A General Bayesian Model For Heteroskedastic Data With Fully Conjugate Full-Conditional Distributions
JOURNAL OF STATISTICAL COMPUTATION AND SIMULATION(2021)
Abstract
Models for heteroskedastic data are relevant in a variety of applications ranging from financial time series to environmental statistics. However, the topic of modelling the variance function conditionally has not seen as much attention as modelling the mean. Volatility models have been used in specific applications, but these models can be difficult to fit in a Bayesian setting due to posterior distributions that are challenging to sample efficiently. In this work, we introduce a general model for heteroskedastic data. This approach models the conditional variance as a function of any desired covariates or random effects. We rely on multivariate log-Gamma distribution theory to construct priors that yield fully conjugate full-conditional distributions for Gibbs sampling. Furthermore, we extend the model to a deep learning approach that can provide highly accurate estimates for time dependent data. We also provide an extension for heavy-tailed data. We illustrate our methodology via three applications.
MoreTranslated text
Key words
Deep learning, echo state network, Gibbs sampling, mixed models, multivariate log-Gamma, spatial, volatility
AI Read Science
Must-Reading Tree
Example
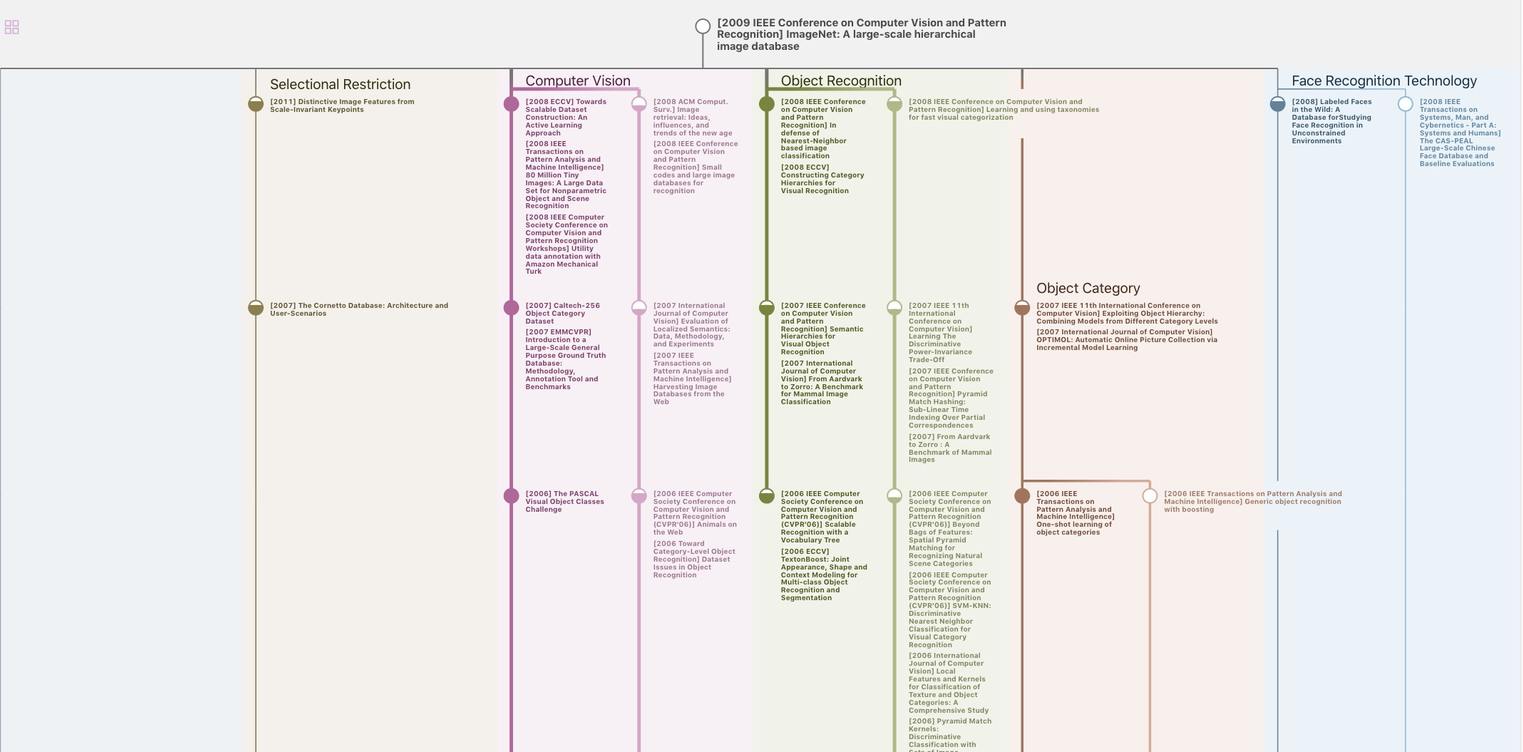
Generate MRT to find the research sequence of this paper
Chat Paper
Summary is being generated by the instructions you defined