Identifying genes and cell types with dynamic alternative polyadenylation in multi-cluster single-cell transcriptomics data
biorxiv(2020)
摘要
Alternative polyadenylation (APA) causes shortening or lengthening of the 3’-untranslated region (3’-UTR), widespread in complex tissues. Bioinformatic tools have been developed to identify dynamic APA in single cell RNA-Seq (scRNA-Seq) data, but with relatively low power and the lack of interpretability for multiple cell types. To address these limitations, we developed a model-based method, scMAPA. scMAPA increases power by building a regression model based on a sensitive quantification of 3’-UTR short and long isoforms. By developing a simulation platform, we demonstrated that scMAPA shows a markedly better sensitivity (9.5% on average) than the previous method with a negligible loss in specificity (0.3% on average). scMAPA improves interpretability by modeling the direction and the degree of dynamic APA for each cell type for each gene, while allowing flexibility to control potential confounders. scMAPA enables to bring a systematic understanding of APA dynamics in complex tissues in human Peripheral Blood Monocellular Cells and mouse brain data.
更多查看译文
AI 理解论文
溯源树
样例
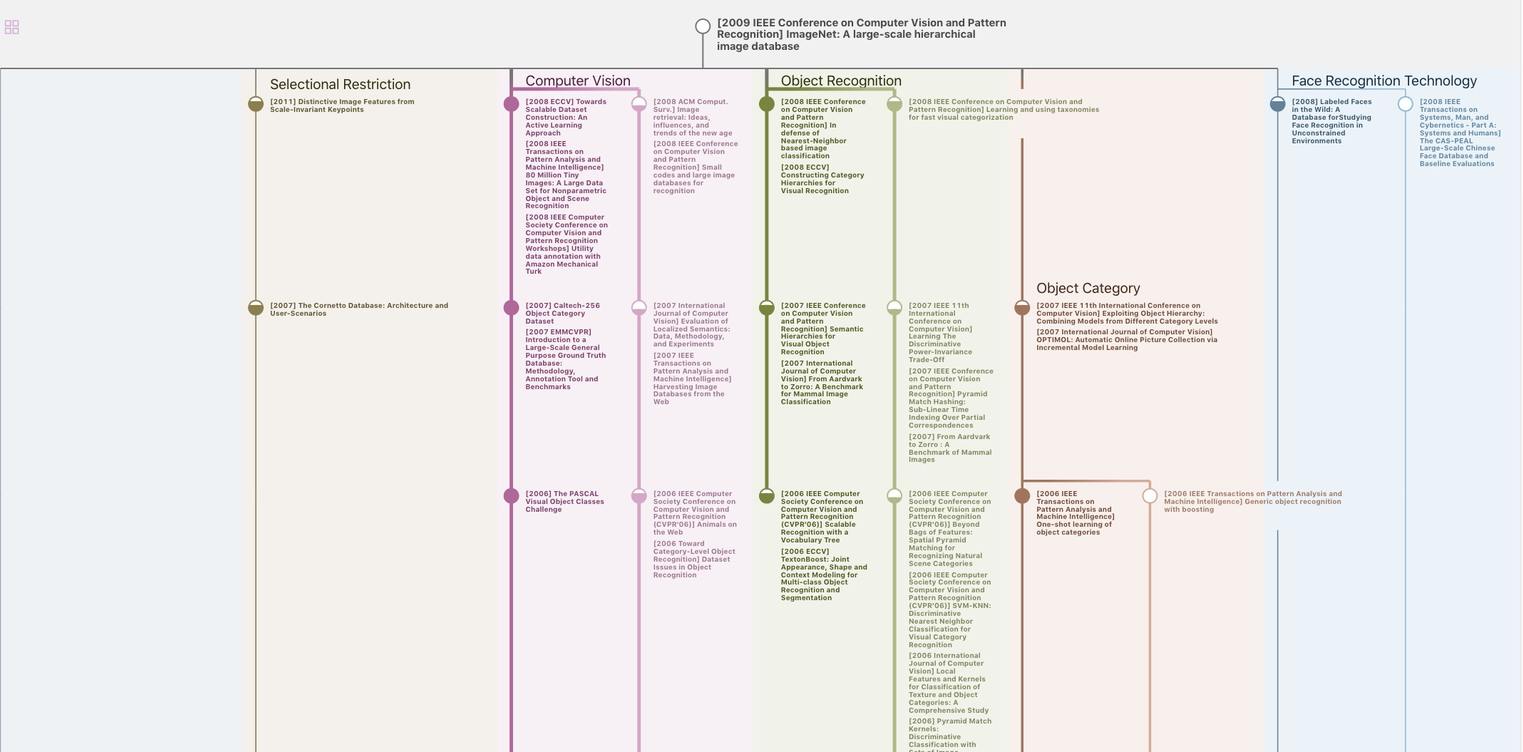
生成溯源树,研究论文发展脉络
Chat Paper
正在生成论文摘要