KPLS–KSER based approach for quality-related monitoring of nonlinear process
ISA Transactions(2021)
摘要
As a commonly used data modeling method, kernel principal least square (KPLS) has been widely used in a variety of process monitoring tasks like soft sensing and fault detection. However, it is not easy to implement fault diagnosis based on KPLS model because the nonlinear mapping of kernel methodology completely obscures the correspondence between the original variable sample and the kernel model. Although the kernel-gradient based algorithms have successfully extracted the analytical solution of contribution plot, they are still very inefficient and impractical because of their high amount of computation. In order to overcome this inherent defect, a new idea of kernel sample equivalent replacement (KSER) will be used in this paper, which reveals an important relationship between centralized kernel matrix and the variance–covariance matrix of centralized variable matrix. By integrating KSER into KPLS, a linear regression between the original variable sample and the output could be directly constructed. This approach makes it possible to use the linear algorithm to diagnose the faulty variables. Based on which, an improved contribution plot algorithm is further integrated to the new model to locate faulty variables. Thereby, the complex nonlinear diagnosis problem can be finally solved by a linear manner with extremely low computational complexity. Simulation results of both numerical and industrial examples demonstrate the effectiveness of the proposed method.
更多查看译文
关键词
Data-driven,Quality-related,Fault diagnosis,Kernel partial least squares,Kernel sample equivalent replacement,Improved contribution plot,Nonlinear process
AI 理解论文
溯源树
样例
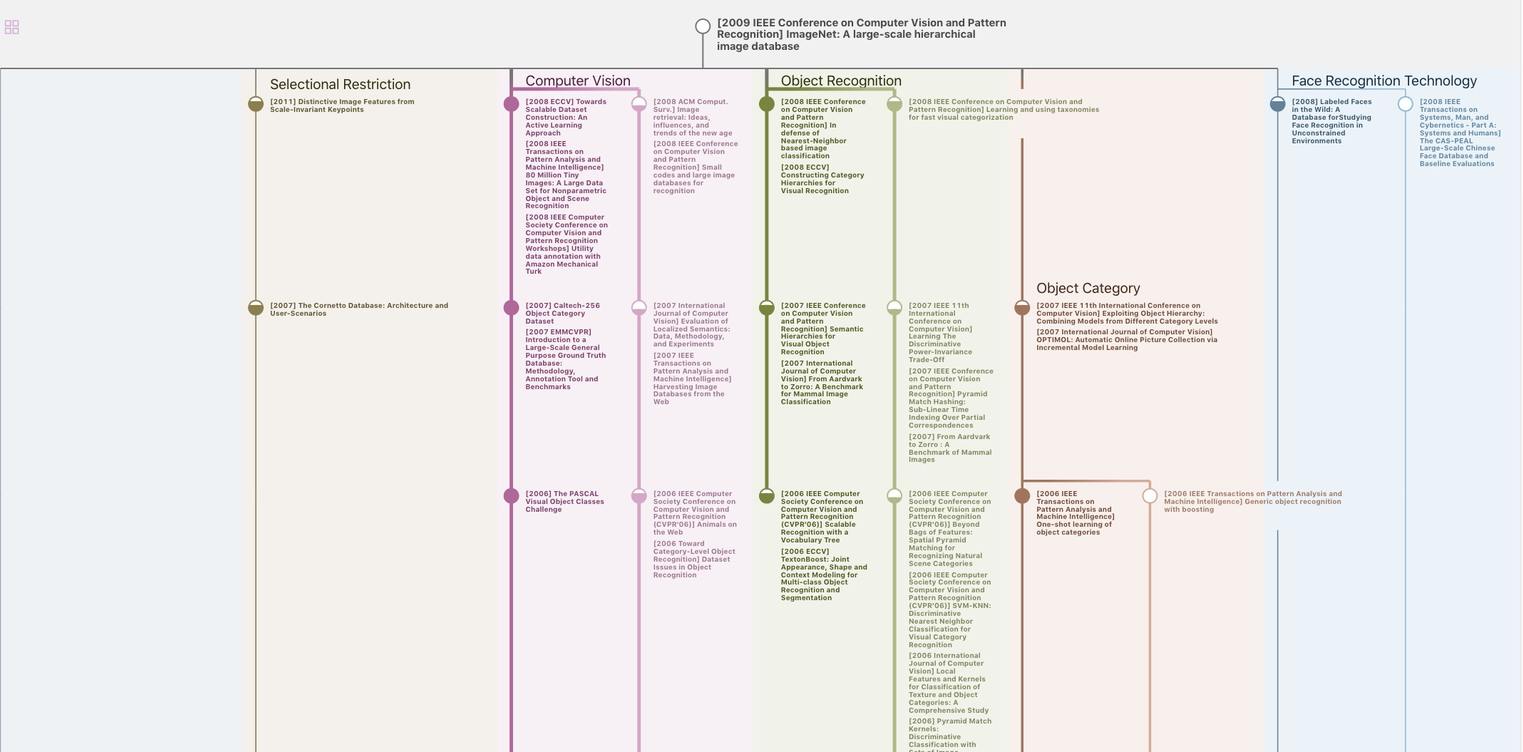
生成溯源树,研究论文发展脉络
Chat Paper
正在生成论文摘要