Machine Learning Identifies Novel Candidates for Drug Repurposing in Alzheimer’s Disease
biorxiv(2020)
摘要
Clinical trials of novel therapeutics for Alzheimer’s Disease (AD) have consumed a large amount of time and resources with largely negative results. Repurposing drugs already approved by the Food and Drug Administration (FDA) for another indication is a more rapid and less expensive option. Repurposing can yield a useful therapeutic and also accelerate proof of concept studies that ultimately lead to a new molecular entity. We present a novel machine learning framework, DRIAD (rug epurposing In ), that quantifies potential associations between the pathology of AD severity (the Braak stage) and molecular mechanisms as encoded in lists of gene names. DRIAD was validated on gene lists known to be associated with AD from other studies and subsequently applied to evaluate lists of genes arising from perturbations in differentiated human neural cell cultures by 80 FDA-approved and clinically tested drugs, producing a ranked list of possible repurposing candidates. Top-scoring drugs were inspected for common trends among their nominal molecular targets and their “off-targets”, revealing a high prevalence of kinases from the Janus (JAK), Unc-51-like (ULK) and NIMA-related (NEK) families. These kinase families are known to modulate pathways related to innate immune signaling, autophagy, and microtubule formation and function, suggesting possible disease-modifying mechanisms of action. We propose that the DRIAD method can be used to nominate drugs that, after additional validation and identification of relevant pharmacodynamic biomarker(s), could be evaluated in a clinical trial.
更多查看译文
关键词
Alzheimer’,s Disease,drug repurposing,machine learning,kinase
AI 理解论文
溯源树
样例
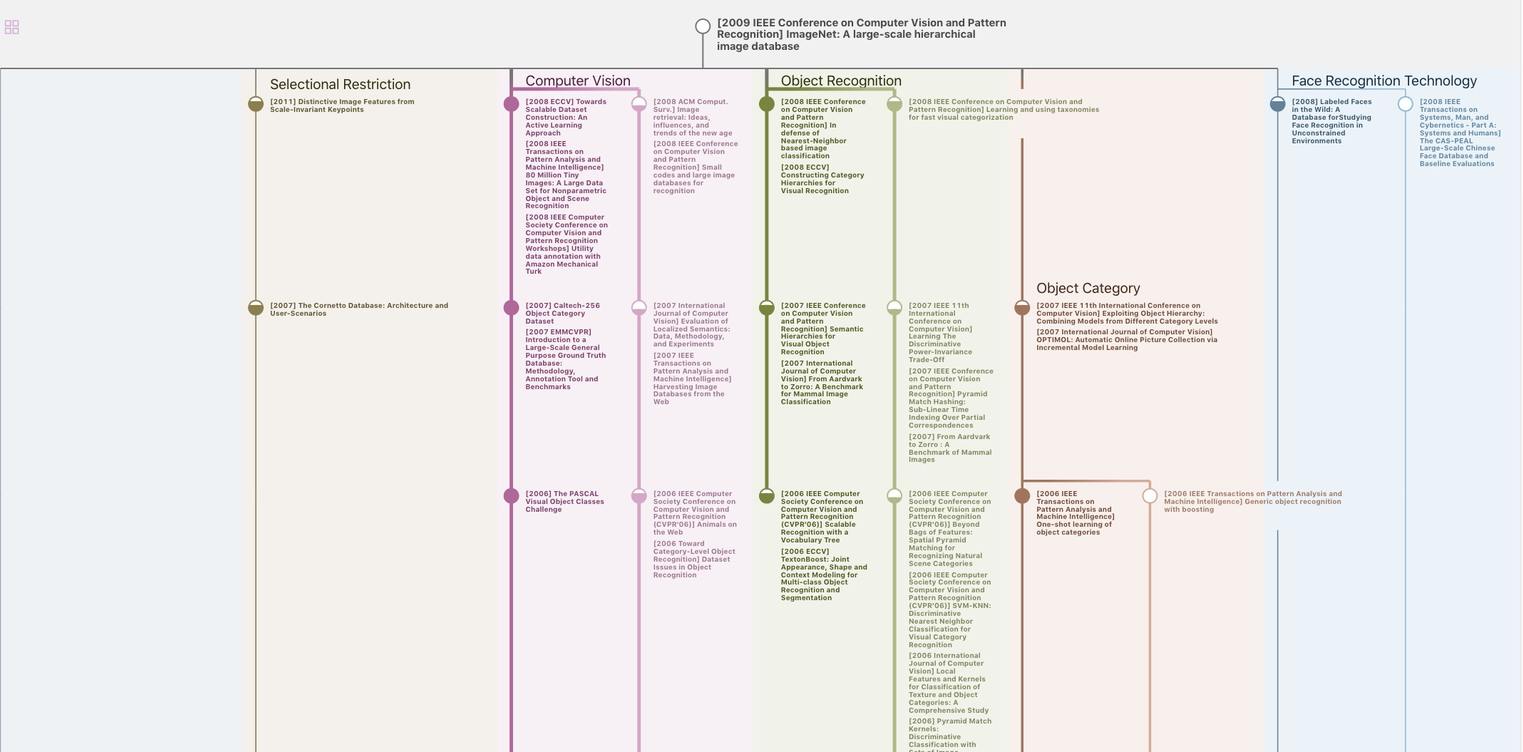
生成溯源树,研究论文发展脉络
Chat Paper
正在生成论文摘要