Surrogate-agent modeling for improved training
Engineering Applications of Artificial Intelligence(2018)
摘要
Extensive skill training using simulations for improving decision making has become a common practice in a multitude of domains and professions due to the expense or danger of training in real-life circumstances, or to speed up the learning process. In this work we propose a novel approach called PATDS (Performance Analysis of Training through Data-Farming Simulations) in which a simulated agent as a virtual copy of a human trainee is constructed and tested in all circumstances to provide feedback and decision support for another round of human learning. PATDS includes the analysis of behavior demonstrated by the trainee in simulations and the identification of relevant behavior patterns; that is, strategies. The identified patterns are in turn used to model a surrogate agent of the trainee. The created agent is then evaluated with data-farming experiments on a variety of simulation parameters to estimate the key weaknesses in its behavior and the overall performance. In subsequent rounds of training, the trainee improves the behavior based on the identified weaknesses and strategic decision recommendations; a new surrogate agent is designed adding new skills from the trainee until the desired overall performance is achieved. We evaluated the proposed approach on two asymmetric urban-terrain threat scenarios where a squad of security forces interacts with hostile crowds. We demonstrate that the data-farming-based evaluation in the complete simulation parameter space is beneficial for improving the trainees’ capabilities.
更多查看译文
关键词
Multi-agent systems,Agent-based simulation training,Decision making,Behavior analysis,Data farming
AI 理解论文
溯源树
样例
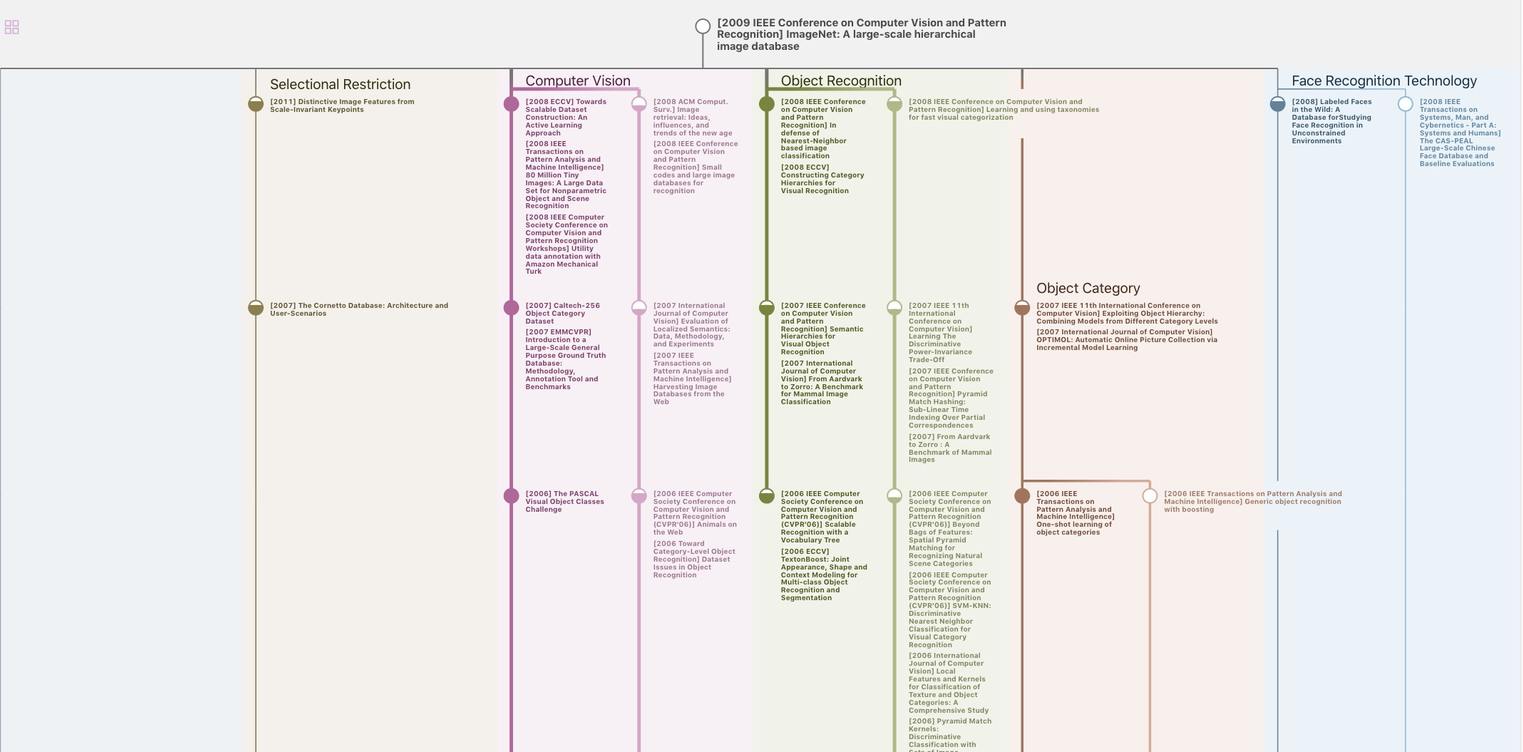
生成溯源树,研究论文发展脉络
Chat Paper
正在生成论文摘要