Learning to Personalize for Web Search Sessions
CIKM '20: The 29th ACM International Conference on Information and Knowledge Management Virtual Event Ireland October, 2020(2020)
摘要
The task of session search focuses on using interaction data to improve relevance for the user's next query at the session level. In this paper, we formulate session search as a personalization task under the framework of learning to rank. Personalization approaches re-rank results to match a user model. Such user models are usually accumulated over time based on the user's browsing behaviour. We use a pre-computed and transparent set of user models based on concepts from the social science literature. Interaction data are used to map each session to these user models. Novel features are then estimated based on such models as well as sessions' interaction data. Extensive experiments on test collections from the TREC session track show statistically significant improvements over current session search algorithms.
更多查看译文
关键词
Session search, User models, Personalization, Retrieval model
AI 理解论文
溯源树
样例
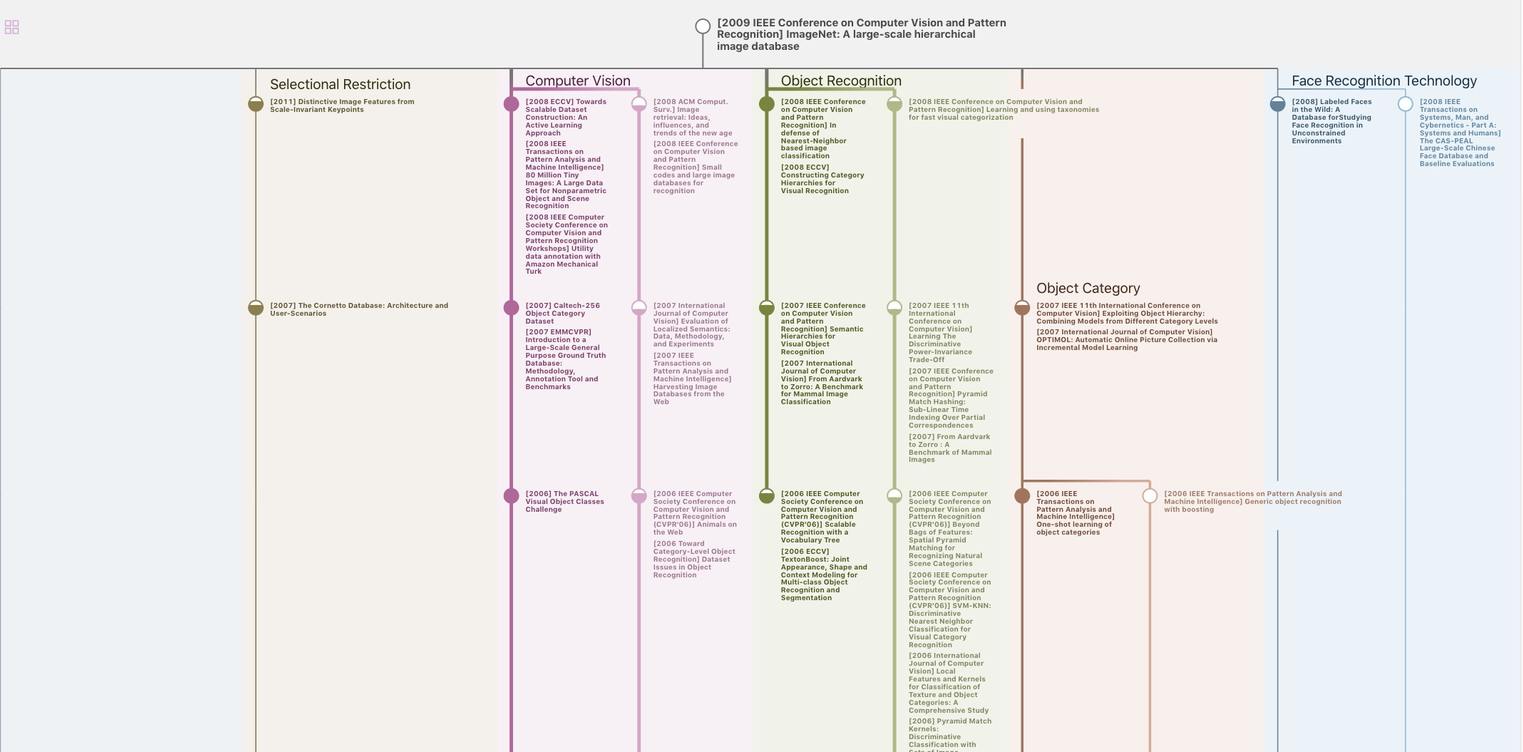
生成溯源树,研究论文发展脉络
Chat Paper
正在生成论文摘要