Revving Up C-13 Nmr Shielding Predictions Across Chemical Space: Benchmarks For Atoms-In-Molecules Kernel Machine Learning With New Data For 134 Kilo Molecules
MACHINE LEARNING-SCIENCE AND TECHNOLOGY(2021)
摘要
The requirement for accelerated and quantitatively accurate screening of nuclear magnetic resonance spectra across the small molecules chemical compound space is two-fold: (1) a robust 'local' machine learning (ML) strategy capturing the effect of the neighborhood on an atom's 'near-sighted' property-chemical shielding; (2) an accurate reference dataset generated with a state-of-the-art first-principles method for training. Herein we report the QM9-NMR dataset comprising isotropic shielding of over 0.8 million C atoms in 134k molecules of the QM9 dataset in gas and five common solvent phases. Using these data for training, we present benchmark results for the prediction transferability of kernel-ridge regression models with popular local descriptors. Our best model, trained on 100k samples, accurately predicts isotropic shielding of 50k 'hold-out' atoms with a mean error of less than 1.9 ppm. For the rapid prediction of new query molecules, the models were trained on geometries from an inexpensive theory. Furthermore, by using a Delta-ML strategy, we quench the error below 1.4 ppm. Finally, we test the transferability on non-trivial benchmark sets that include benchmark molecules comprising 10-17 heavy atoms and drugs.
更多查看译文
关键词
NMR machine learning, kernel ridge regression, drug compounds
AI 理解论文
溯源树
样例
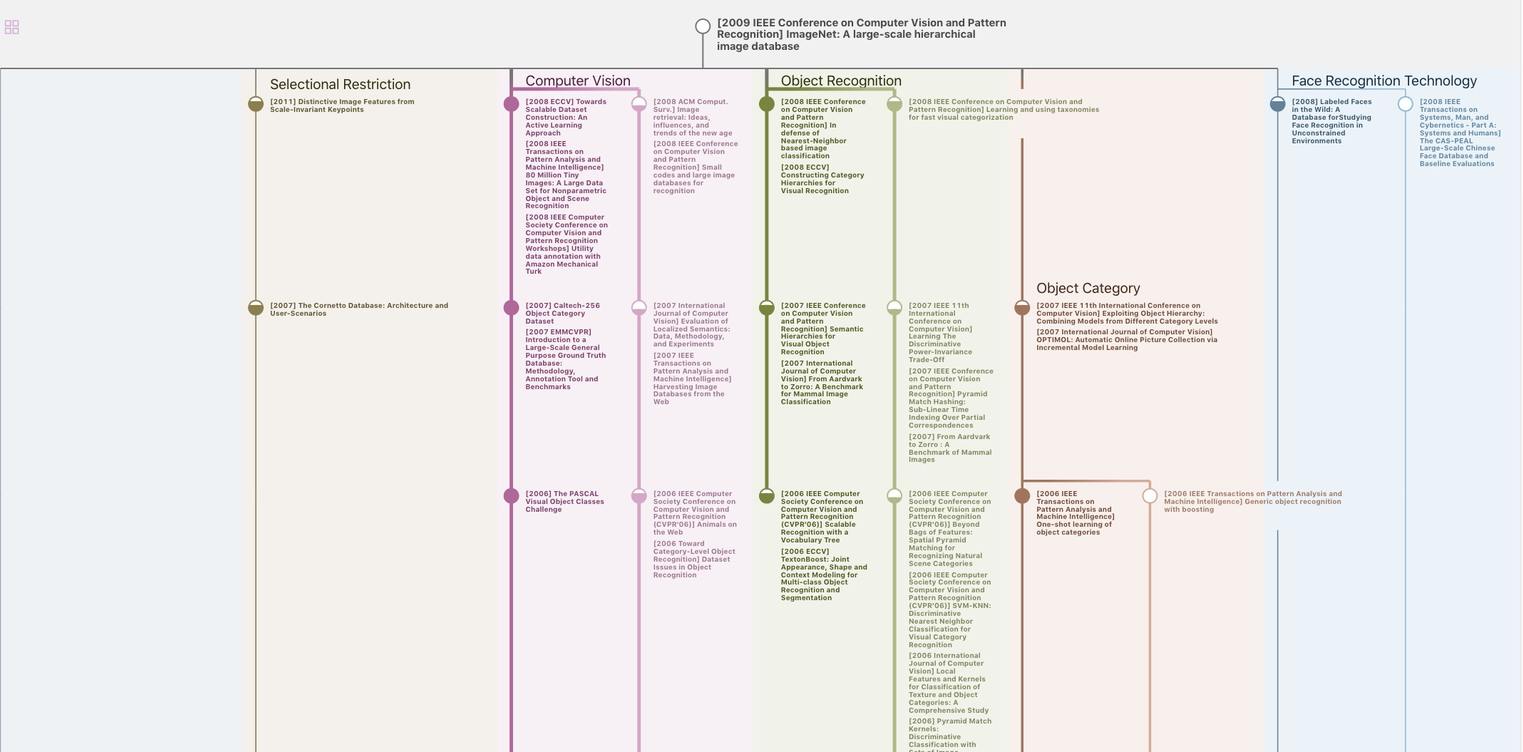
生成溯源树,研究论文发展脉络
Chat Paper
正在生成论文摘要