Statistical inference for high-dimensional vector autoregression with measurement error
STATISTICA SINICA(2023)
摘要
High-dimensional vector autoregressions with measurement errors are frequently encountered in scientific and business applications. We study the statistical inference of the transition matrix under such models. Although numerous works have examined sparse estimations of the transition matrix, relative few provide inference solutions, especially in the high-dimensional setting. We study both global and simultaneous testing of the transition matrix. We first develop a new sparse expectation-maximization algorithm to estimate the model parameters, and carefully characterize the estimation precision. Next, we construct a Gaussian matrix, after proper bias and variance corrections, from which we derive the test statistics. Then, we develop the test procedures and establish their asymptotic guarantees. Finally, we use simulations to investigate performance of our tests, and apply the tests to a neuroimaging-based brain connectivity analysis.
更多查看译文
关键词
Brain network analysis,covariance inference,expectation-maximization,global testing,simultaneous testing,vector autoregression
AI 理解论文
溯源树
样例
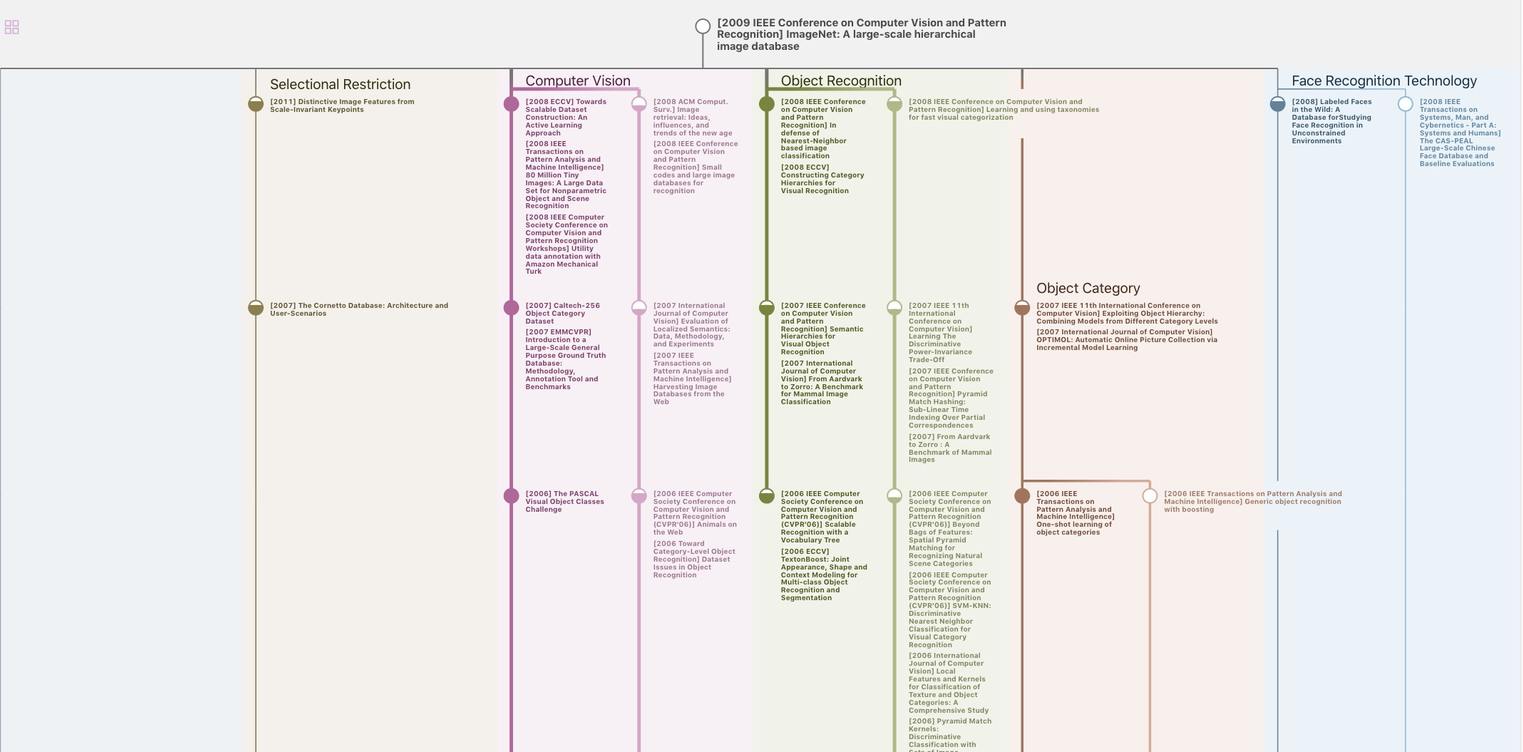
生成溯源树,研究论文发展脉络
Chat Paper
正在生成论文摘要