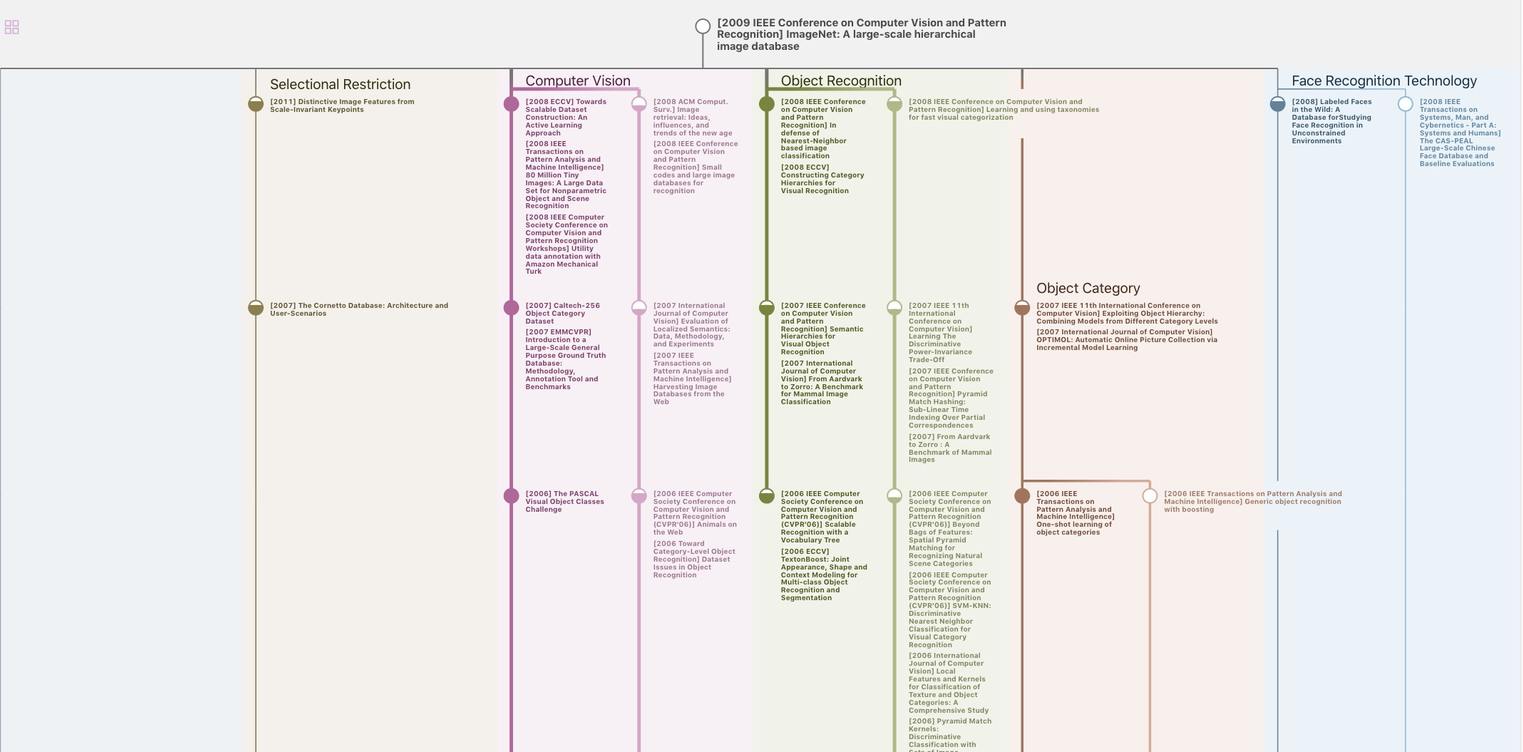
Generate MRT to find the research sequence of this paper
Chat Paper
Summary is being generated by the instructions you defined