A real-time search strategy for finding urban disease vector infestations
biorxiv(2020)
摘要
Containing domestic vector infestation requires the ability to swiftly locate and treat infested homes. In urban settings where vectors are heterogeneously distributed throughout a dense housing matrix, the task of locating infestations can be challenging. Here, we present a novel stochastic compartmental model developed to help locate infested homes in urban areas. We designed the model using infestation data for the Chagas disease vector species in Arequipa, Peru. Our approach incorporates disease vector counts at each observed house, and the vector’s complex spatial dispersal dynamics. We used a Bayesian method to augment the observed data, estimate the insect population growth and dispersal parameters, and determine posterior infestation probabilities of households. We investigated the properties of the model through simulation studies, followed by field testing in Arequipa. Simulation studies showed the model to be accurate in its estimates of two parameters of interest: the growth rate of a domestic triatomine bug colony and the probability of a triatomine bug successfully invading a new home after dispersing from an infested home. When testing the model in the field, data collection using model estimates was hindered by low household participation rates, which severely limited the algorithm and in turn, the model’s predictive power. While future optimization efforts must improve the model’s capabilities when household participation is low, our approach is nonetheless an important step toward integrating data with predictive modeling to carry out evidence-based vector surveillance in cities.
更多查看译文
关键词
urban,search,disease,finding,real-time
AI 理解论文
溯源树
样例
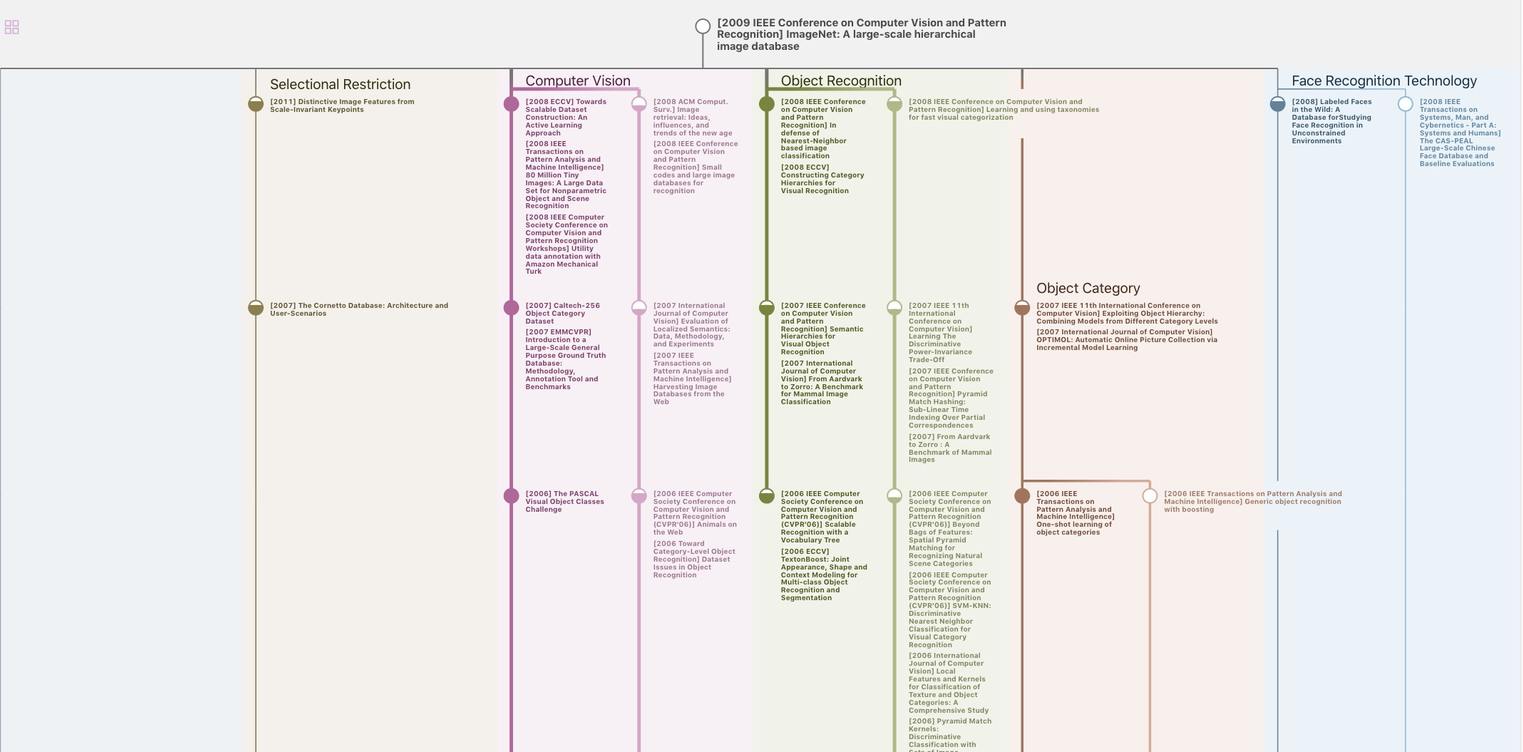
生成溯源树,研究论文发展脉络
Chat Paper
正在生成论文摘要