Convergence acceleration of Monte Carlo many-body perturbation methods by direct sampling
JOURNAL OF CHEMICAL PHYSICS(2020)
摘要
In the Monte Carlo many-body perturbation (MC-MP) method, the conventional correlation-correction formula, which is a long sum of products of low-dimensional integrals, is first recast into a short sum of high-dimensional integrals over electron-pair and imaginary-time coordinates. These high-dimensional integrals are then evaluated by the Monte Carlo method with random coordinates generated by the Metropolis-Hasting algorithm according to a suitable distribution. The latter algorithm, while advantageous in its ability to sample nearly any distribution, introduces autocorrelation in sampled coordinates, which, in turn, increases the statistical uncertainty of the integrals and thus the computational cost. It also involves wasteful rejected moves and an initial "burn-in" step as well as displays hysteresis. Here, an algorithm is proposed that directly produces a random sequence of electron-pair coordinates for the same distribution used in the MC-MP method, which is free from autocorrelation, rejected moves, a burn-in step, or hysteresis. This direct-sampling algorithm is shown to accelerate second- and third-order Monte Carlo many-body perturbation calculations by up to 222% and 38%, respectively.
更多查看译文
关键词
monte carlo,convergence acceleration,many-body
AI 理解论文
溯源树
样例
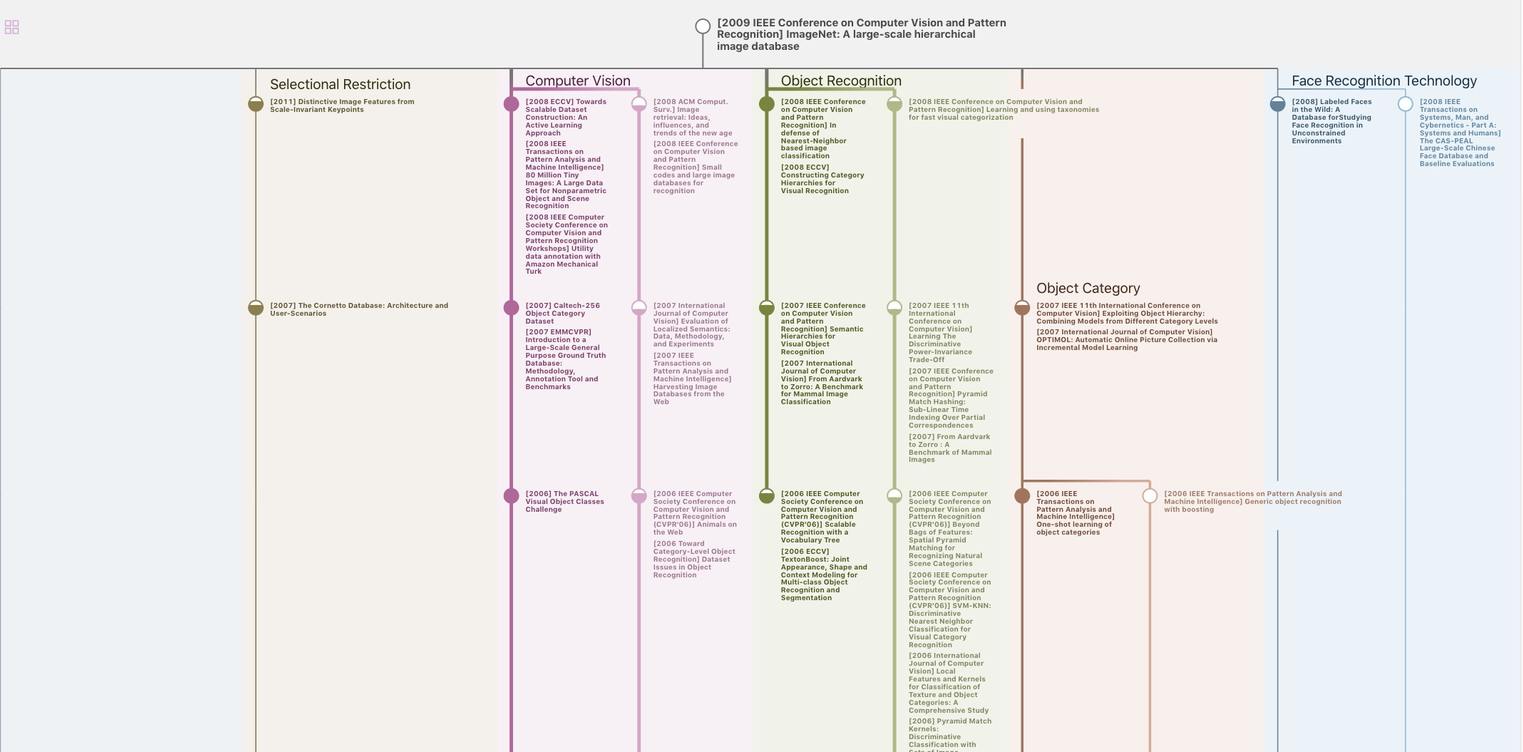
生成溯源树,研究论文发展脉络
Chat Paper
正在生成论文摘要