A Reinforcement Learning Approach for Rebalancing Electric Vehicle Sharing Systems
IEEE TRANSACTIONS ON INTELLIGENT TRANSPORTATION SYSTEMS(2022)
摘要
This paper proposes a reinforcement learning approach for nightly offline rebalancing operations in free-floating electric vehicle sharing systems (FFEVSS). Due to sparse demand in a network, FFEVSS requires relocation of electrical vehicles (EVs) to charging stations and demander nodes, which is typically done by a group of drivers. A shuttle is used to pick up and drop off drivers throughout the network. The objective of this study is to solve the shuttle routing problem to finish the rebalancing work in minimal time. We consider a reinforcement learning framework for the problem, in which a central controller determines the routing policies of a fleet of multiple shuttles. We deploy a policy gradient method for training recurrent neural networks and compare the obtained policy results with heuristic solutions. Our numerical studies show that unlike the existing solutions in the literature, the proposed methods allow solving the general version of the problem with no restrictions on the urban EV network structure and charging requirements of EVs. Moreover, the learned policies offer a wide range of flexibility, resulting in a significant reduction in the time needed to rebalance the network.
更多查看译文
关键词
Shared mobility, reinforcement learning, neural combinatorial optimization, vehicle routing
AI 理解论文
溯源树
样例
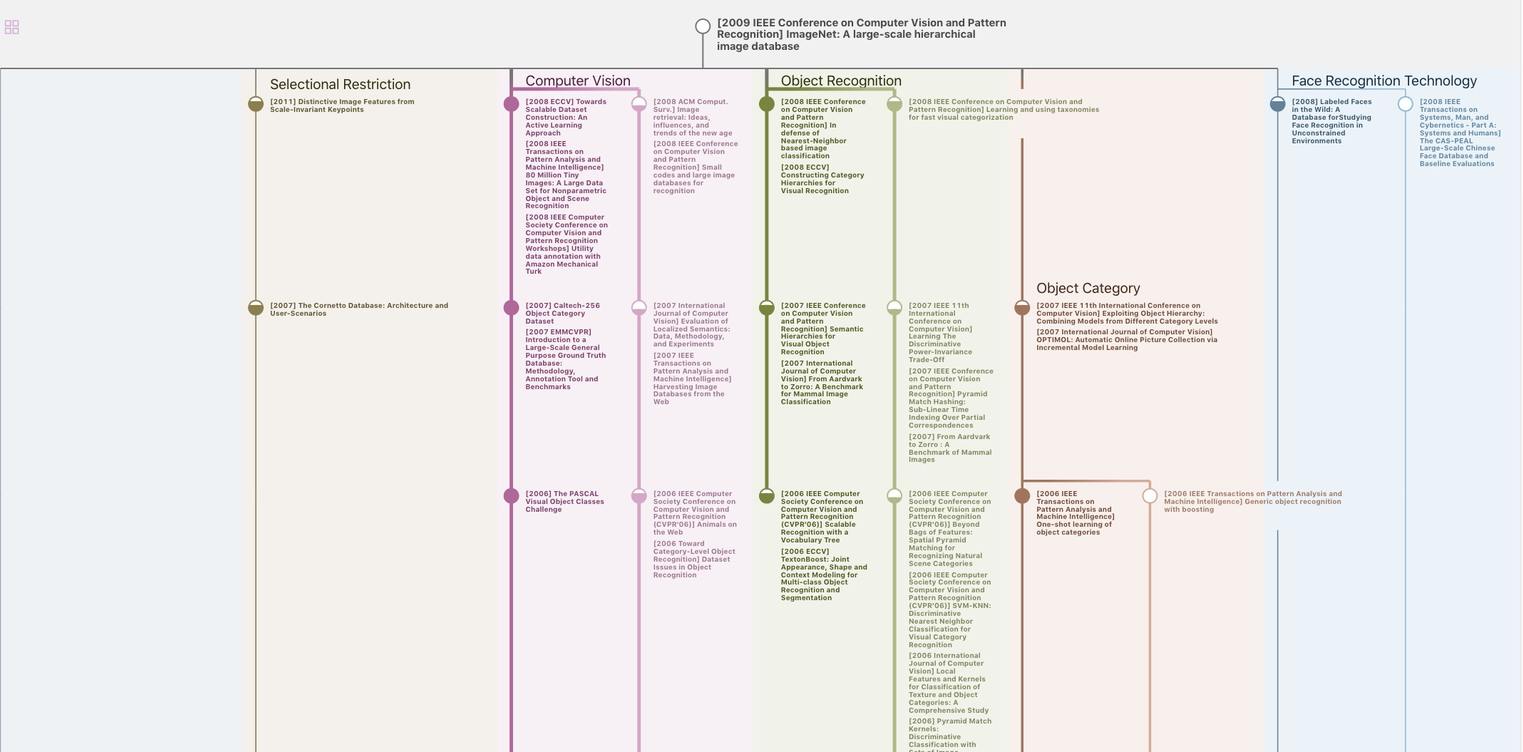
生成溯源树,研究论文发展脉络
Chat Paper
正在生成论文摘要