Biomedical Event Extraction on Graph Edge-conditioned Attention Networks with Hierarchical Knowledge Graphs
EMNLP(2020)
摘要
Biomedical event extraction is critical in understanding biomolecular interactions described in scientific corpus. One of the main challenges is to identify nested structured events that are associated with non-indicative trigger words. We propose to incorporate domain knowledge from Unified Medical Language System (UMLS) to a pre-trained language model via Graph Edge-conditioned Attention Networks (GEANet) and hierarchical graph representation. To better recognize the trigger words, each sentence is first grounded to a sentence graph based on a jointly modeled hierarchical knowledge graph from UMLS. The grounded graphs are then propagated by GEANet, a novel graph neural networks for enhanced capabilities in inferring complex events. On BioNLP 2011 GENIA Event Extraction task, our approach achieved 1.41% F1 and 3.19% F1 improvements on all events and complex events, respectively. Ablation studies confirm the importance of GEANet and hierarchical KG.
更多查看译文
关键词
biomedical event extraction,attention networks,edge-conditioned
AI 理解论文
溯源树
样例
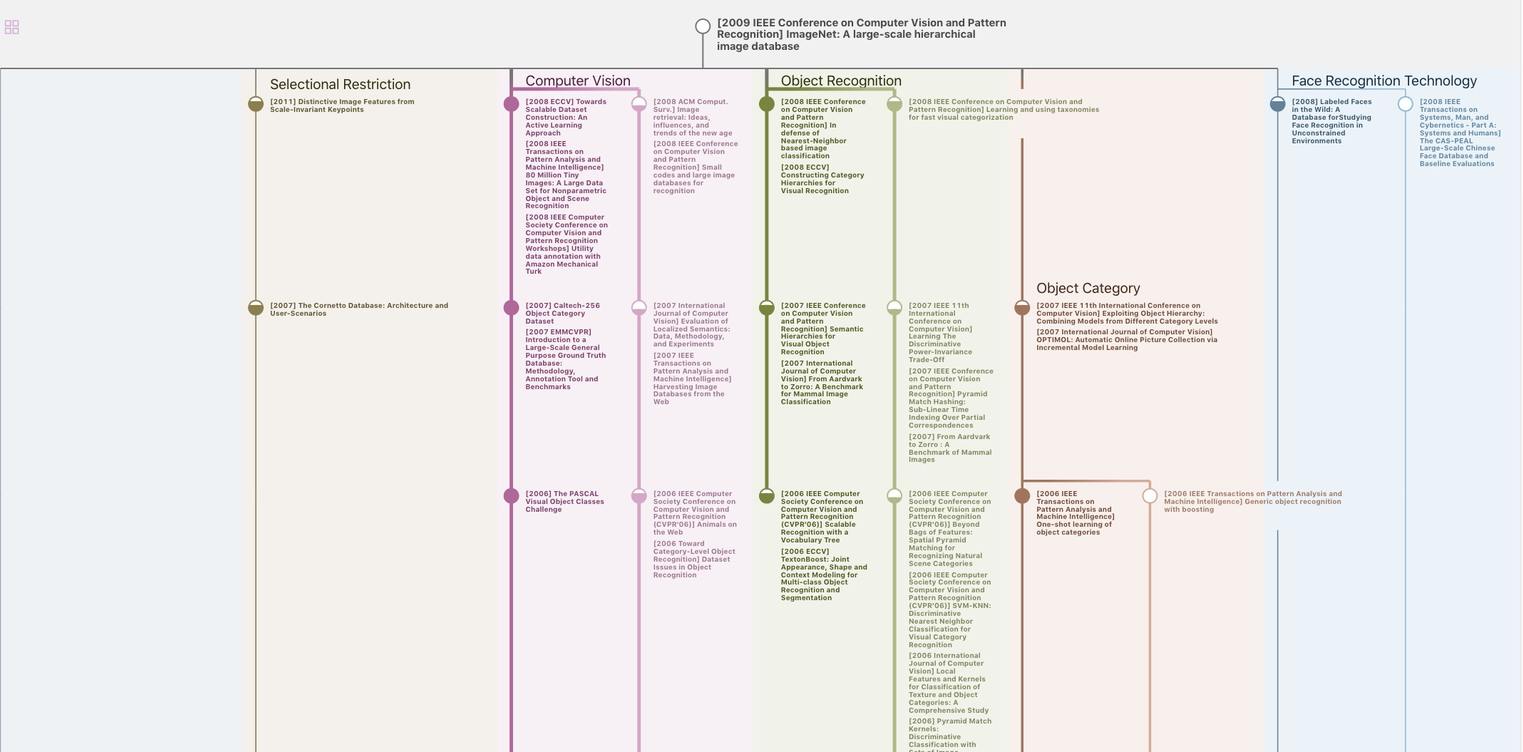
生成溯源树,研究论文发展脉络
Chat Paper
正在生成论文摘要