Oncogenetic Network Estimation with Disjunctive Bayesian Networks: Learning from Unstratified Samples while Preserving Mutual Exclusivity Relations
biorxiv(2020)
Abstract
Cancer is the process of accumulating genetic alterations that confer selective advantages to tumor cells. The order in which aberrations occur is not arbitrary, and inferring the order of events is a challenging problem due to the lack of longitudinal samples from tumors. Moreover, a network model of oncogenesis should capture biological facts such as distinct progression trajectories of cancer subtypes and patterns of mutual exclusivity of alterations in the same pathways. In this paper, we present the Disjunctive Bayesian Network (DBN), a novel cancer progression model. Unlike previous models of oncogenesis, DBN naturally captures mutually exclusive alterations. Besides, DBN is flexible enough to represent progression trajectories of cancer subtypes, therefore allowing one to learn the progression network from unstratified data, i.e., mixed samples from multiple subtypes. We provide a scalable genetic algorithm to learn the structure of DBN from cross-sectional cancer data. To test our model, we simulate synthetic data from known progression networks and show that our algorithm infers the ground truth network with high accuracy. Finally, we apply our model to copy number data for colon cancer and mutation data for bladder cancer and observe that the recovered progression network matches known biological facts.
MoreTranslated text
Key words
Bayesian network,cancer progression,oncogenetic model,tumor phylogenetic
AI Read Science
Must-Reading Tree
Example
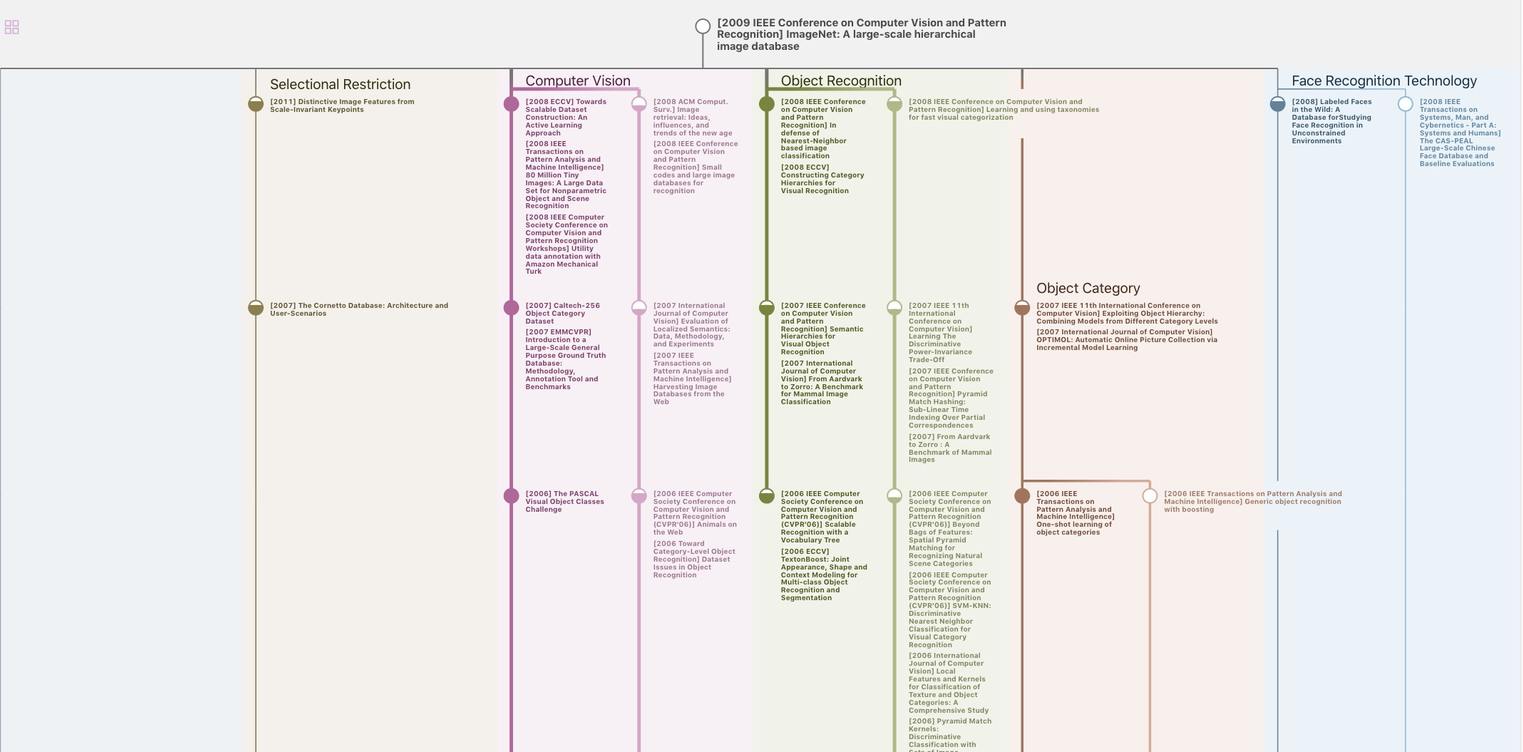
Generate MRT to find the research sequence of this paper
Chat Paper
Summary is being generated by the instructions you defined