Provable Finite Data Generalization with Group Autoencoder
arxiv(2020)
摘要
Deep Autoencoders (AEs) provide a versatile framework to learn a compressed, interpretable, or structured representation of data. As such, AEs have been used extensively for denoising, compression, data completion as well as pre-training of Deep Networks (DNs) for various tasks such as classification. By providing a careful analysis of current AEs from a spline perspective, we can interpret the input-output mapping, in turn allowing us to derive conditions for generalization and reconstruction guarantee. By assuming a Lie group structure on the data at hand, we are able to derive a novel regularization of AEs, allowing for the first time to ensure the generalization of AEs in the finite training set case. We validate our theoretical analysis by demonstrating how this regularization significantly increases the generalization of the AE on various datasets.
更多查看译文
关键词
provable finite data generalization,group autoencoder
AI 理解论文
溯源树
样例
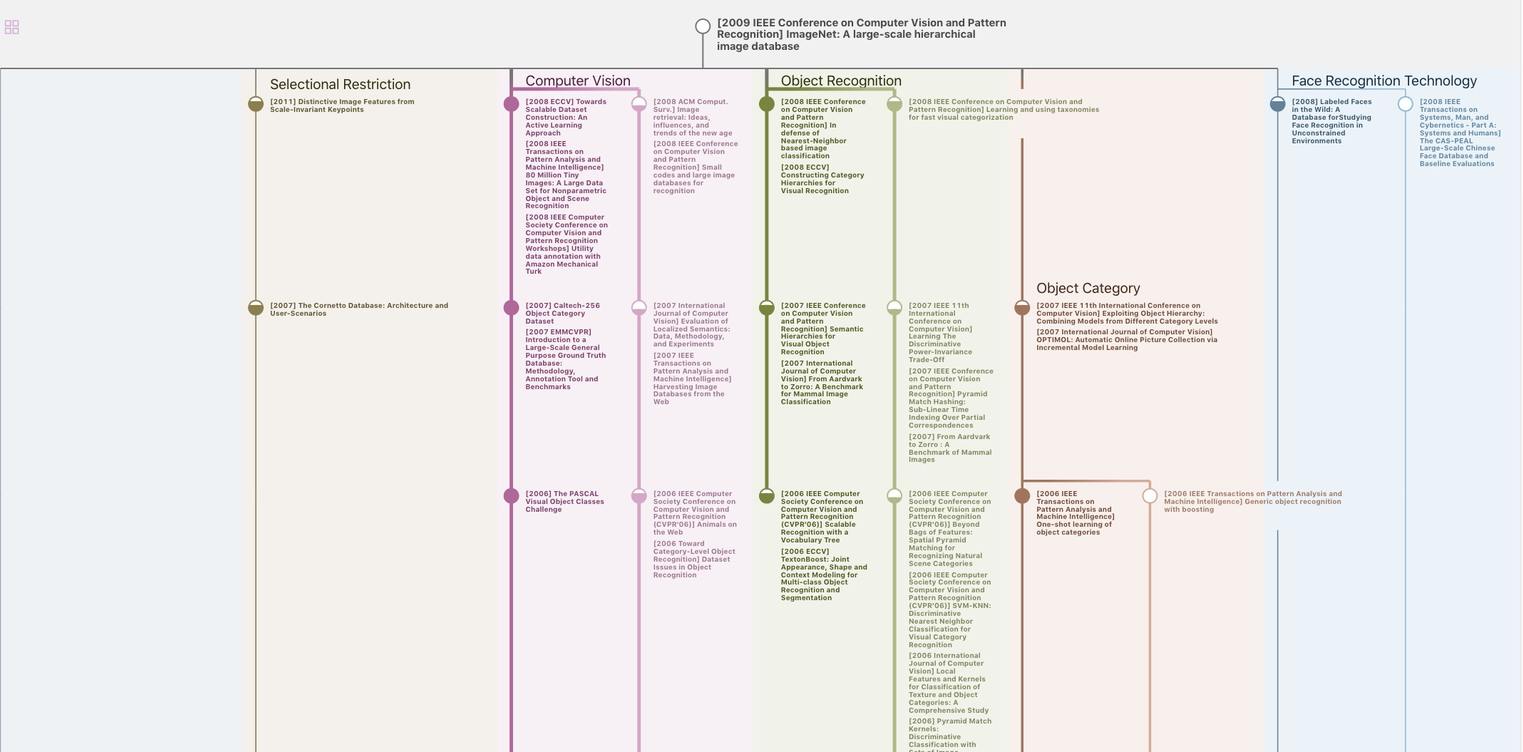
生成溯源树,研究论文发展脉络
Chat Paper
正在生成论文摘要