Hierarchical Bayesian bootstrap for heterogeneous treatment effect estimation.
The international journal of biostatistics(2022)
摘要
A major focus of causal inference is the estimation of heterogeneous average treatment effects (HTE) - average treatment effects within strata of another variable of interest such as levels of a biomarker, education, or age strata. Inference involves estimating a stratum-specific regression and integrating it over the distribution of confounders in that stratum - which itself must be estimated. Standard practice involves estimating these stratum-specific confounder distributions independently (e.g. via the empirical distribution or Rubin's Bayesian bootstrap), which becomes problematic for sparsely populated strata with few observed confounder vectors. In this paper, we develop a nonparametric hierarchical Bayesian bootstrap (HBB) prior over the stratum-specific confounder distributions for HTE estimation. The HBB partially pools the stratum-specific distributions, thereby allowing principled borrowing of confounder information across strata when sparsity is a concern. We show that posterior inference under the HBB can yield efficiency gains over standard marginalization approaches while avoiding strong parametric assumptions about the confounder distribution. We use our approach to estimate the adverse event risk of proton versus photon chemoradiotherapy across various cancer types.
更多查看译文
关键词
Bayesian nonparametrics,Dirichlet process,bootstrap,causal inference,heterogenous treatment effects
AI 理解论文
溯源树
样例
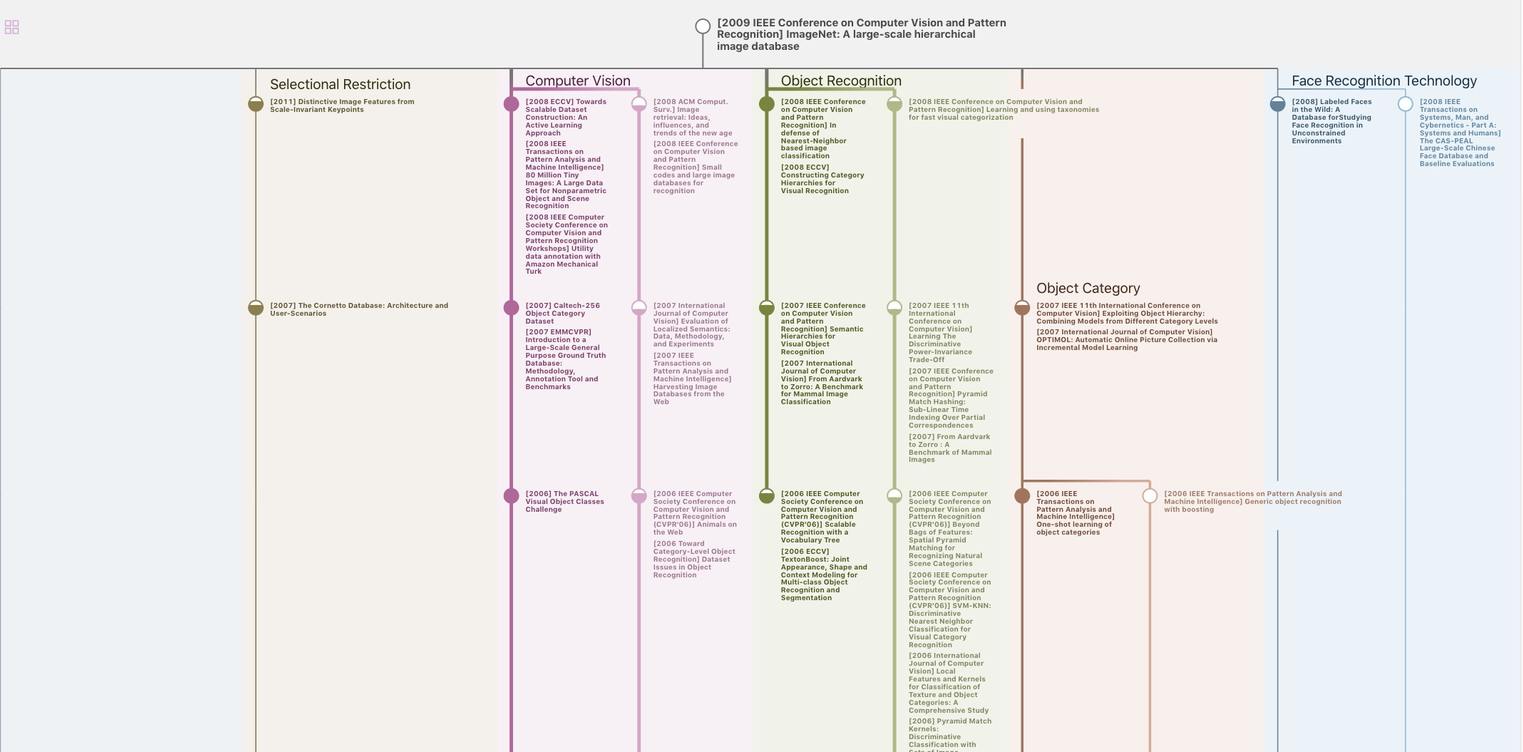
生成溯源树,研究论文发展脉络
Chat Paper
正在生成论文摘要