Reinforcement Learning Based Congestion Control in a Real Environment
2020 29th International Conference on Computer Communications and Networks (ICCCN)(2020)
摘要
Congestion control plays an important role in the Internet to handle real-world network traffic. It has been dominated by hand-crafted heuristics for decades. Recently, reinforcement learning shows great potentials to automatically learn optimal or near-optimal control policies to enhance the performance of congestion control. However, existing solutions train agents in either simulators or emulators, which cannot fully reflect the real-world environment and degrade the performance of network communication. In order to eliminate the performance degradation caused by training in the simulated environment, we first highlight the necessity and challenges to train a learningbased agent in real-world networks. Then we propose a framework, ARC, for learning congestion control policies in a real environment based on asynchronous execution and demonstrate its effectiveness in accelerating the training. We evaluate our scheme on the real testbed and compare it with state-of-the-art congestion control schemes. Experimental results demonstrate that our schemes can achieve higher throughput and lower latency in comparison with existing schemes.
更多查看译文
关键词
Reinforcement learning,network system,congestion control
AI 理解论文
溯源树
样例
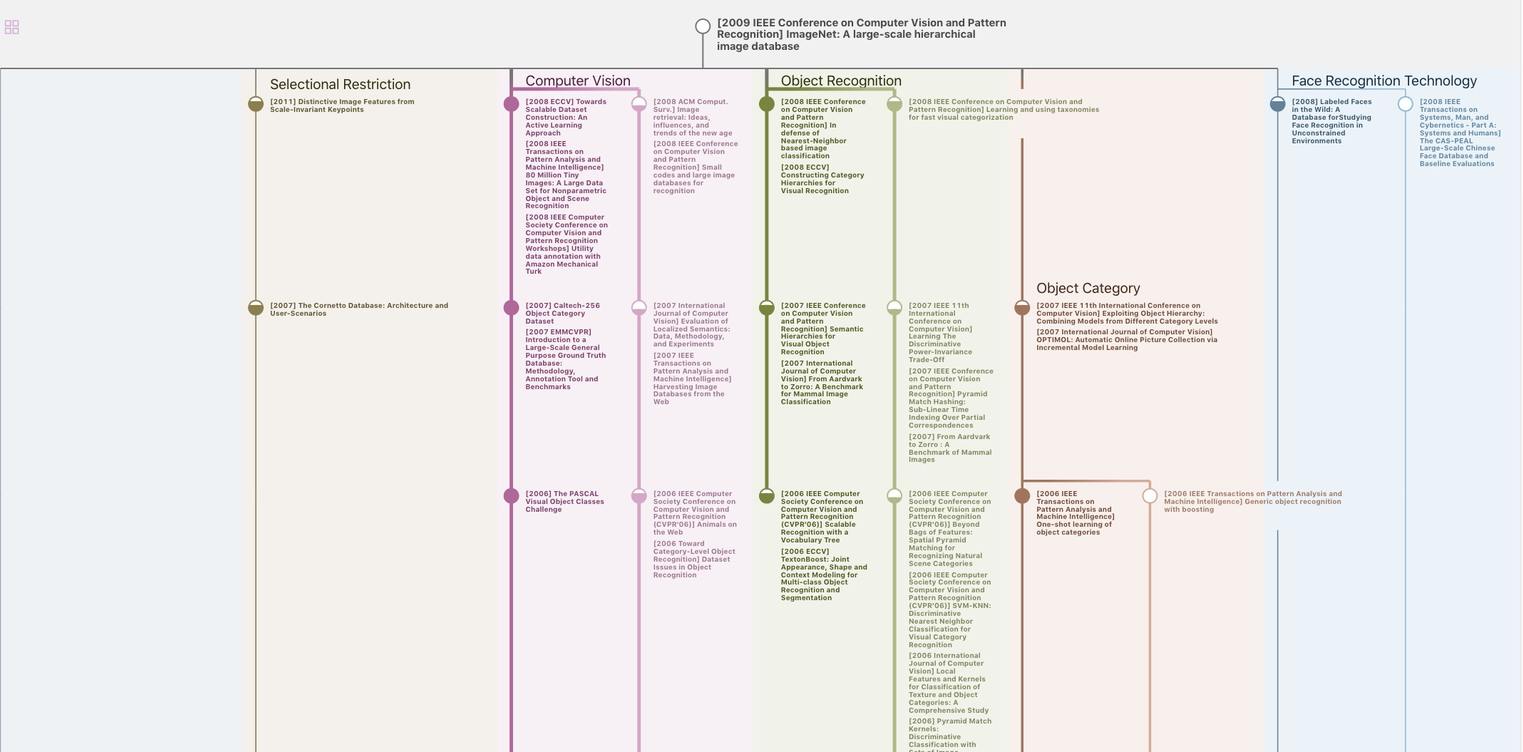
生成溯源树,研究论文发展脉络
Chat Paper
正在生成论文摘要