Deep Analog-to-Digital Converter for Wireless Communication
AI and Optical Data Sciences II(2020)
摘要
With the advent of the 5G wireless networks, achieving tens of gigabits per second throughputs and low, milliseconds, latency has become a reality. This level of performance will fuel numerous real-time applications, such as autonomy and augmented reality, where the computationally heavy tasks can be performed in the cloud. The increase in the bandwidth along with the use of dense constellations places a significant burden on the speed and accuracy of analog-to-digital converters (ADC). A popular approach to create wideband ADCs is utilizing multiple channels each operating at a lower speed in the time-interleaved fashion. However, an interleaved ADC comes with its own set of challenges. The parallel architecture is very sensitive to the inter-channel mismatch, timing jitter, clock skew between different ADC channels as well as the nonlinearity within individual channels. Consequently, complex post-calibration is required using digital signal processing (DSP) after the ADC. The traditional DSP calibration consumes a significant amount of power and its design requires knowledge of the source and type of errors which are becoming increasingly difficult to predict in nanometer CMOS processes. In this paper, instead of individually targeting each source of error, we utilize a deep learning algorithm to learn the complete and complex ADC behavior and to compensate for it in realtime. We demonstrate this "Deep ADC" technique on an 8G Sample/s 8-channel time-interleaved ADC with the QAM-OFDM modulated data. Simulation results for different QAM symbol constellations and OFDM subcarriers show dramatic improvements of approximately 5 bits in the dynamic range with a concomitant drastic reduction in symbol error rate. We further discuss the hardware implementation including latency, power consumption, memory requirements, and chip area.
更多查看译文
关键词
converter,analog-to-digital
AI 理解论文
溯源树
样例
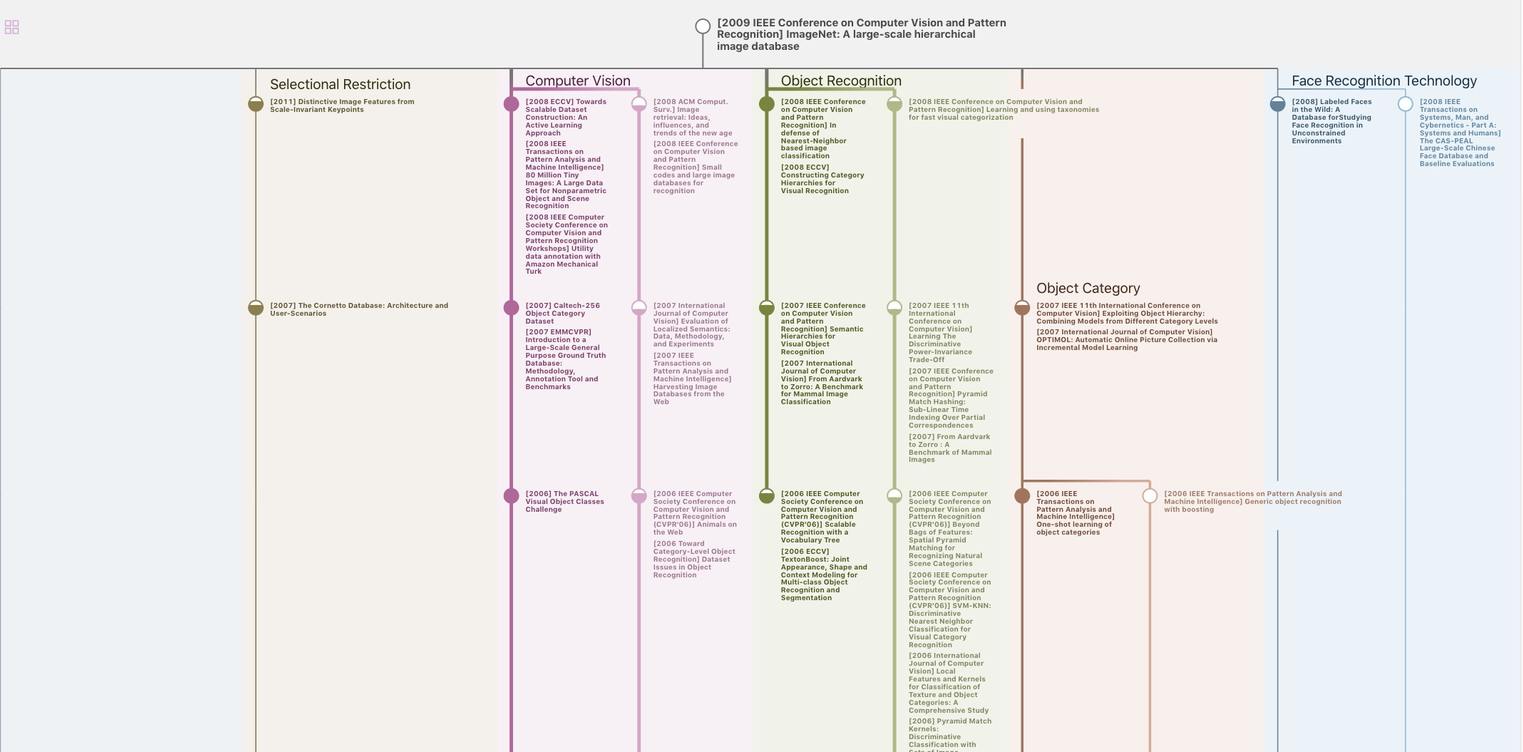
生成溯源树,研究论文发展脉络
Chat Paper
正在生成论文摘要