Composition analysis of PM 2.5 at multiple sites in Zhengzhou, China: implications for characterization and source apportionment at different pollution levels
Environmental Science and Pollution Research(2020)
Abstract
Zhengzhou is one of the most heavily polluted cities in China. This study collected samples of PM 2.5 (atmospheric fine particulate matter with aerodynamic diameter ≤ 2.5 μm) at five sites in different functional areas of Zhengzhou in 2016 to investigate the chemical properties and sources of PM 2.5 at three pollution levels, i.e., PM 2.5 ≤ 75 μg/m 3 (non-pollution, NP), 75 μg/m 3 < PM 2.5 ≤ 150 μg/m 3 (moderate pollution, MP), and PM 2.5 > 150 μg/m 3 (heavy pollution, HP). Chemical analysis was conducted, and source categories and potential source region were identified for PM 2.5 at different pollution levels. The health risks of toxic elements were evaluated. Results showed that the average PM 2.5 concentration in Zhengzhou was 119 μg/m 3 , and the sum of the concentrations of SO 4 2− , NO 3 − , and NH 4 + increased with the aggravation of pollution level (23, 42, and 114 μg/m 3 at NP, MP, and HP days, respectively). Positive Matrix Factorization analysis indicated that secondary aerosols, coal combustion, vehicle traffic, industrial processes, biomass burning, and dust were the main sources of PM 2.5 at three pollution levels, and accounted for 38.4%, 21.6%, 16.7%, 7.4%, 7.7%, and 8.1% on HP days, respectively. Trajectory clustering analysis showed that close-range transport was one of the dominant factors on HP days in Zhengzhou. The potential source areas were mainly located in Xinxiang, Kaifeng, Xuchang, and Pingdingshan. Significant risks existed in the non-carcinogenic risk of As (1.4–2.3) for children at three pollution levels and the non-carcinogenic risk of Pb (1.0–1.4) for children with NP and MP days.
MoreTranslated text
Key words
Fine particle, Pollution characteristics, Positive Matrix Factorization, Hybrid Single-Particle Lagrangian Integrated Trajectory Model, Enrichment factor, Health risk assessment
AI Read Science
Must-Reading Tree
Example
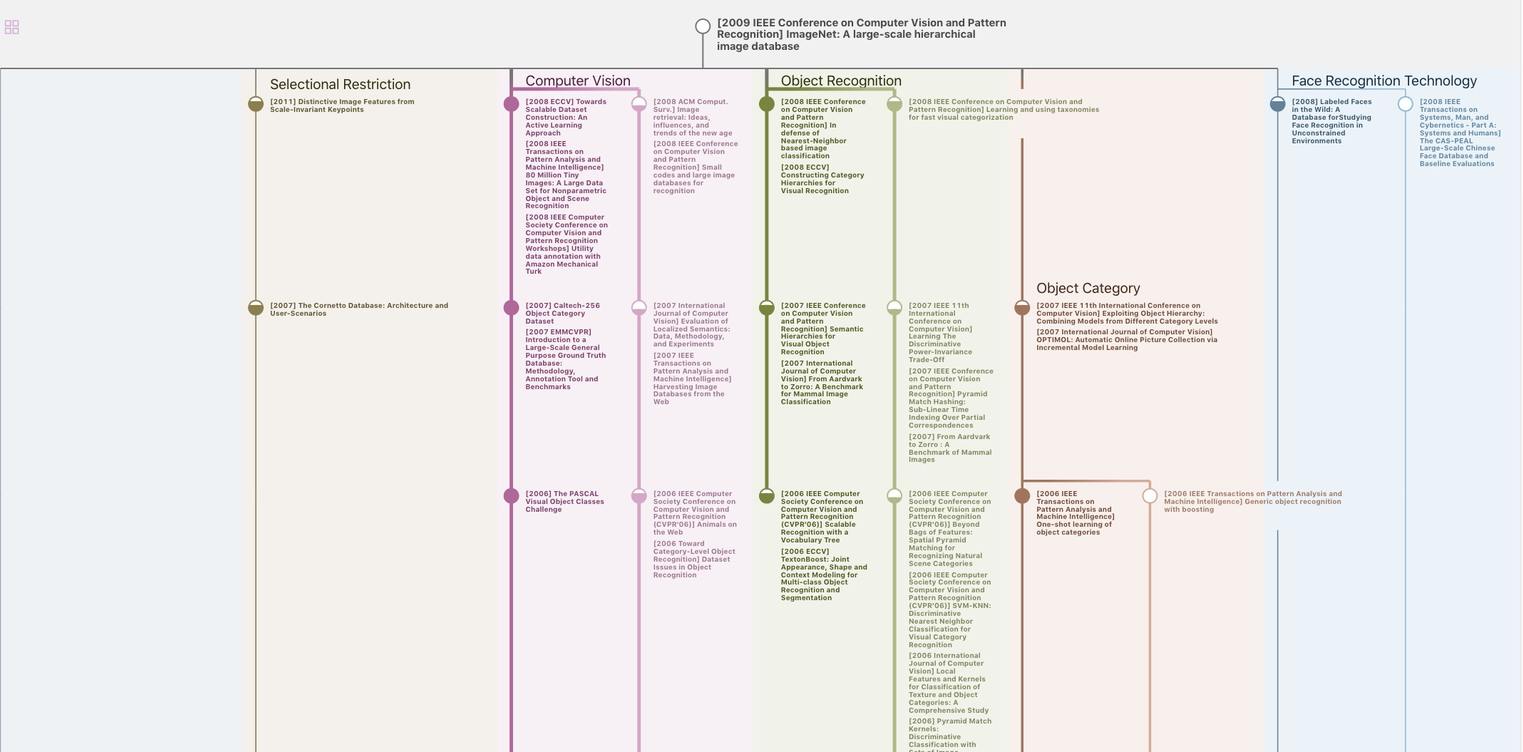
Generate MRT to find the research sequence of this paper
Chat Paper
Summary is being generated by the instructions you defined