CopulaGNN: Towards Integrating Representational and Correlational Roles of Graphs in Graph Neural Networks
ICLR(2021)
摘要
Graph-structured data are ubiquitous. However, graphs encode diverse types of information and thus play different roles in data representation. In this paper, we distinguish the \textit{representational} and the \textit{correlational} roles played by the graphs in node-level prediction tasks, and we investigate how Graph Neural Network (GNN) models can effectively leverage both types of information. Conceptually, the representational information provides guidance for the model to construct better node features; while the correlational information indicates the correlation between node outcomes conditional on node features. Through a simulation study, we find that many popular GNN models are incapable of effectively utilizing the correlational information. By leveraging the idea of the copula, a principled way to describe the dependence among multivariate random variables, we offer a general solution. The proposed Copula Graph Neural Network (CopulaGNN) can take a wide range of GNN models as base models and utilize both representational and correlational information stored in the graphs. Experimental results on two types of regression tasks verify the effectiveness of the proposed method.
更多查看译文
关键词
graphs neural networks,correlational roles
AI 理解论文
溯源树
样例
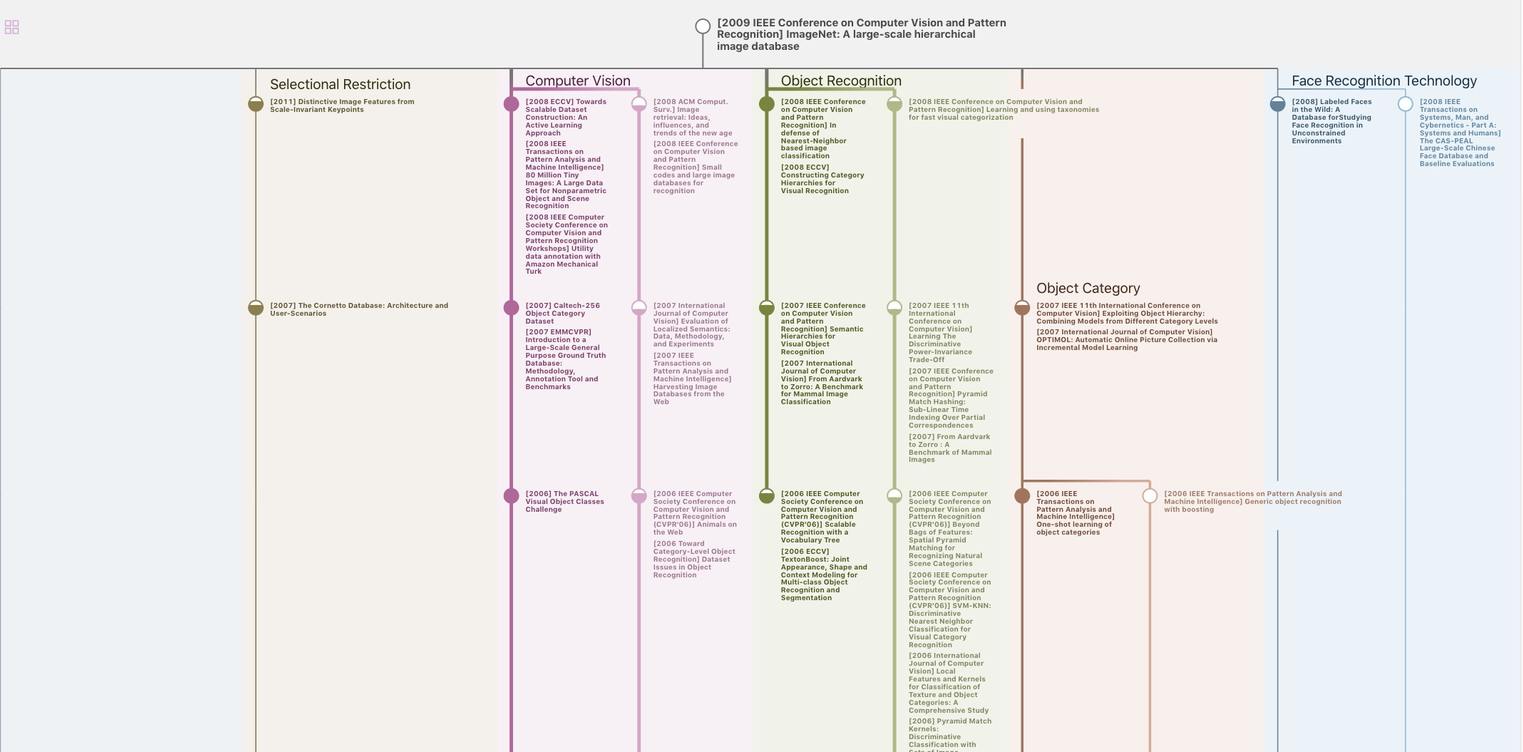
生成溯源树,研究论文发展脉络
Chat Paper
正在生成论文摘要