Neuromuscular Disease Detection Employing Deep Feature Extraction From Cross Spectrum Images Of Electromyography Signals
42ND ANNUAL INTERNATIONAL CONFERENCES OF THE IEEE ENGINEERING IN MEDICINE AND BIOLOGY SOCIETY: ENABLING INNOVATIVE TECHNOLOGIES FOR GLOBAL HEALTHCARE EMBC'20(2020)
摘要
In this paper, a deep learning framework for detection and classification of EMG signals for diagnosis of neuromuscular disorders is proposed employing cross wavelet transform. Cross wavelet transform which is a modification of continuous wavelet transform is an important tool to analyze any non-stationary signal in time scale and in time-frequency frame. To this end, EMG signals of healthy, myopathy and Amyotrophic lateral sclerosis disorders were procured from an online existing database. A healthy EMG signal was chosen as reference and cross wavelet transform of the rest of the healthy as well as the disease EMG signals was done with the reference. From the resulting cross wavelet spectrum images of EMG signals, a convolution neural network (CNN) based automated deep feature extraction technique was implemented. The extracted deep features were further subjected to feature ranking employing one way analysis of variance (ANOVA) test. The extracted deep features with high degree of statistical significance were fed to several benchmark machine learning classifiers for the purpose of discrimination of EMG signals. Two binary classification problems are addressed in this paper and it has been observed that the highest mean classification accuracy of 100% is achieved using the statistically significant extracted deep features. The proposed method can be implemented for real-time detection of neuromuscular disorders.
更多查看译文
关键词
Convolution neural network, classification, cross-wavelet transform and electromyography
AI 理解论文
溯源树
样例
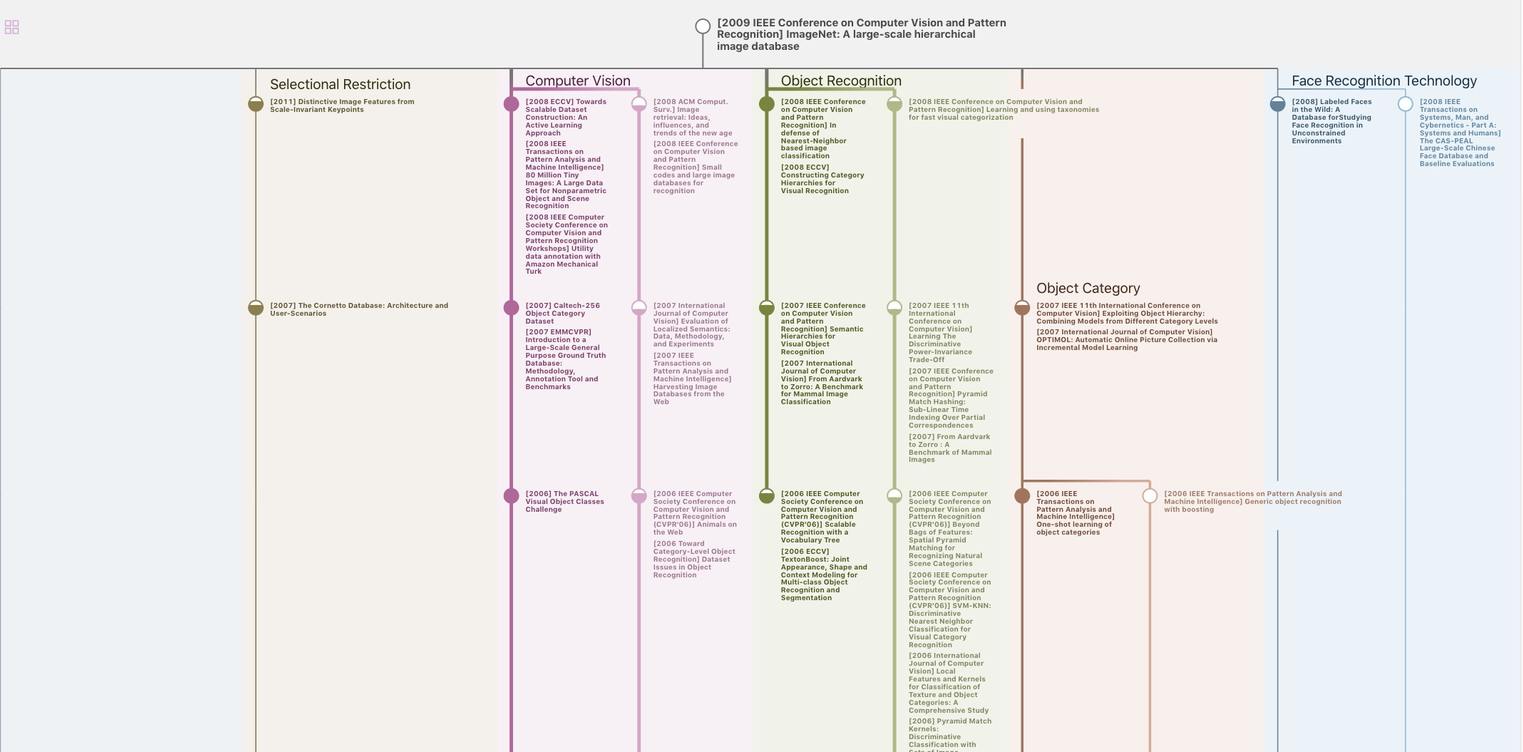
生成溯源树,研究论文发展脉络
Chat Paper
正在生成论文摘要