Machine Learning in Optical Communication Systems and Networks
2020 IEEE Workshop on Signal Processing Systems (SiPS)(2020)
摘要
Machine learning (ML) is finding efficient application in innumerous fields of our modern society. The range of applications spans from the creation of new perfumes to the development of automatic weapons and almost everything in between [1]. Given the huge success of ML in all those fields it was completely natural that the optical communications community tried to take advantage of such efficient data-driven approximators. In this talk we start by addressing the question of when ML can be useful in optical systems and networks namely: • for which type of problems should we rely on probabilistic ML algorithms rather than on traditional deterministic approaches? • when is ML cost-effective against existing / alternatives approaches? Afterwards, the major decisions that need to be taken during the training phase of a ML algorithm are briefly overview, and other practical aspects such as the importance of monitoring the ML algorithm during its life cycle or the challenges faced when the algorithm goes towards a production environment are also introduced [1]. As far as possible, the concepts will be illustrated using realistic use-cases [2–6]. Two different use-cases will be mainly used: (1) Quality of Transmission (QoT) estimation in open / disaggregated systems (ii) Raman amplifier inverse system design QoT estimation in open/disaggregated networks is a big challenge as a compromise between accuracy, computational-time, and shared information needs to be achieved. The usually proprietary heuristic models used (i.e. derived by the system vendor), can't be immediately applied to estimate the performance of lightpaths traversing equipment from different vendors. This is being addressed by industry consortiums promoting common models to be used in such scenarios, however at the cost of accuracy. We present a framework composed by low and high precision estimators and a calibration engine based on Deep Neural Networks (DNN), allowing to control open vs. proprietary implementations. In this way, supported in a first estimation given by the open implementation, the DNN can enhance the performance of the lightpath QoT estimation up to a level close to the one achieved with the proprietary implementation. The second use-case that will be addressed is the Raman amplifier inverse system design which consists of selecting pump powers and wavelengths that would result in a targeted gain profile [6]. This is a challenging task due to complex interaction between pumps and Raman gain making the irreversible problem very demanding. Conventionally requires trial and error with some human guidance. Moreover, in open scenarios different vendors select different Raman pumps both in terms of power and wavelength. This complex problem will be tackled using two different ML frameworks: Autoencoders and Generative Adversarial Networks (GANs).
更多查看译文
关键词
Machine learning,Optical fiber networks,Estimation,Stimulated emission,Optical fiber amplifiers,System analysis and design
AI 理解论文
溯源树
样例
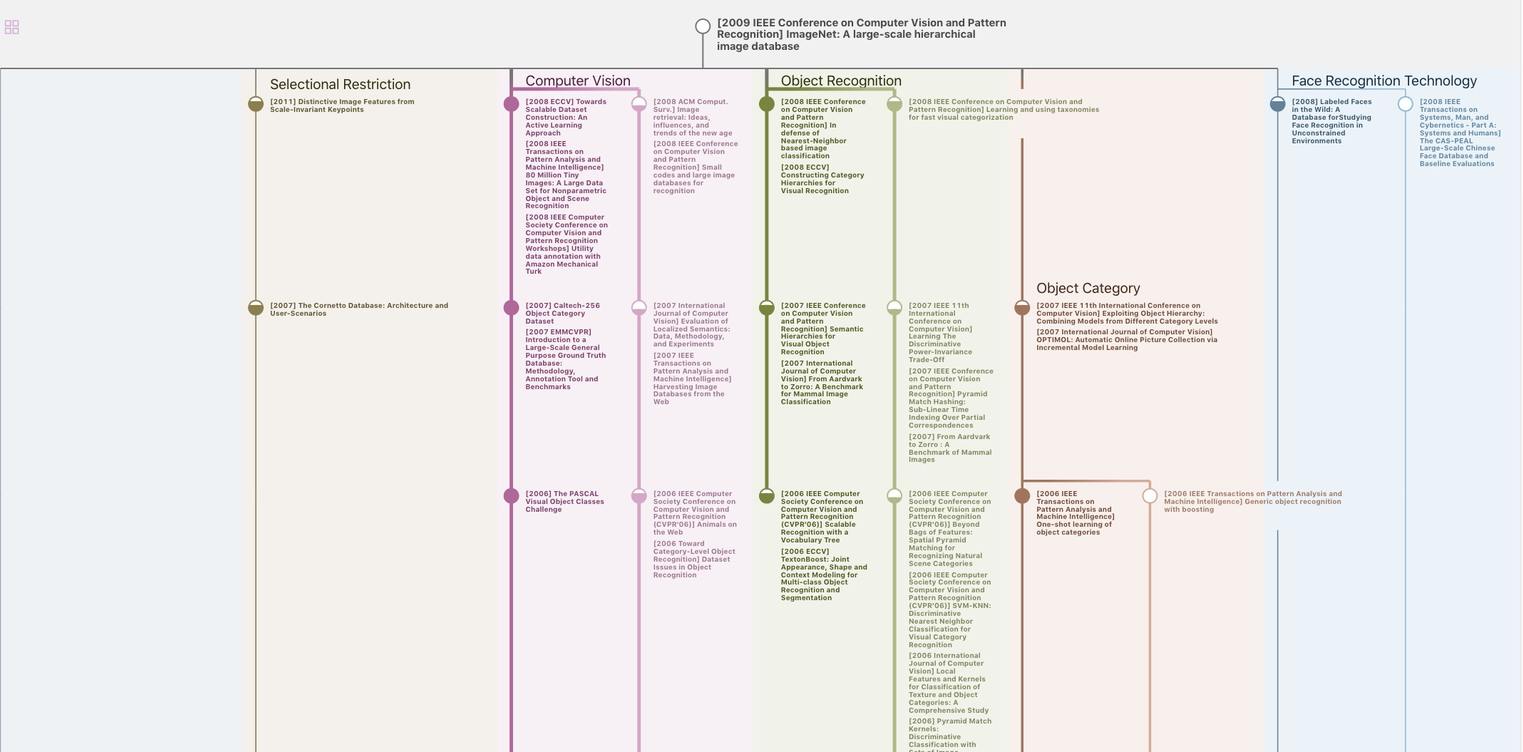
生成溯源树,研究论文发展脉络
Chat Paper
正在生成论文摘要