Context Modeling with Evidence Filter for Multiple Choice Question Answering.
IEEE International Conference on Acoustics, Speech, and Signal Processing (ICASSP)(2022)
摘要
Multiple-Choice Question Answering (MCQA) is a challenging task in machine reading comprehension. The main challenge in MCQA is to extract "evidence" from the given context that supports the correct answer. In the OpenbookQA dataset, the requirement of extracting "evidence" is particularly important due to the mutual independence of sentences in the context. Existing work tackles this problem by annotated evidence or distant supervision with rules which overly rely on human efforts. To address the challenge, we propose a simple yet effective approach termed evidence filtering to model the relationships between the encoded contexts with respect to different options collectively and to potentially highlight the evidence sentences and filter out unrelated sentences. In addition to the effective reduction of human efforts of our approach compared, through extensive experiments on OpenbookQA, we show that the proposed approach outperforms the models that use the same backbone and more training data; and our parameter analysis also demonstrates the interpretability of our approach.
更多查看译文
关键词
Natural Language Processing,Machine Reading Comprehension,Question Answering,Evidence Extraction
AI 理解论文
溯源树
样例
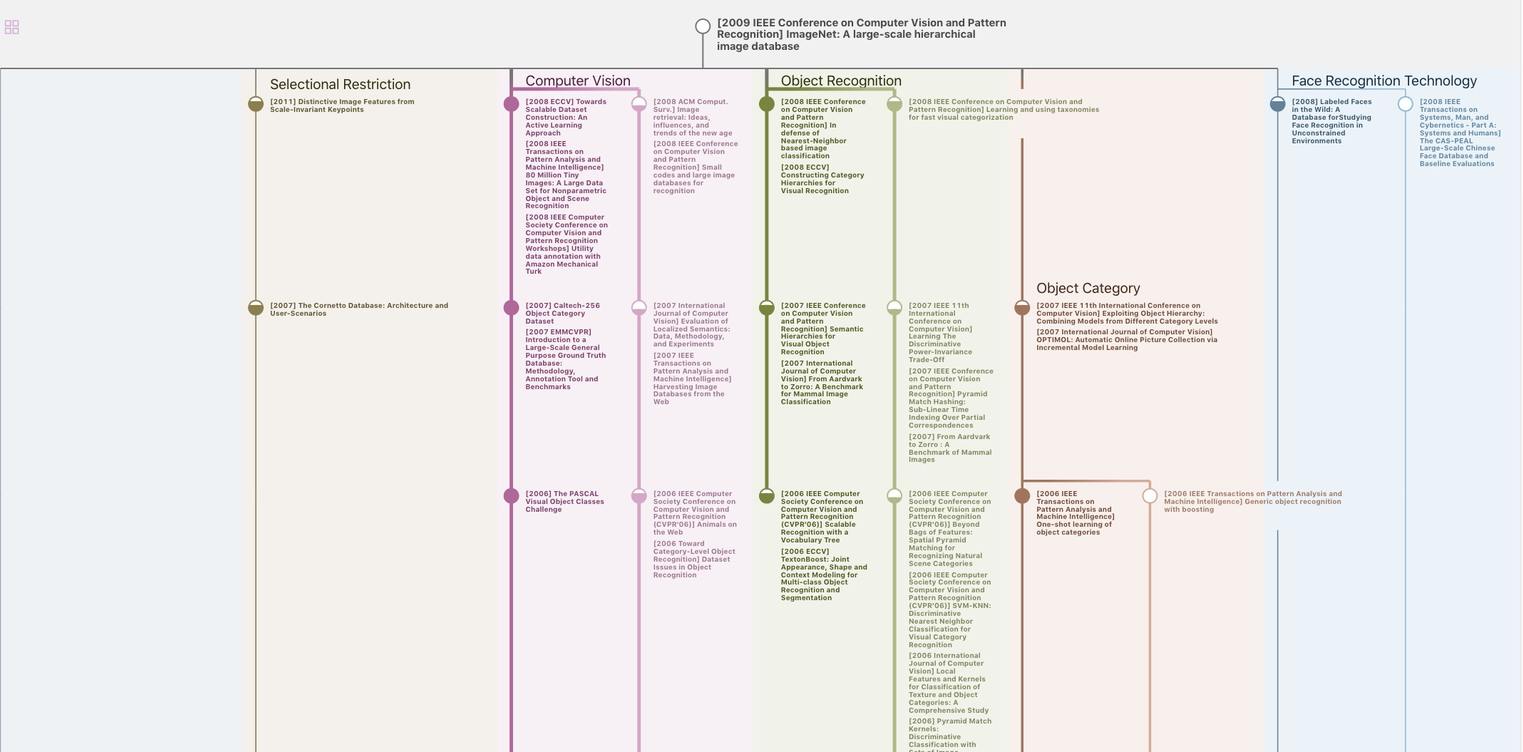
生成溯源树,研究论文发展脉络
Chat Paper
正在生成论文摘要