Oracle-Efficient Reinforcement Learning in Factored MDPs with Unknown Structure
arxiv(2021)
摘要
We consider provably-efficient reinforcement learning (RL) in non-episodic factored Markov decision processes (FMDPs). All previous algorithms for regret minimization in this setting made the strong assumption that the factored structure of the FMDP is known to the learner in advance. In this paper, we provide the first provably-efficient algorithm that has to learn the structure of the FMDP while minimizing its regret. Our algorithm is based on the optimism in face of uncertainty principle, combined with a simple statistical method for structure learning, and can be implemented efficiently given oracle-access to an FMDP planner. It maintains its computational efficiency even though the number of possible structures is exponential.
更多查看译文
AI 理解论文
溯源树
样例
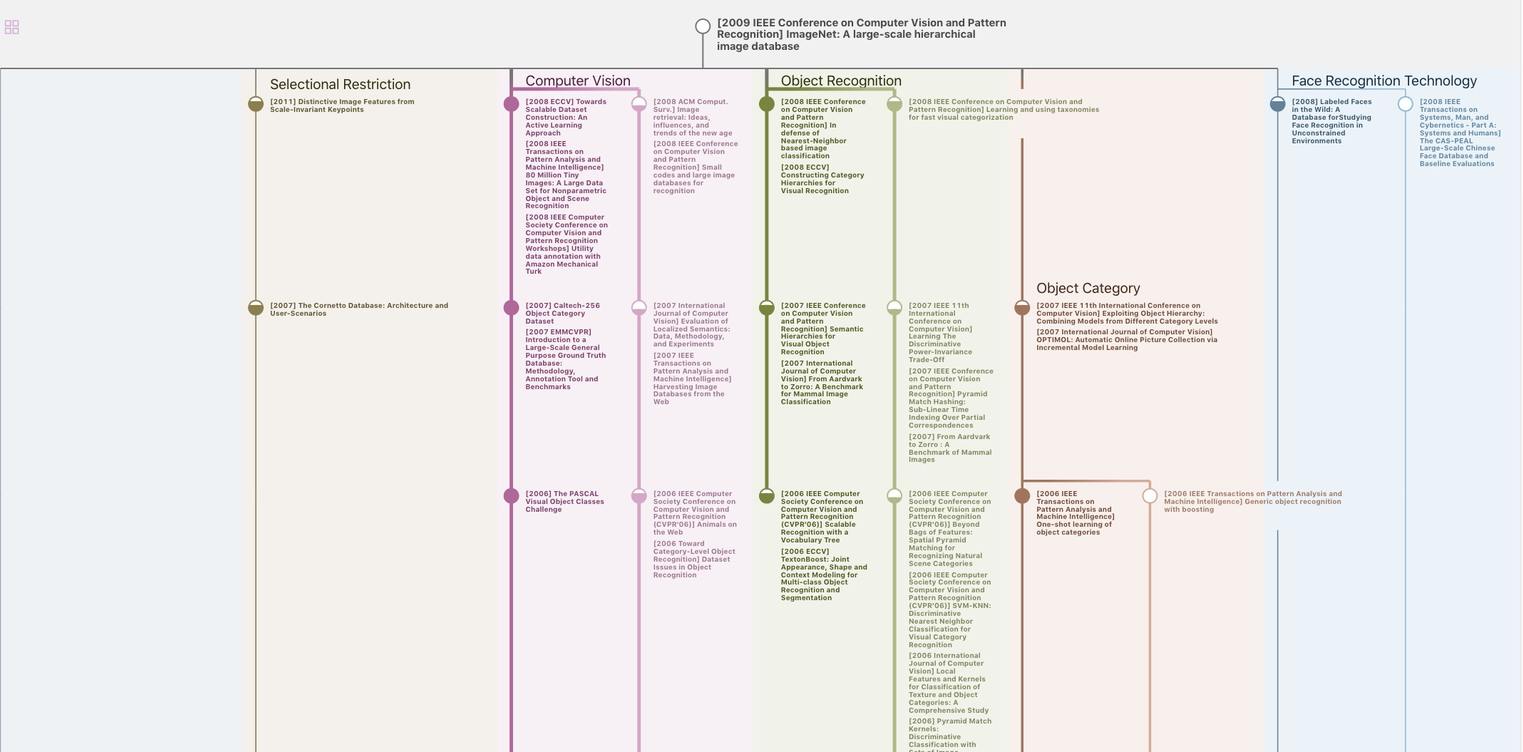
生成溯源树,研究论文发展脉络
Chat Paper
正在生成论文摘要