Accelerating T(2)mapping of the brain by integrating deep learning priors with low-rank and sparse modeling
MAGNETIC RESONANCE IN MEDICINE(2021)
摘要
Purpose To accelerate T(2)mapping with highly sparse sampling by integrating deep learning image priors with low-rank and sparse modeling. Methods The proposed method achieves high-speed T(2)mapping by highly sparsely sampling (k, TE)-space. Image reconstruction from the undersampled data was done by exploiting the low-rank structure and sparsity in the T-2-weighted image sequence and image priors learned from training data. The image priors for a single TE were generated from the public Human Connectome Project data using a tissue-based deep learning method; the image priors were then transferred to other TEs using a generalized series-based method. With these image priors, the proposed reconstruction method used a low-rank model and a sparse model to capture subject-dependent novel features. Results The proposed method was evaluated using experimental data obtained from both healthy subjects and tumor patients using a turbo spin-echo sequence. High-quality T(2)maps at the resolution of 0.9 x 0.9 x 3.0 mm(3)were obtained successfully from highly undersampled data with an acceleration factor of 8. Compared with the existing compressed sensing-based methods, the proposed method produced significantly reduced reconstruction errors. Compared with the deep learning-based methods, the proposed method recovered novel features better. Conclusion This paper demonstrates the feasibility of learning T-2-weighted image priors for multiple TEs using tissue-based deep learning and generalized series-based learning. A new method was proposed to effectively integrate these image priors with low-rank and sparse modeling to reconstruct high-quality images from highly undersampled data. The proposed method will supplement other acquisition-based methods to achieve high-speed T(2)mapping.
更多查看译文
关键词
deep learning, low-rank modeling, quantitative imaging, sparse modeling, T(2)mapping
AI 理解论文
溯源树
样例
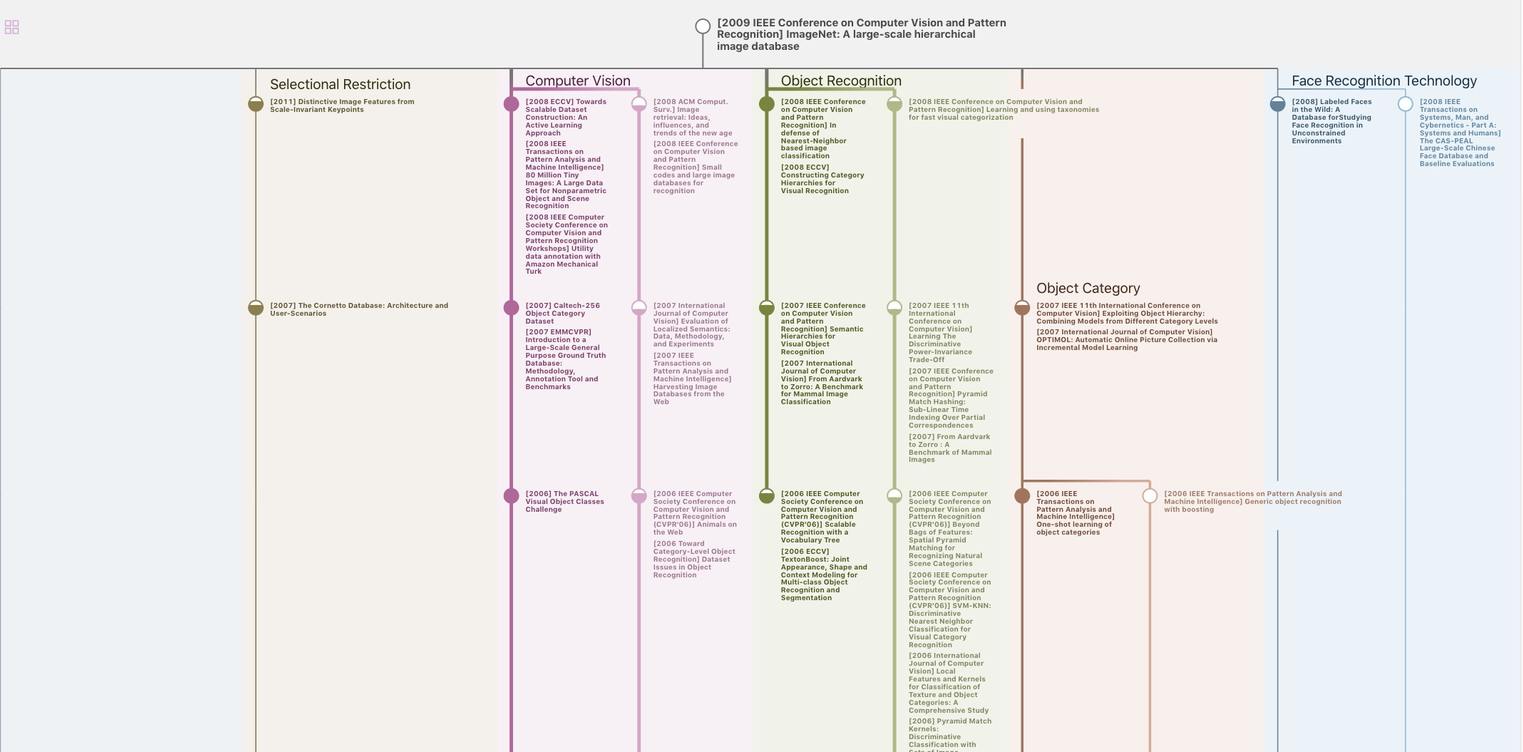
生成溯源树,研究论文发展脉络
Chat Paper
正在生成论文摘要