Deep Learning Based Covert Attack Identification for Industrial Control Systems
2020 19th IEEE International Conference on Machine Learning and Applications (ICMLA)(2020)
摘要
Cybersecurity of Industrial Control Systems (ICS) is drawing significant concerns as data communication increasingly leverages wireless networks. A lot of data-driven methods were developed for detecting cyberattacks, but few are focused on distinguishing them from equipment faults. In this paper, we develop a data-driven framework that can be used to detect, diagnose, and localize a type of cyberattack called covert attacks on smart grids. The framework has a hybrid design that combines an autoencoder, a recurrent neural network (RNN) with a Long-Short-Term-Memory (LSTM) layer, and a Deep Neural Network (DNN). This data-driven framework considers the temporal behavior of a generic physical system that extracts features from the time series of the sensor measurements that can be used for detecting covert attacks, distinguishing them from equipment faults, as well as localize the attack/fault. We evaluate the performance of the proposed method through a realistic simulation study on the IEEE 14-bus model as a typical example of ICS. We compare the performance of the proposed method with the traditional model-based method to show its applicability and efficacy.
更多查看译文
关键词
cybersecurity,industrial control system,smart grid,LSTM
AI 理解论文
溯源树
样例
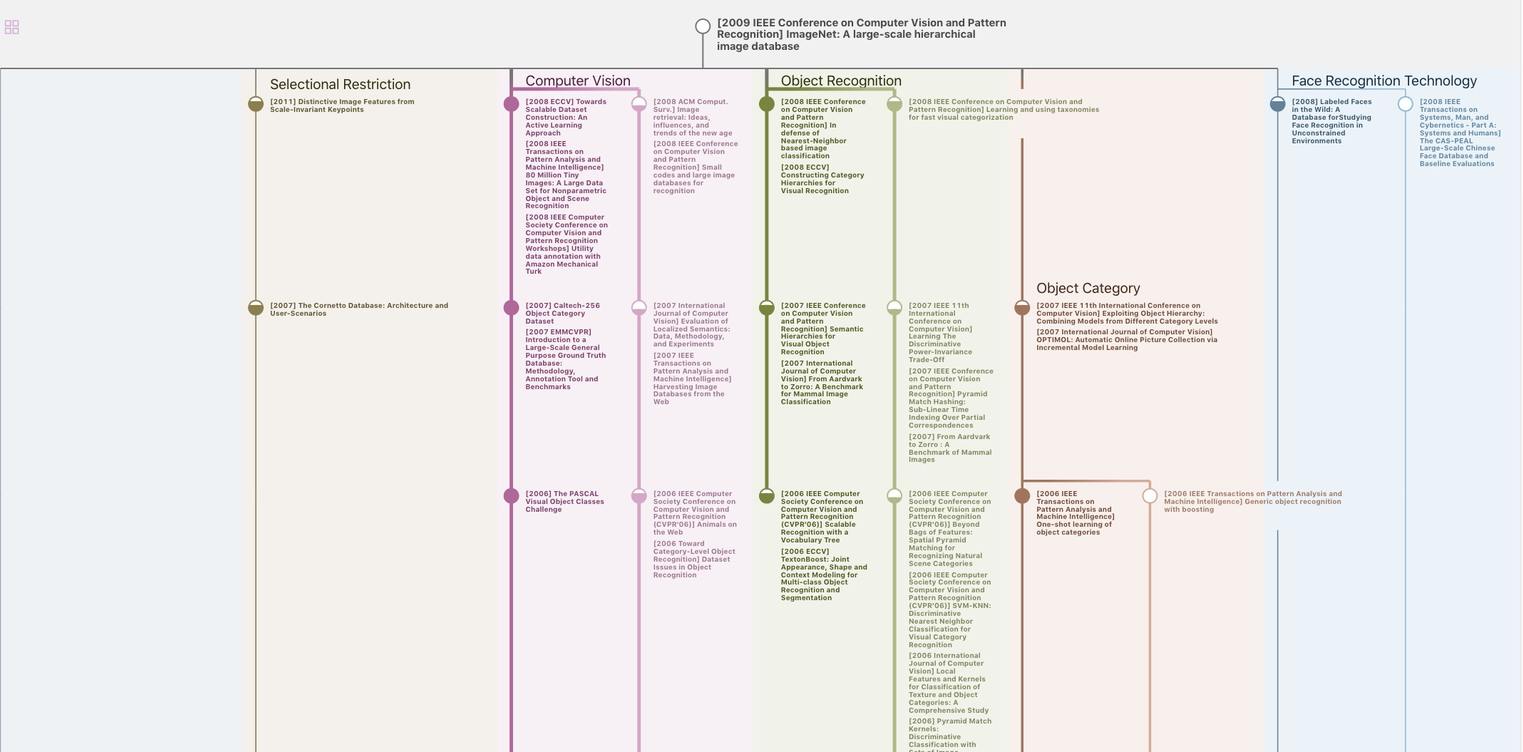
生成溯源树,研究论文发展脉络
Chat Paper
正在生成论文摘要