Predicting Early Stage Drug Induced Parkinsonism Using Unsupervised And Supervised Machine Learning
42ND ANNUAL INTERNATIONAL CONFERENCES OF THE IEEE ENGINEERING IN MEDICINE AND BIOLOGY SOCIETY: ENABLING INNOVATIVE TECHNOLOGIES FOR GLOBAL HEALTHCARE EMBC'20(2020)
摘要
Drug Induced Parkinsonism (DIP) is the most common, debilitating movement disorder induced by antipsychotics. There is no tool available in clinical practice to effectively diagnose the symptoms at the onset of the disease. In this study, the variations in gait accelerometer data due to the intermittency of tremor at the initial stages is examined. These variations are used to train a logistic regression model to predict subjects with early-stage DIP. The logistic classifier predicts if a subject is a DIP or control with approximately 89% sensitivity and 96% specificity. This paper discusses the algorithm used to extract the features in gait data for training the classifier to predict DIP at the earliest.
更多查看译文
关键词
Algorithms,Humans,Logistic Models,Parkinson Disease, Secondary,Supervised Machine Learning,Tremor
AI 理解论文
溯源树
样例
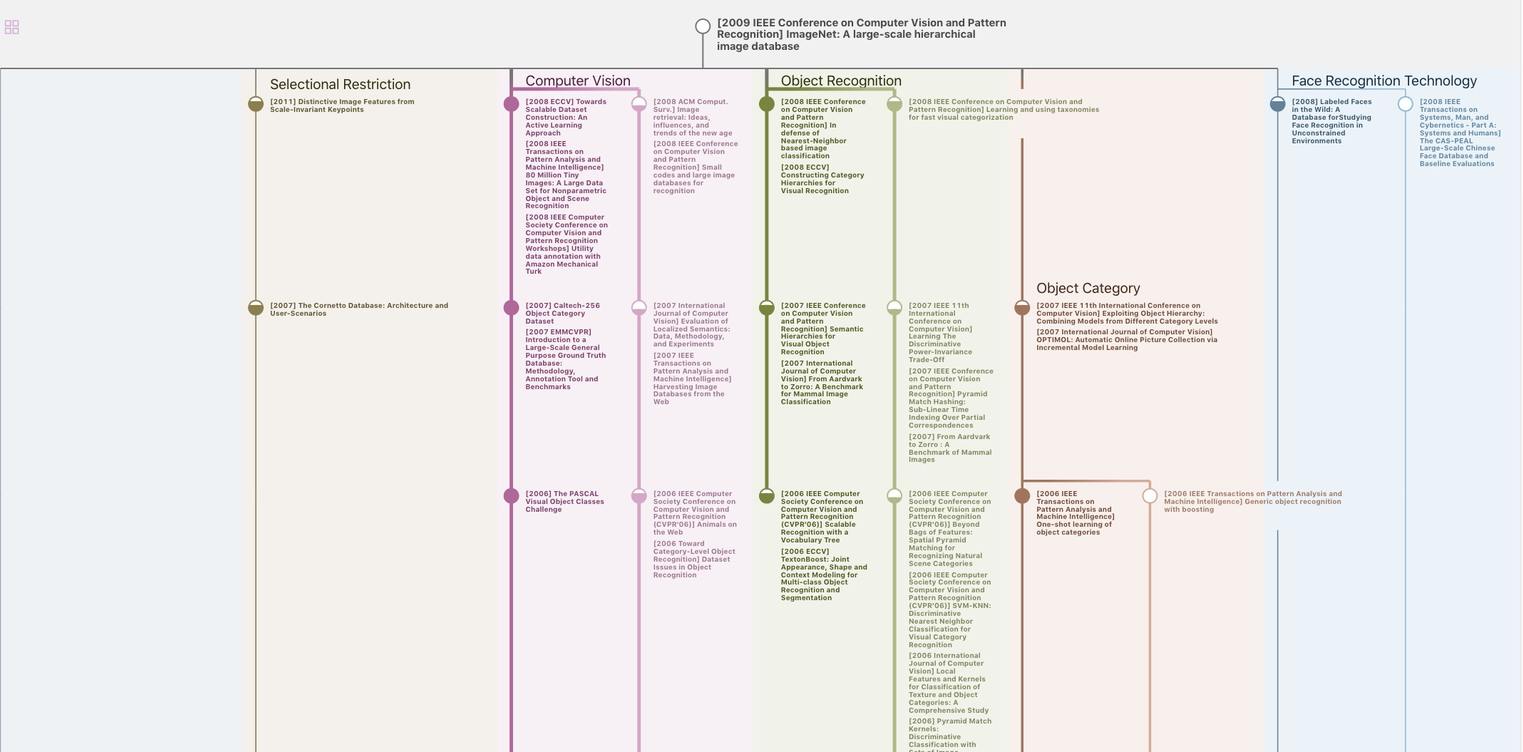
生成溯源树,研究论文发展脉络
Chat Paper
正在生成论文摘要