Leveraging Multisource Information in Matrix Factorization for Social Collaborative Filtering
2020 International Joint Conference on Neural Networks (IJCNN)(2020)
摘要
Collaborative Filtering is a technique that automatically predicts the interest of an active user by collecting rating information from other similar users or items. However, existing works either leave out the user reliability or cannot capture the implicit correlation between two users who are similar but not socially connected. Besides, they also take the trust value between users is either 0 or 1, thus will degenerate the prediction accuracy. In this work, we propose a social collaborative filtering algorithm via leveraging multisource information in matrix factorization, which aims to solve the above problems via employing both rating records and users’ social network information. To this end, we design a novel recommendation framework, which consists of two fundamental components: computation of user reliability and implicit interaction, designing of user-shared feature space. In particular, the latter performs matrix factorization based on user reliability. Finally, a reasonable objective function is designed to synthetically consider the multisource information of users, such that high-quality recommendation can be produced. Experimental results demonstrate that the proposed model achieves better accuracy than other counterpart recommendation techniques.
更多查看译文
关键词
Reliability,Correlation,Social network services,Collaboration,Recommender systems,Matrix decomposition
AI 理解论文
溯源树
样例
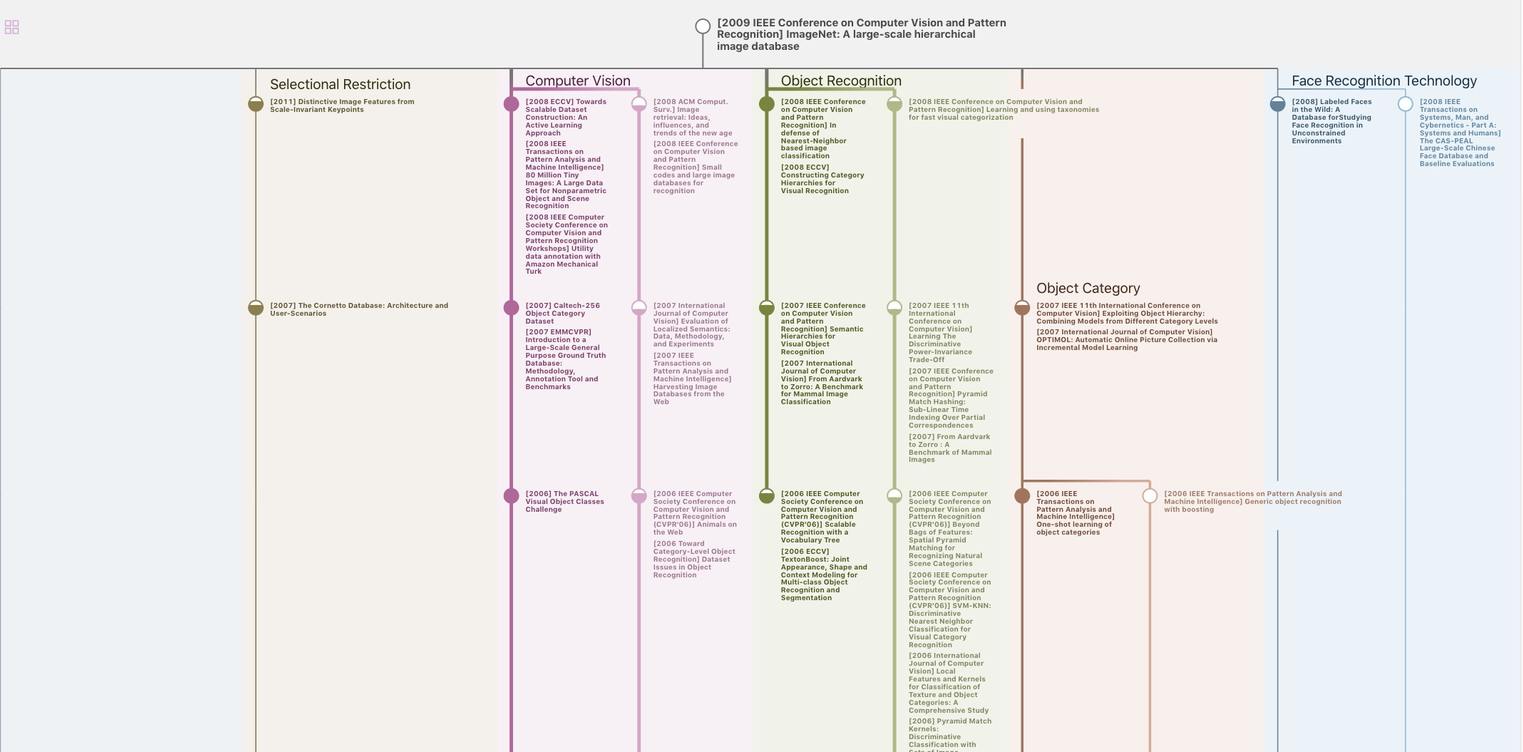
生成溯源树,研究论文发展脉络
Chat Paper
正在生成论文摘要