Multi-Trait Regressor Stacking Increased Genomic Prediction Accuracy Of Sorghum Grain Composition
AGRONOMY-BASEL(2020)
摘要
Genomic prediction has enabled plant breeders to estimate breeding values of unobserved genotypes and environments. The use of genomic prediction will be extremely valuable for compositional traits for which phenotyping is labor-intensive and destructive for most accurate results. We studied the potential of Bayesian multi-output regressor stacking (BMORS) model in improving prediction performance over single trait single environment (STSE) models using a grain sorghum diversity panel (GSDP) and a biparental recombinant inbred lines (RILs) population. A total of five highly correlated grain composition traits-amylose, fat, gross energy, protein and starch, with genomic heritability ranging from 0.24 to 0.59 in the GSDP and 0.69 to 0.83 in the RILs were studied. Average prediction accuracies from the STSE model were within a range of 0.4 to 0.6 for all traits across both populations except amylose (0.25) in the GSDP. Prediction accuracy for BMORS increased by 41% and 32% on average over STSE in the GSDP and RILs, respectively. Prediction of whole environments by training with remaining environments in BMORS resulted in moderate to high prediction accuracy. Our results show regression stacking methods such as BMORS have potential to accurately predict unobserved individuals and environments, and implementation of such models can accelerate genetic gain.
更多查看译文
关键词
genomics, genomic selection, genomic prediction, marker-assisted selection, whole genome regression, grain quality, near infra-red spectroscopy, cereal crop, sorghum, multi-trait
AI 理解论文
溯源树
样例
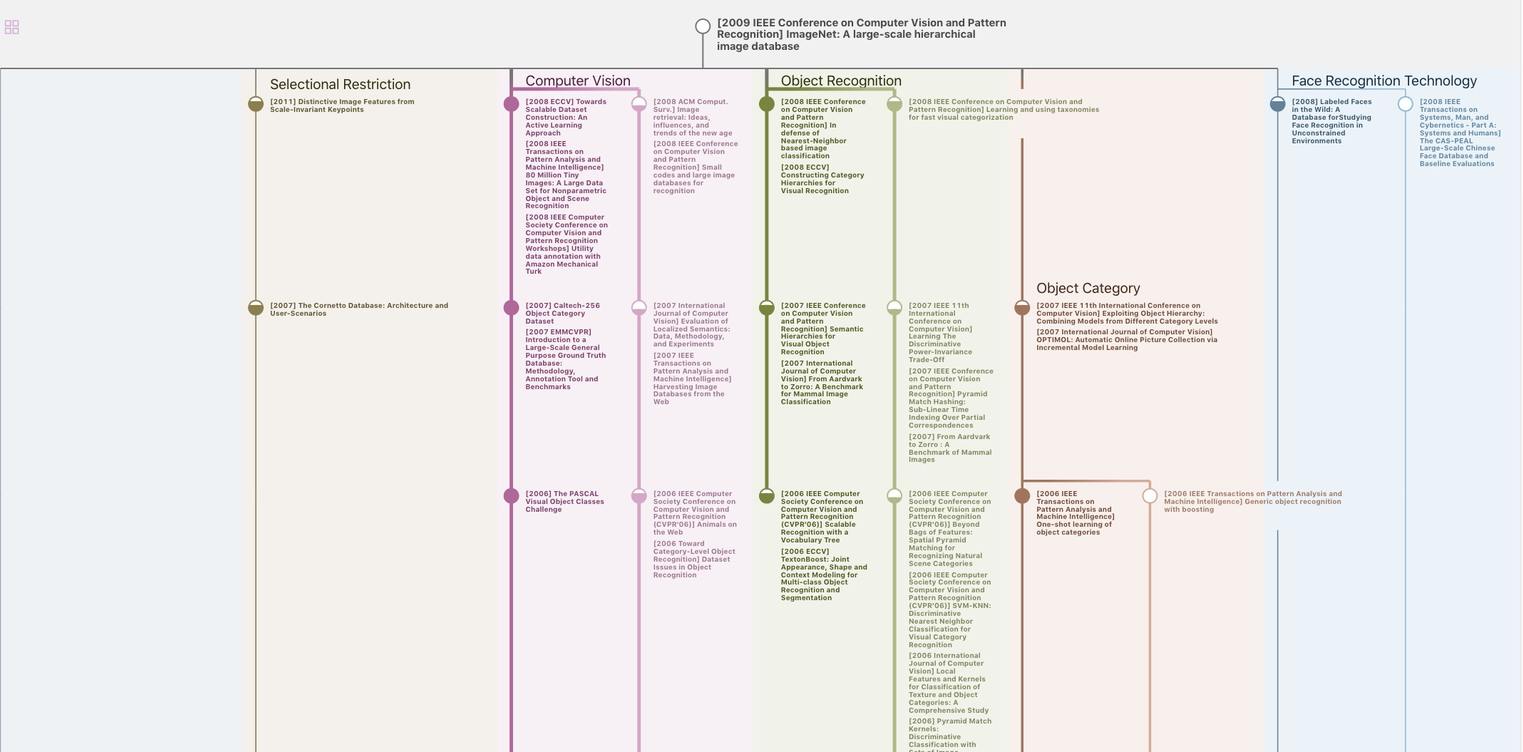
生成溯源树,研究论文发展脉络
Chat Paper
正在生成论文摘要