A family of mixture models for biclustering
STATISTICAL ANALYSIS AND DATA MINING(2022)
摘要
Biclustering is used for simultaneous clustering of the observations and variables when there is no group structure known a priori. It is being increasingly used in bioinformatics, text analytics, and so on. Previously, biclustering has been introduced in a model-based clustering framework by utilizing a structure similar to a mixture of factor analyzers. In such models, observed variables X are modeled using a latent variable U that is assumed to be from N(0, I). Clustering of variables are introduced by imposing constraints on the entries of the factor loading matrix to be 0 and 1 that results in block diagonal covariance matrices. However, this approach is overly restrictive as off-diagonal elements in the blocks of the covariance matrices can only be 1 which can lead to unsatisfactory model fit on complex data. Here, the latent variable U is assumed to be from a N(0, T) where T is a diagonal matrix. This ensures that the off-diagonal terms in the block matrices within the covariance matrices are non-zero and not restricted to be 1. This leads to a superior model fit on complex data. A family of models is developed by imposing constraints on the components of the covariance matrix. For parameter estimation, an alternating expectation conditional maximization (AECM) algorithm is used. Finally, the proposed method is illustrated using simulated and real datasets.
更多查看译文
关键词
AECM, biclustering, factor analysis, mixture models, model-based clustering
AI 理解论文
溯源树
样例
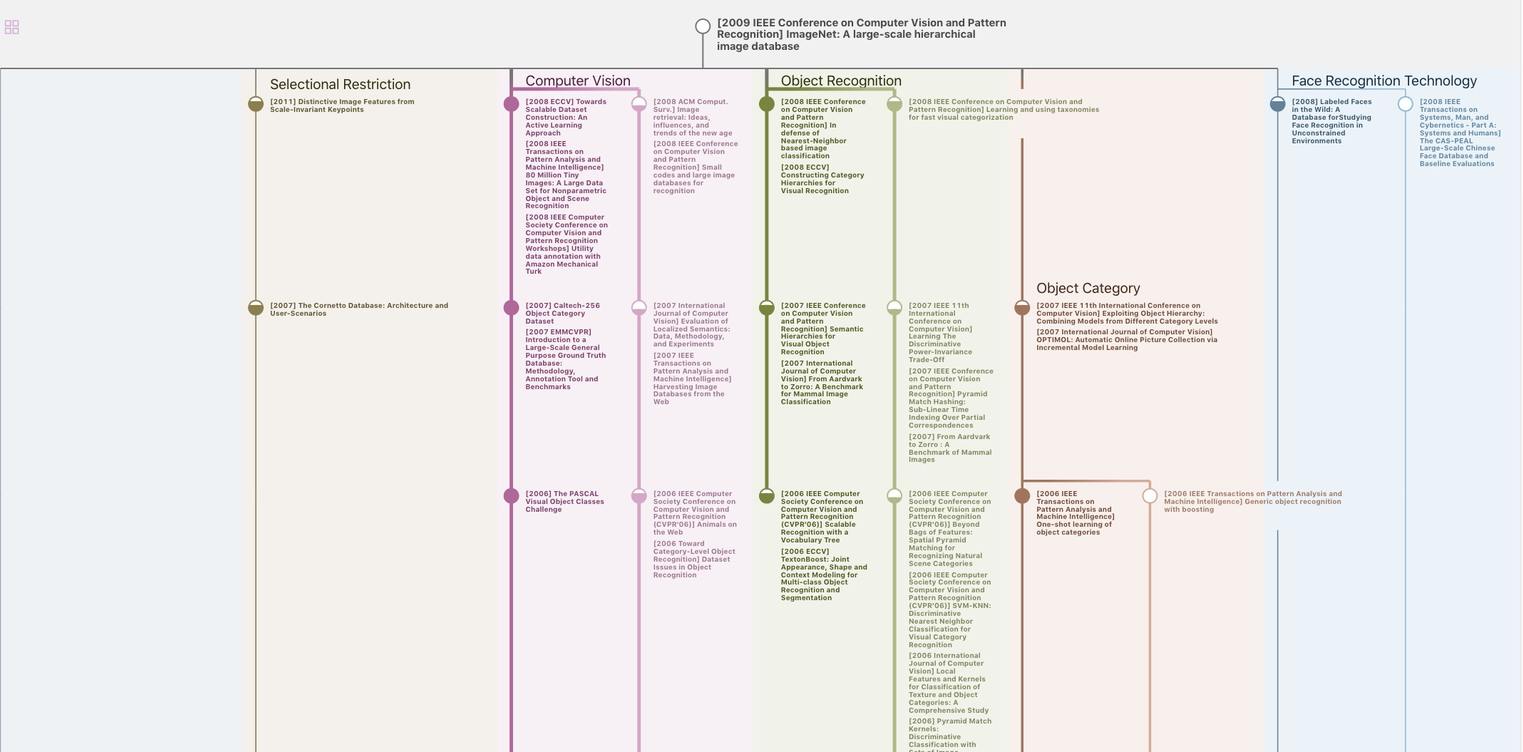
生成溯源树,研究论文发展脉络
Chat Paper
正在生成论文摘要