Reinforcement learning using expectation maximization based guided policy search for stochastic dynamics
Neurocomputing(2022)
摘要
Guided policy search algorithms have been proven to work with incredible accuracy not only for controlling complicated dynamical systems, but also in learning optimal policies from exploration of various unseen instances. This paper deals with a trajectory optimization problem for an unknown dynamical system subject to measurement noise using expectation maximization and extends it to learning (optimal) policies which have less stochasticity in trajectories because of the higher exploitation efficiency. Theoretical and empirical evidence of learned optimal policies for the new approach is depicted in comparison to some well known baselines which are evaluated on an autonomous system with widely used performance metrics.
更多查看译文
关键词
Stochastic systems,Expectation maximization,Guided policy search,Reinforcement learning,Maximum likelihood,Trajectory optimization
AI 理解论文
溯源树
样例
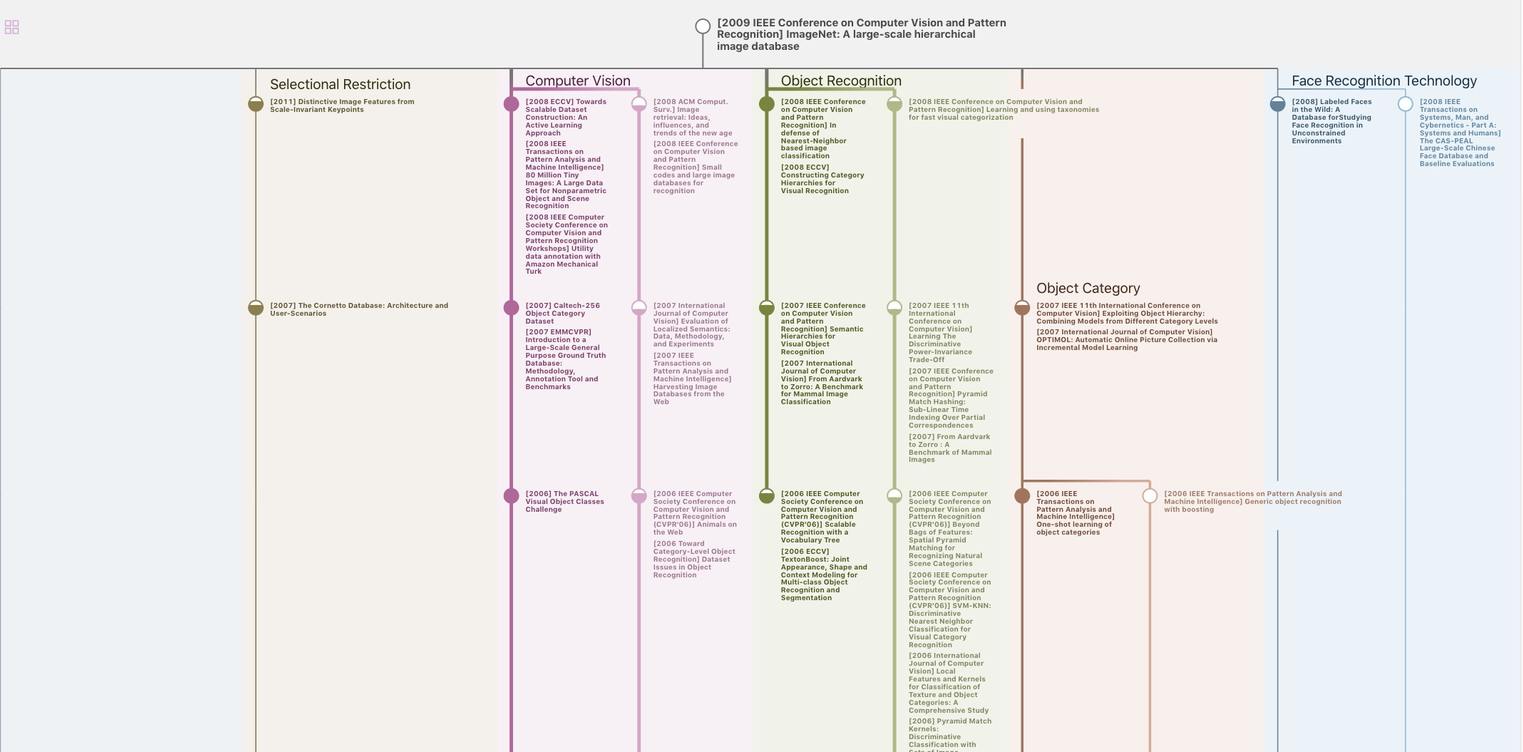
生成溯源树,研究论文发展脉络
Chat Paper
正在生成论文摘要