Prognostic biomarkers related to breast cancer recurrence identified based on Logit model analysis
WORLD JOURNAL OF SURGICAL ONCOLOGY(2020)
摘要
Background This study intended to determine important genes related to the prognosis and recurrence of breast cancer. Methods Gene expression data of breast cancer patients were downloaded from TCGA database. Breast cancer samples with recurrence and death were defined as poor disease-free survival (DFS) group, while samples without recurrence and survival beyond 5 years were defined as better DFS group. Another gene expression profile dataset (GSE45725) of breast cancer was downloaded as the validation data. Differentially expressed genes (DEGs) were screened between better and poor DFS groups, which were then performed function enrichment analysis. The DEGs that were enriched in the GO function and KEGG signaling pathway were selected for cox regression analysis and Logit regression (LR) model analysis. Finally, correlation analysis between LR model classification and survival prognosis was analyzed. Results Based on the breast cancer gene expression profile data in TCGA, 540 DEGs were screened between better DFS and poor DFS groups, including 177 downregulated and 363 upregulated DEGs. A total of 283 DEGs were involved in all GO functions and KEGG signaling pathways. Through LR model screening, 10 important feature DEGs were identified and validated, among which, ABCA3 , CCL22 , FOXJ1 , IL1RN , KCNIP3 , MAP2K6 , and MRPL13 , were significantly expressed in both groups in the two data sets. ABCA3 , CCL22 , FOXJ1 , IL1RN , and MAP2K6 were good prognostic factors, while KCNIP3 and MRPL13 were poor prognostic factors. Conclusion ABCA3 , CCL22 , FOXJ1 , IL1RN , and MAP2K6 may serve as good prognostic factors, while KCNIP3 and MRPL13 may be poor prognostic factors for the prognosis of breast cancer.
更多查看译文
关键词
Breast cancer,Prognosis,Recurrence,Logit regression model
AI 理解论文
溯源树
样例
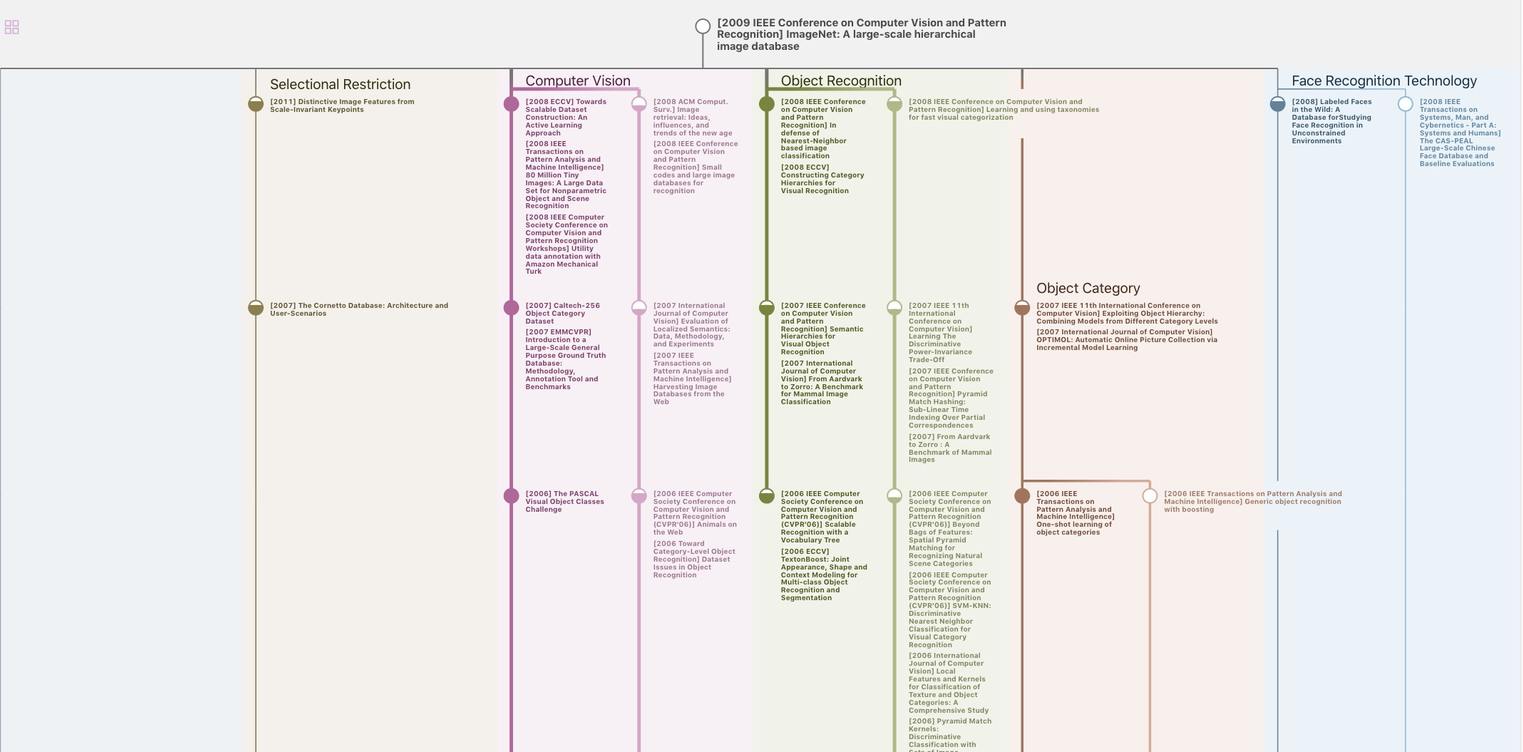
生成溯源树,研究论文发展脉络
Chat Paper
正在生成论文摘要