A Deep Learning Approach for Tissue Spatial Quantification and Genomic Correlations of Histopathological Images
biorxiv(2020)
摘要
Epithelial and stromal tissue are components of the tumor microenvironment and play a major role in tumor initiation and progression. Distinguishing stroma from epithelial tissues is critically important for spatial characterization of the tumor microenvironment. We propose an image analysis pipeline based on a Convolutional Neural Network (CNN) model to classify epithelial and stromal regions in whole-slide images. The CNN model was trained using well-annotated breast cancer tissue microarrays and validated with images from The Cancer Genome Atlas (TCGA) project. Our model achieves a classification accuracy of 91.02%, which outperforms other state-of-the-art methods. Using this model, we generated pixel-level epithelial/stromal tissue maps for 1,000 TCGA breast cancer slide images that are paired with gene expression data. We subsequently estimated the epithelial and stromal ratios and performed correlation analysis to model the relationship between gene expression and tissue ratios. Gene Ontology enrichment analyses of genes that were highly correlated with tissue ratios suggest the same tissue was associated with similar biological processes in different breast cancer subtypes, whereas each subtype had its own idiosyncratic biological processes governing the development of these tissues. Taken all together, our approach can lead to new insights in exploring relationships between image-based phenotypes and their underlying genomic data and biological processes for all types of solid tumors.
更多查看译文
关键词
Whole-slide tissue image,Deep learning,Integrative genomics,Breast cancer
AI 理解论文
溯源树
样例
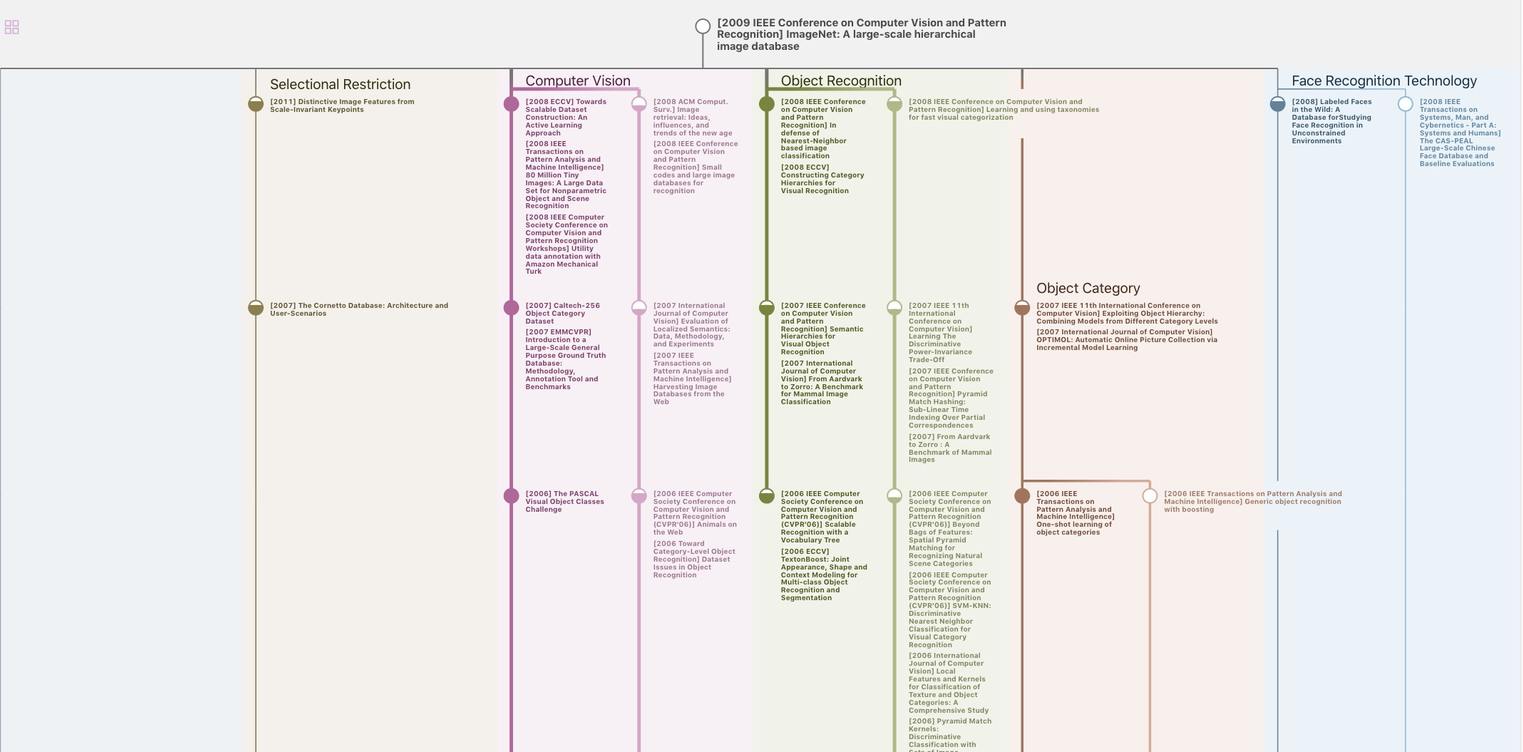
生成溯源树,研究论文发展脉络
Chat Paper
正在生成论文摘要