Solving Zero-Sum Games through Alternating Projections
arxiv(2021)
摘要
In this work, we establish near-linear and strong convergence for a natural first-order iterative algorithm that simulates Von Neumann's Alternating Projections method in zero-sum games. First, we provide a precise analysis of Optimistic Gradient Descent/Ascent (OGDA) -- an optimistic variant of Gradient Descent/Ascent \cite{DBLP:journals/corr/abs-1711-00141} -- for \emph{unconstrained} bilinear games, extending and strengthening prior results along several directions. Our characterization is based on a closed-form solution we derive for the dynamics, while our results also reveal several surprising properties. Indeed, our main algorithmic contribution is founded on a geometric feature of OGDA we discovered; namely, the limit points of the dynamics are the orthogonal projection of the initial state to the space of attractors. Motivated by this property, we show that the equilibria for a natural class of \emph{constrained} bilinear games are the intersection of the unconstrained stationary points with the corresponding probability simplexes. Thus, we employ OGDA to implement an Alternating Projections procedure, converging to an $\epsilon$-approximate Nash equilibrium in $\widetilde{\mathcal{O}}(\log^2(1/\epsilon))$ iterations. Although our algorithm closely resembles the no-regret projected OGDA dynamics, it surpasses the optimal no-regret convergence rate of $\Theta(1/\epsilon)$ \cite{DASKALAKIS2015327}, while it also supplements the recent work in pursuing last-iterate guarantees in saddle-point problems \cite{daskalakis2018lastiterate,mertikopoulos2018optimistic}. Finally, we illustrate an -- in principle -- trivial reduction from any game to the assumed class of instances, without altering the space of equilibria.
更多查看译文
AI 理解论文
溯源树
样例
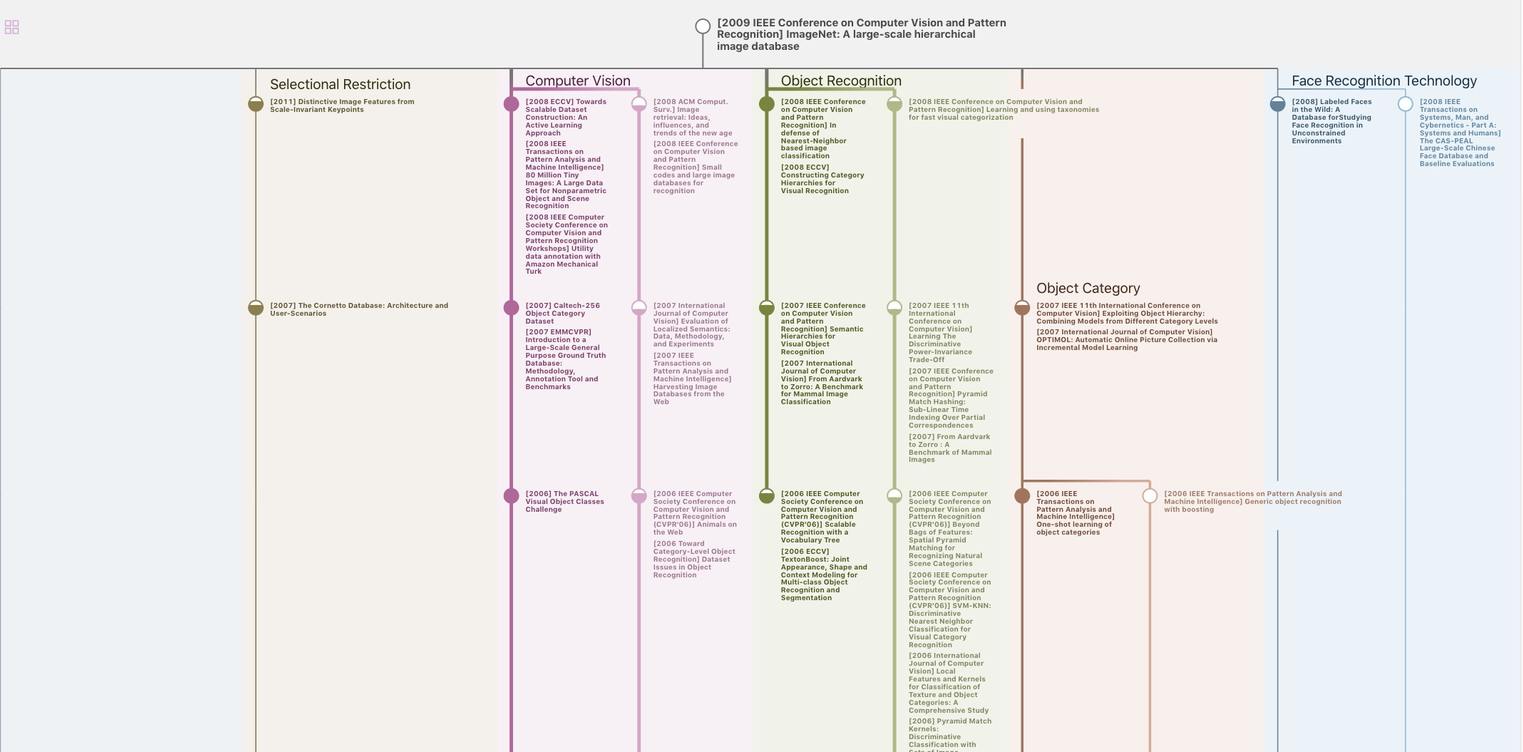
生成溯源树,研究论文发展脉络
Chat Paper
正在生成论文摘要