Learning to Generalize for Sequential Decision Making
EMNLP(2020)
摘要
We consider problems of making sequences of decisions to accomplish tasks, interacting via the medium of language. These problems are often tackled with reinforcement learning approaches. We find that these models do not generalize well when applied to novel task domains. However, the large amount of computation necessary to adequately train and explore the search space of sequential decision making, under a reinforcement learning paradigm, precludes the inclusion of large contextualized language models, which might otherwise enable the desired generalization ability. We introduce a teacher-student imitation learning methodology and a means of converting a reinforcement learning model into a natural language understanding model. Together, these methodologies enable the introduction of contextualized language models into the sequential decision making problem space. We show that models can learn faster and generalize more, leveraging both the imitation learning and the reformulation. Our models exceed teacher performance on various held-out decision problems, by up to 7% on in-domain problems and 24% on out-of-domain problems.
更多查看译文
关键词
generalize,learning,decision
AI 理解论文
溯源树
样例
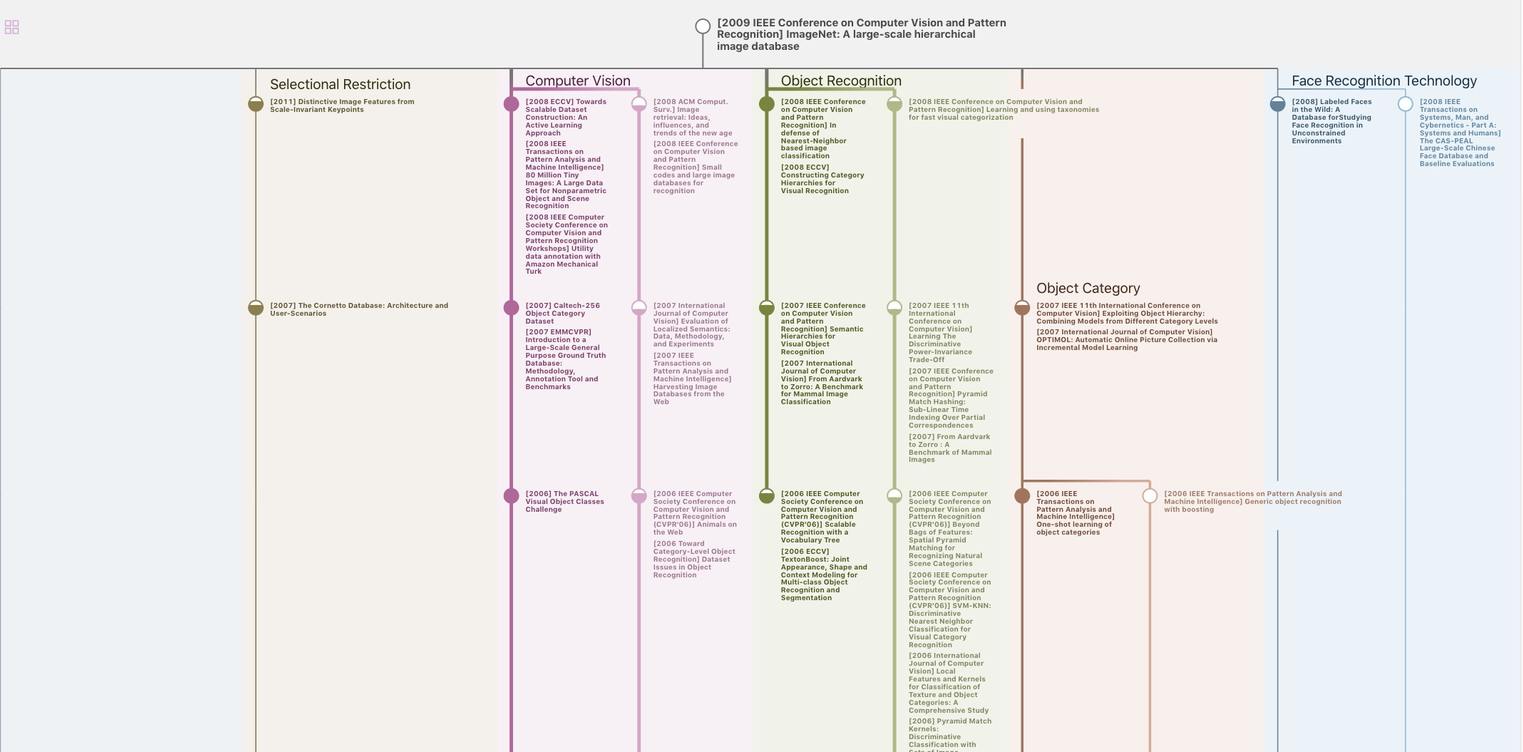
生成溯源树,研究论文发展脉络
Chat Paper
正在生成论文摘要