Using real-time fluorescence and deformability cytometry and deep learning to transfer molecular specificity to label-free sorting
biorxiv(2019)
摘要
The identification and separation of specific cells from heterogeneous populations is an essential prerequisite for further analysis or use. Conventional passive and active separation approaches rely on fluorescent or magnetic tags introduced to the cells of interest through molecular markers. Such labeling is time- and cost-intensive, can alter cellular properties, and might be incompatible with subsequent use, for example, in transplantation. Alternative label-free approaches utilizing morphological or mechanical features are attractive, but lack molecular specificity. Here we combine image-based real-time fluorescence and deformability cytometry (RT-FDC) with downstream cell sorting using standing surface acoustic waves (SSAW). We demonstrate basic sorting capabilities of the device by separating cell mimics and blood cell types based on fluorescence as well as deformability and other image parameters. The identification of blood sub-populations is enhanced by flow alignment and deformation of cells in the microfluidic channel constriction. In addition, the classification of blood cells using established fluorescence-based markers provides hundreds of thousands of labeled cell images used to train a deep neural network. The trained algorithm, with latency optimized to below 1 ms, is then used to identify and sort unlabeled blood cells at rates of 100 cells/sec. This approach transfers molecular specificity into label-free sorting and opens up new possibilities for basic biological research and clinical therapeutic applications.
更多查看译文
关键词
mechanical phenotype,label-free cell sorting,standing surface acoustic waves (SSAW),artificial intelligence,deep neural networks,flow cytometry,image-based sorting
AI 理解论文
溯源树
样例
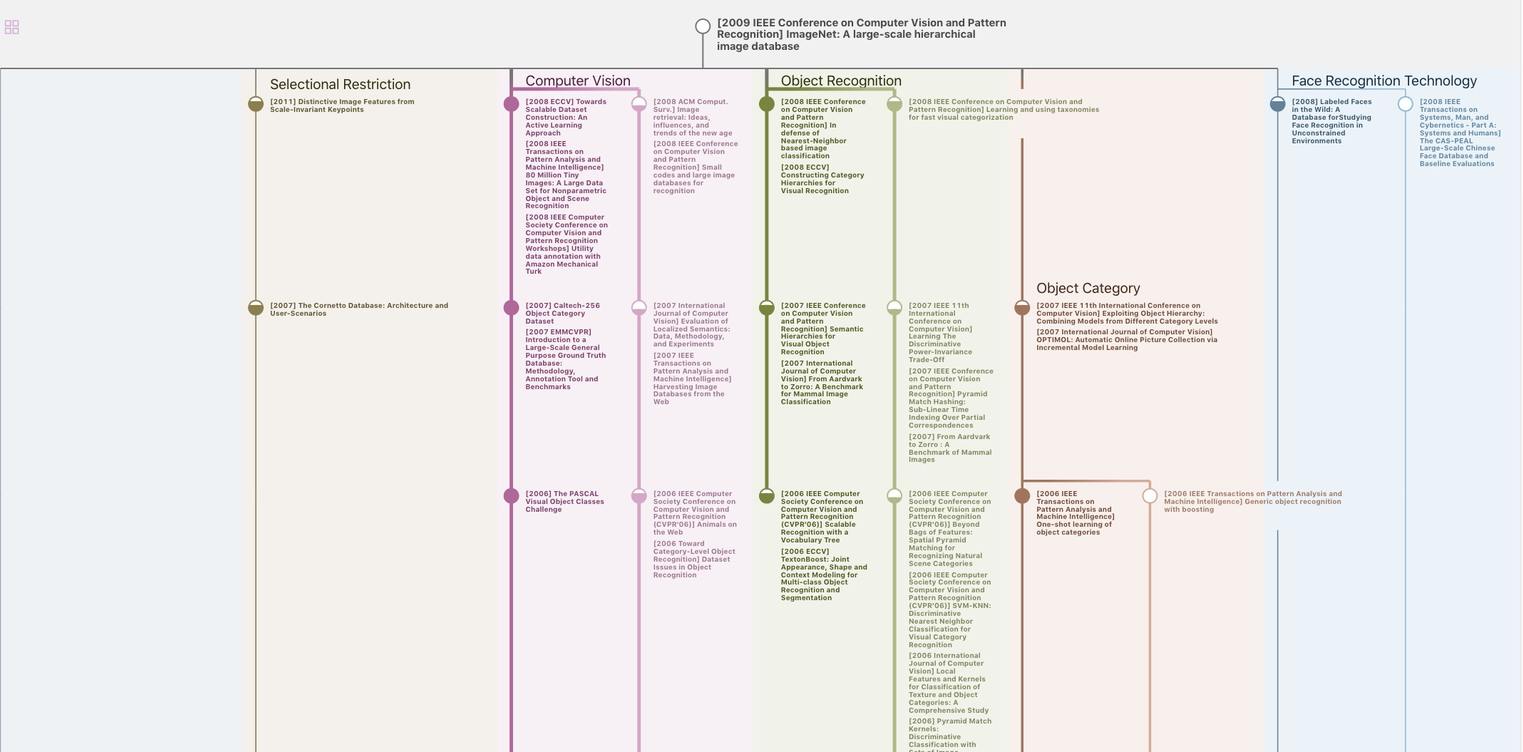
生成溯源树,研究论文发展脉络
Chat Paper
正在生成论文摘要