CASSPER: A Semantic Segmentation based Particle Picking Algorithm for Single Particle Cryo-Electron Microscopy
bioRxiv (Cold Spring Harbor Laboratory)(2020)
Abstract
Single-particle cryo-electron microscopy has emerged as the method of choice for structure determination of proteins and protein complexes. However, particle identification and selection which is a prerequisite for achieving high-resolution still poses a major bottleneck for automating the steps of structure determination. Here, we present a generalised deep learning tool, CASSPER, for the automated detection and isolation of protein particles in transmission microscope images. This deep learning tool uses Semantic Segmentation and a collection of visually prepared training samples to capture the differences in the transmission intensities of protein, ice, carbon and other impurities found in the micrograph. CASSPER is the first method to do pixel level classification and completely eliminates the need of manual particle picking. Integration of Contrast Limited Adaptive Histogram Equalization (CLAHE) in CASSPER enables high-fidelity particle detection even in micrographs with variable ice thickness and contrast. In addition, our generalized model for cross molecule picking works with high efficiency on unseen datasets and can potentially pick particles on-the-fly, thereby, enabling automation of data processing.
MoreTranslated text
Key words
particle picking algorithm,semantic segmentation,cryo-electron
AI Read Science
Must-Reading Tree
Example
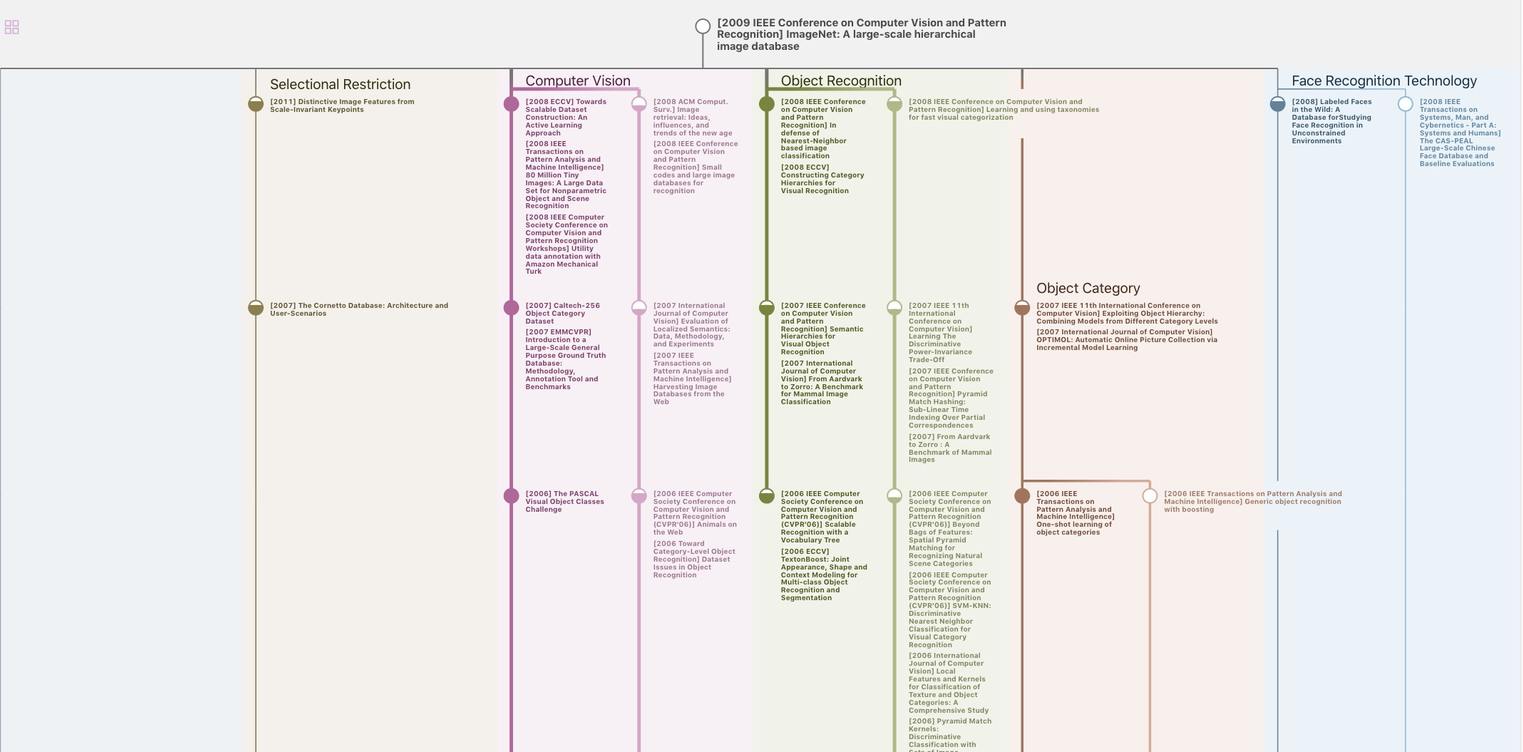
Generate MRT to find the research sequence of this paper
Chat Paper
Summary is being generated by the instructions you defined